Border Trespasser Classification Using Artificial Intelligence
IEEE ACCESS(2021)
Abstract
Monitoring the border is a very important task for national security. Wireless sensor networks (WSN) appear well suited in this application. This work aims to monitor a large-scale geographical framework that represents the borders of countries. Researchers take the Tunisian Algerian border as an example. This border is labeled by the illegal passage of intruders between the two countries. The task is to identify the intruders and study their kinematics based on speed, acceleration, and bearing. The appropriate types of sensors are determined according to the nature of intruders. Six classification techniques are compared which are: Naive Bayes, Support Vector Machine (SVM), Multilayer Perceptron, Best First Decision Tree (BF-Tree), Logistic Alternating Decision Tree (LAD-Tree), and J48. The comparison of the performance of the classification techniques is provided in terms of correct differentiation rates, confusion matrices, and the time taken to build each model. Four different levels of cross-validation are used to validate the classifiers. The results indicate that J48 has achieved the highest correct classification rate with a relatively low model-building time.
MoreTranslated text
Key words
Sensors, Wireless sensor networks, Sensor phenomena and characterization, Magnetic sensors, Kinematics, Surveillance, Support vector machines, Border surveillance, classification, machine learning, sensing, wireless sensor networks
AI Read Science
Must-Reading Tree
Example
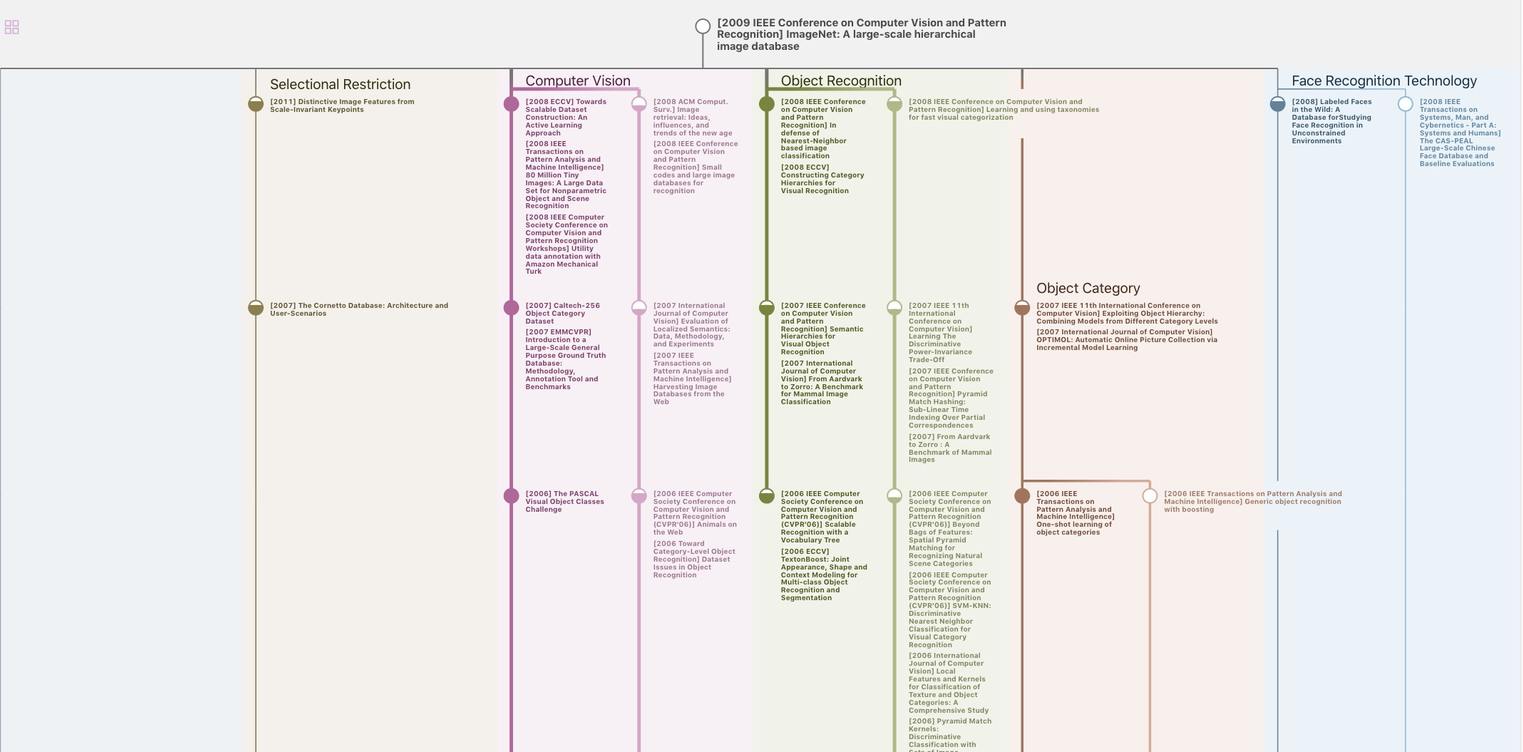
Generate MRT to find the research sequence of this paper
Chat Paper
Summary is being generated by the instructions you defined