Difference Value Network For Image Super-Resolution
IEEE SIGNAL PROCESSING LETTERS(2021)
摘要
Recently, improved performance has been achieved in image super-resolution (SR) by using deep convolutional neural networks (CNNs). However, most existing networks neglect the feature correlations of adjacent layers, causing features at different levels to not be fully utilized. In this paper, a novel difference value network (DVN) is proposed to address this problem. The proposed network makes full use of different levels of features by using the difference values (D-values) of adjacent layers. Specifically, a difference value block (DVB) is designed to extract the difference values of adjacent layers. The extracted difference value can highlight which regions should be paid more attention to, so as to guide image SR. Further, a difference value group (DVG) is designed to integrate the difference values extracted by the difference value block into its output. In this way, the DVG can provide additional structure prior for image SR. Finally, to make our network more stable, a multipath supervised reconstruction block is proposed to supervise the reconstruction process. The experimental results on five benchmark datasets show that the proposed network can achieve better reconstruction results than the compared SR methods.
更多查看译文
关键词
Image reconstruction, Feature extraction, Convolution, Superresolution, Training, Graphics, Correlation, Image super-resolution, deep convolutional neural networks, adjacent layers, difference value
AI 理解论文
溯源树
样例
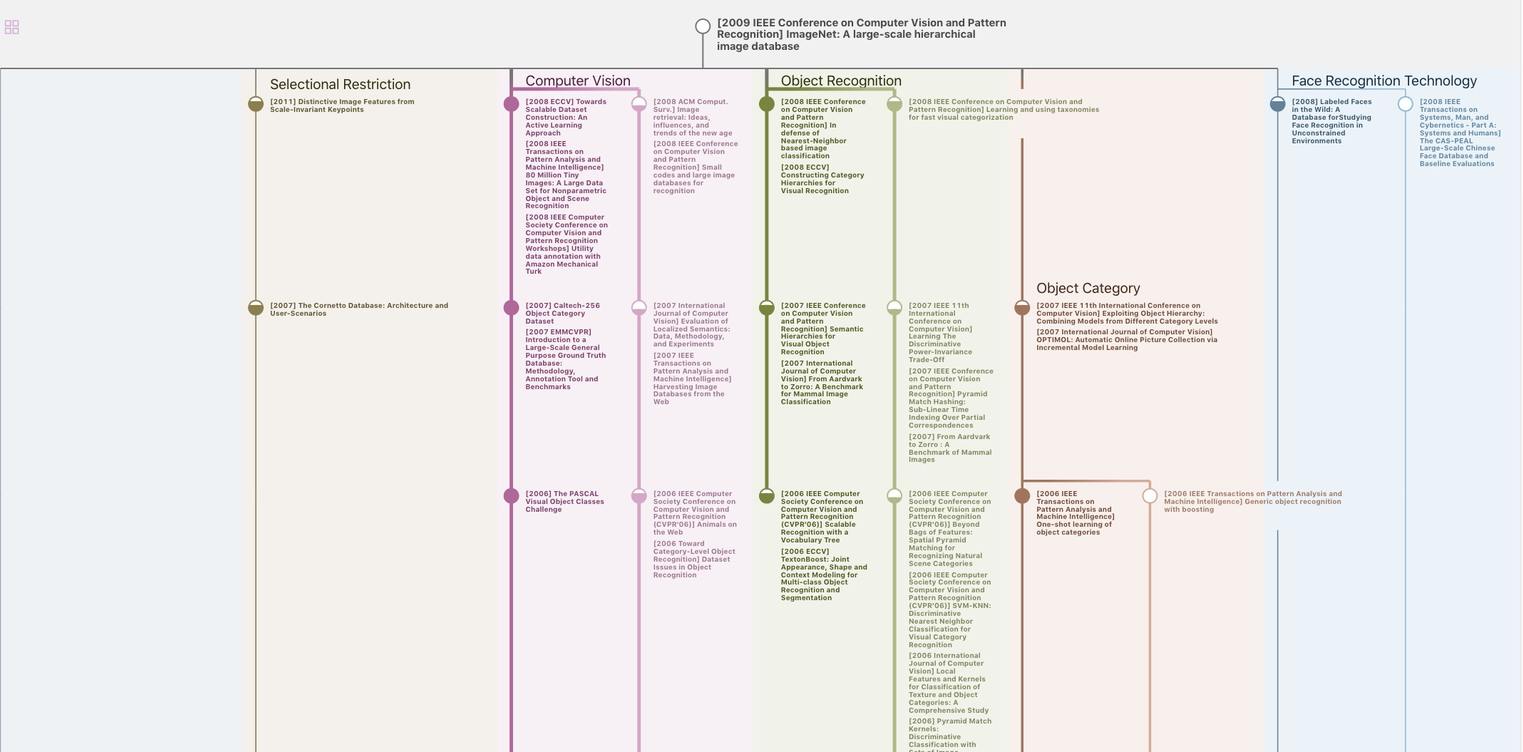
生成溯源树,研究论文发展脉络
Chat Paper
正在生成论文摘要