Development of a novel hybrid multi-boosting neural network model for spatial prediction of urban flood
GEOCARTO INTERNATIONAL(2022)
摘要
In this study, a new hybridized machine learning algorithm for urban flood susceptibility mapping, named MultiB-MLPNN, was developed using a multi-boosting technique and MLPNN. The model was tested in Amol City, Iran, a data-scarce city in an ungauged area which is prone to severe flood inundation events and currently lacks flood prevention infrastructure. Performance of the hybridized model was compared with that of a standalone MLPNN model, random forest and boosted regression trees. Area under the curve, efficiency, true skill statistic, Matthews correlation coefficient, misclassification rate, sensitivity and specificity were used to evaluate model performance. In validation, the MultiB-MLPNN model showed the best predictive performance. The hybridized MultiB-MLPNN model is thus useful for generating realistic flood susceptibility maps for data-scarce urban areas. The maps can be used to develop risk-reduction measures to protect urban areas from devastating floods, particularly where available data are insufficient to support physically based hydrological or hydraulic models.
更多查看译文
关键词
Urban planning, artificial intelligence, neural networks, boosting, GIS
AI 理解论文
溯源树
样例
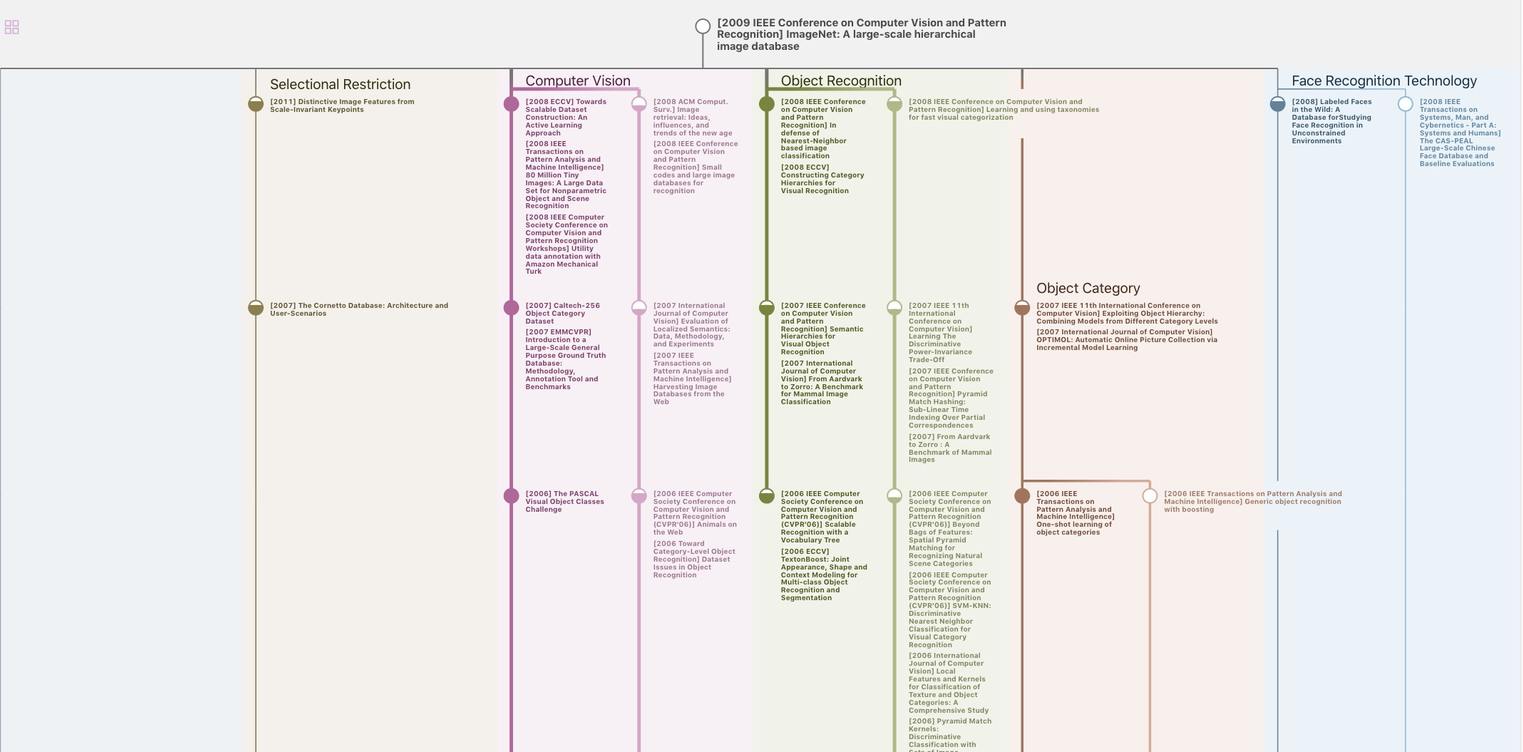
生成溯源树,研究论文发展脉络
Chat Paper
正在生成论文摘要