A Deep Learning Technique-Based Automatic Monitoring Method For Experimental Urban Road Inundation
JOURNAL OF HYDROINFORMATICS(2021)
摘要
Reports indicate that high-cost, insecurity, and difficulty in complex environments hinder the traditional urban road inundation monitoring approach. This work proposed an automatic monitoring method for experimental urban road inundation based on the YOLOv2 deep learning framework. The proposed method is an affordable, secure, with high accuracy rates in urban road inundation evaluation. The automatic detection of experimental urban road inundation was carried out under both dry and wet conditions on roads in the study area with a scale of a few m(2). The validation average accuracy rate of the model was high with 90.1% inundation detection, while its training average accuracy rate was 96.1%. This indicated that the model has effective performance with high detection accuracy and recognition ability. Besides, the inundated water area of the experimental inundation region and the real road inundation region in the images was computed, showing that the relative errors of the measured area and the computed area were less than 20%. The results indicated that the proposed method can provide reliable inundation area evaluation. Therefore, our findings provide an effective guide in the management of urban floods and urban flood-warning, as well as systematic validation data for hydrologic and hydrodynamic models.
更多查看译文
关键词
deep learning technique, experimental urban road inundation, inundation area, YOLOv2 model
AI 理解论文
溯源树
样例
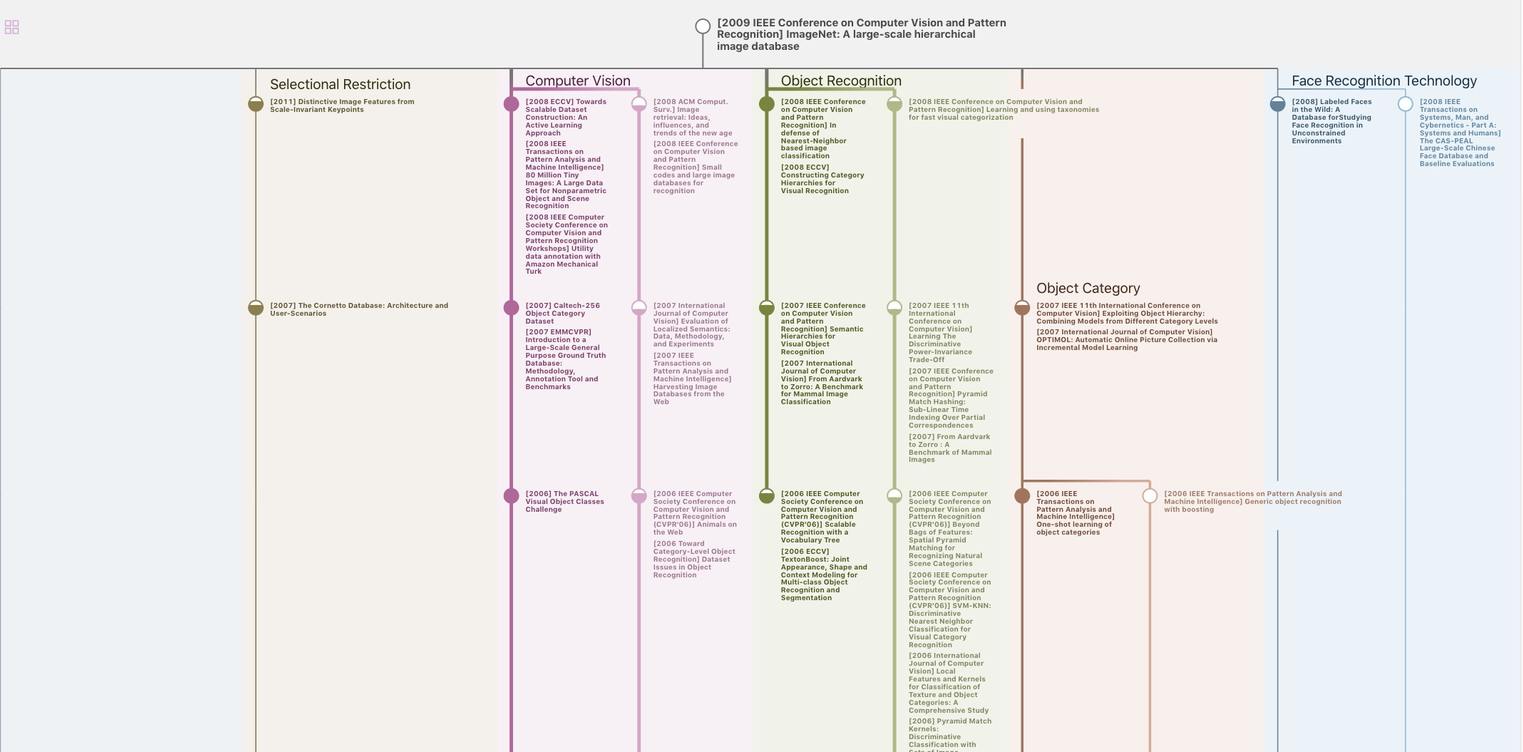
生成溯源树,研究论文发展脉络
Chat Paper
正在生成论文摘要