Estimating lockdown-induced European NO 2 changes using satellite and surface observations and air quality models
Atmospheric Chemistry and Physics(2021)
摘要
Abstract. This study provides a comprehensive assessment of\nNO 2 changes across the main European urban areas induced by COVID-19\nlockdowns using satellite retrievals from the Tropospheric Monitoring\nInstrument (TROPOMI) onboard the Sentinel-5p satellite, surface site\nmeasurements, and simulations from the Copernicus Atmosphere Monitoring\nService (CAMS) regional ensemble of air quality models. Some recent\nTROPOMI-based estimates of changes in atmospheric NO 2 concentrations\nhave neglected the influence of weather variability between the reference\nand lockdown periods. Here we provide weather-normalized estimates based on\na machine learning method (gradient boosting) along with an assessment of\nthe biases that can be expected from methods that omit the influence of\nweather. We also compare the weather-normalized satellite-estimated NO 2 \ncolumn changes with weather-normalized surface NO 2 concentration\nchanges and the CAMS regional ensemble, composed of 11 models, using\nrecently published estimates of emission reductions induced by the lockdown.\nAll estimates show similar NO 2 reductions. Locations where the lockdown\nmeasures were stricter show stronger reductions, and, conversely, locations\nwhere softer measures were implemented show milder reductions in NO 2 \npollution levels. Average reduction estimates based on either satellite\nobservations ( − 23 %), surface stations ( − 43 %), or models ( − 32 %) are\npresented, showing the importance of vertical sampling but also the\nhorizontal representativeness. Surface station estimates are significantly\nchanged when sampled to the TROPOMI overpasses ( − 37 %), pointing out the\nimportance of the variability in time of such estimates. Observation-based\nmachine learning estimates show a stronger temporal variability than\nmodel-based estimates.
更多查看译文
AI 理解论文
溯源树
样例
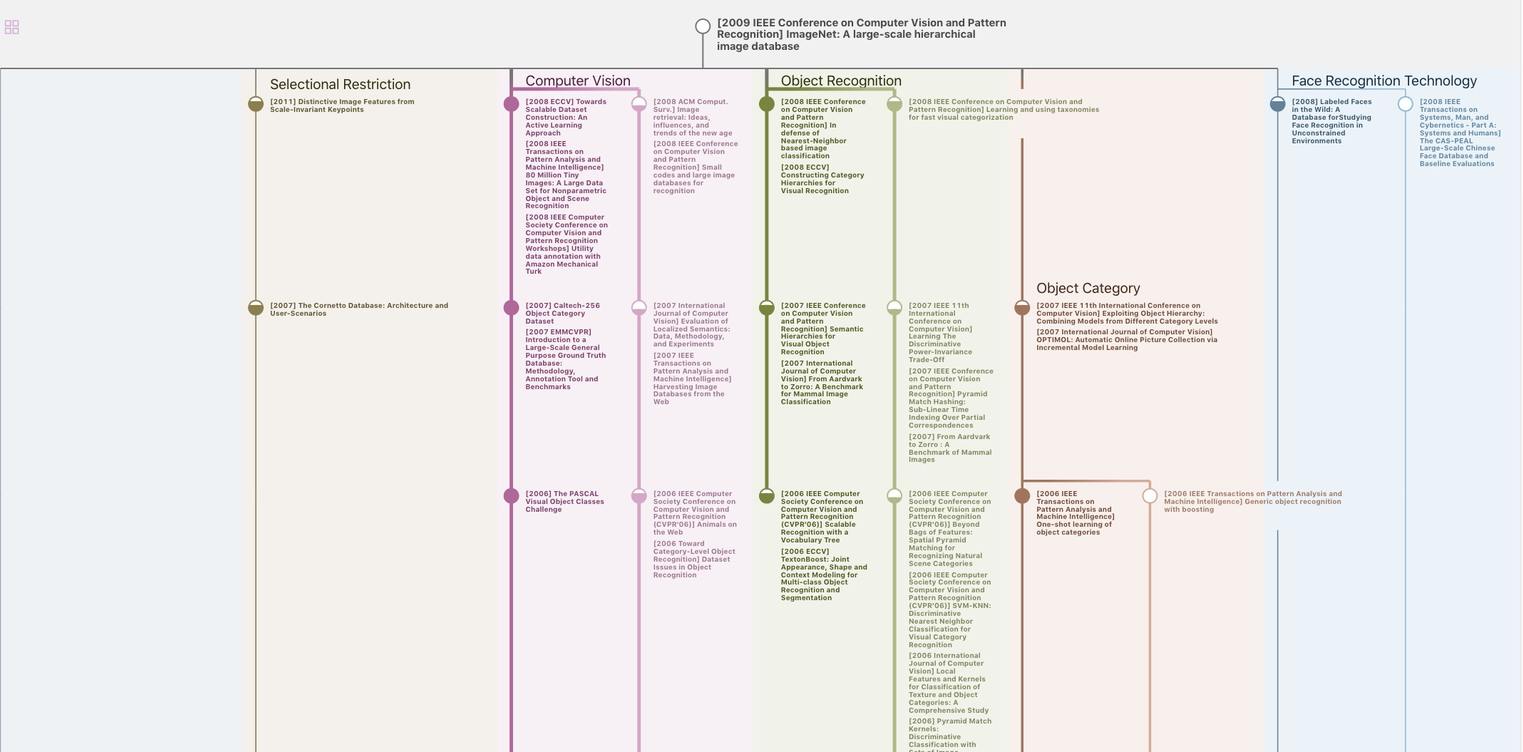
生成溯源树,研究论文发展脉络
Chat Paper
正在生成论文摘要