AdaGUM: An Adaptive Graph Updating Model-Based Anomaly Detection Method for Edge Computing Environment
SECURITY AND COMMUNICATION NETWORKS(2021)
Abstract
With the rapid development of Internet of Things (IoT), massive sensor data are being generated by the sensors deployed everywhere at an unprecedented rate. As the number of Internet of Things devices is estimated to grow to 25 billion by 2021, when facing the explicit or implicit anomalies in the real-time sensor data collected from Internet of Things devices, it is necessary to develop an effective and efficient anomaly detection method for IoT devices. Recent advances in the edge computing have significant impacts on the solution of anomaly detection in IoT. In this study, an adaptive graph updating model is first presented, based on which a novel anomaly detection method for edge computing environment is then proposed. At the cloud center, the unknown patterns are classified by a deep leaning model, based on the classification results, the feature graphs are updated periodically, and the classification results are constantly transmitted to each edge node where a cache is employed to keep the newly emerging anomalies or normal patterns temporarily until the edge node receives a newly updated feature graph. Finally, a series of comparison experiments are conducted to demonstrate the effectiveness of the proposed anomaly detection method for edge computing. And the results show that the proposed method can detect the anomalies in the real-time sensor data efficiently and accurately. More than that, the proposed method performs well when there exist newly emerging patterns, no matter they are anomalous or normal.
MoreTranslated text
Key words
edge computing environment,anomaly detection method,model-based
AI Read Science
Must-Reading Tree
Example
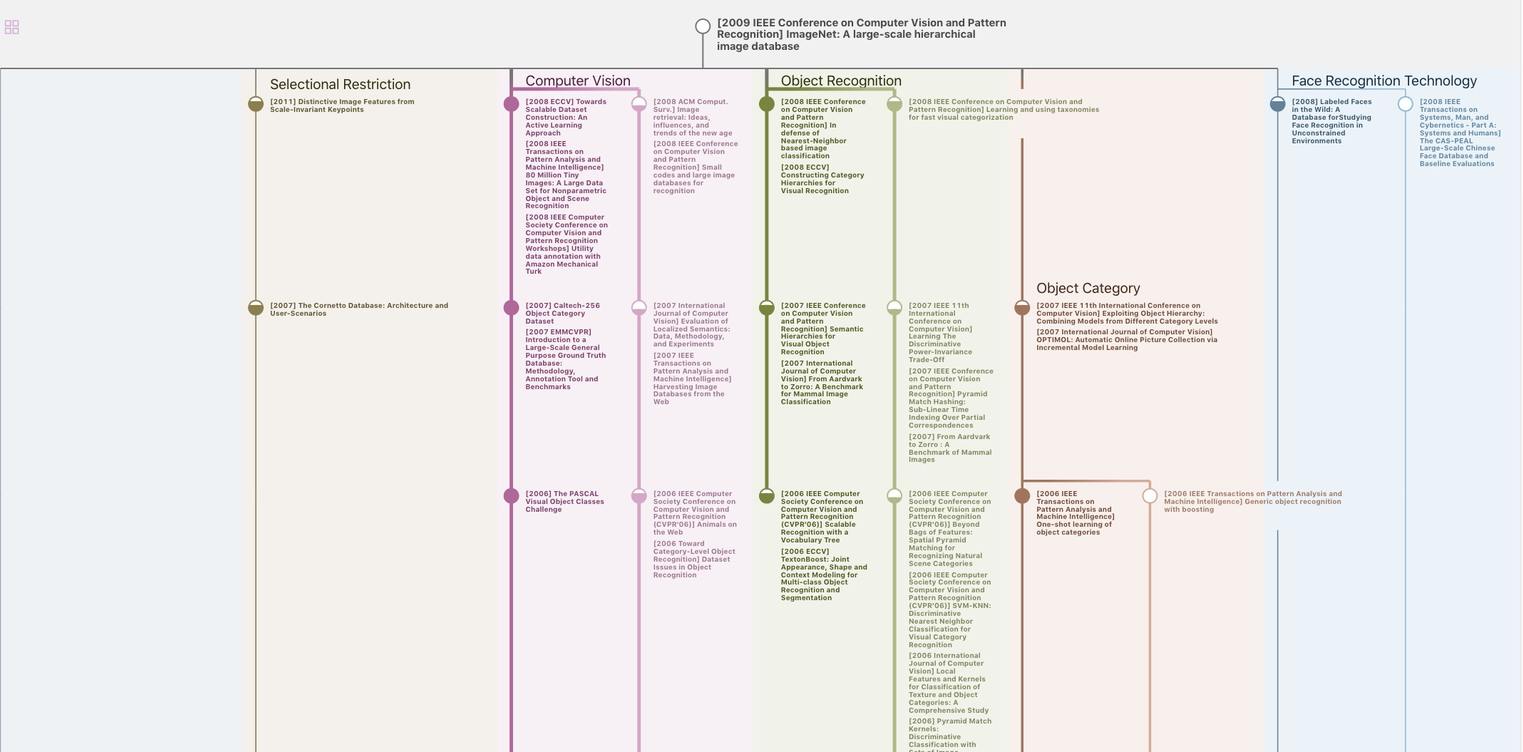
Generate MRT to find the research sequence of this paper
Chat Paper
Summary is being generated by the instructions you defined