Hierarchical Pose Classification for Infant Action Analysis and Mental Development Assessment
2021 IEEE INTERNATIONAL CONFERENCE ON ACOUSTICS, SPEECH AND SIGNAL PROCESSING (ICASSP 2021)(2021)
摘要
Based on Alberta Infant Motor Scale (AIMS), a questionnaire that tracks an infant’s motor function, an infant’s mental development can be evaluated by recording poses a baby can achieve. Therefore, it is meaningful to propose a systematic image-based pose classifier to classify infant actions based on AIMS to provide early diagnosis of a potential develop-mental disorder such as Autism. This paper presents a hierarchical pose classifier, given a baby image frame that com-bines the benefits of 3D human pose estimation and scene context information. Due to privacy policies, we cannot collect enough real infant images/videos for experiments. In-stead, we generate synthetic baby images with the help of the Skinned Multi-Infant Linear (SMIL) model. Images are first fed into a ResNet-50 for coarse-level pose classification. A stacked hourglass CNN and a hierarchical 3D pose estimation scheme are used for 2D/3D pose estimation. Finally, an innovative Hierarchical Infant Pose Classifier (HIPC) takes the estimated 3D keypoints and coarse-level pose classification confidence scores to give the fine-level baby pose classification results. Our experimental results show that our hierarchical pose classifier achieves accurate and stable performance on infant pose recognition.
更多查看译文
关键词
Human Pose Estimation, Deep Learning, Hierarchical Classification, ResNet, HIPC
AI 理解论文
溯源树
样例
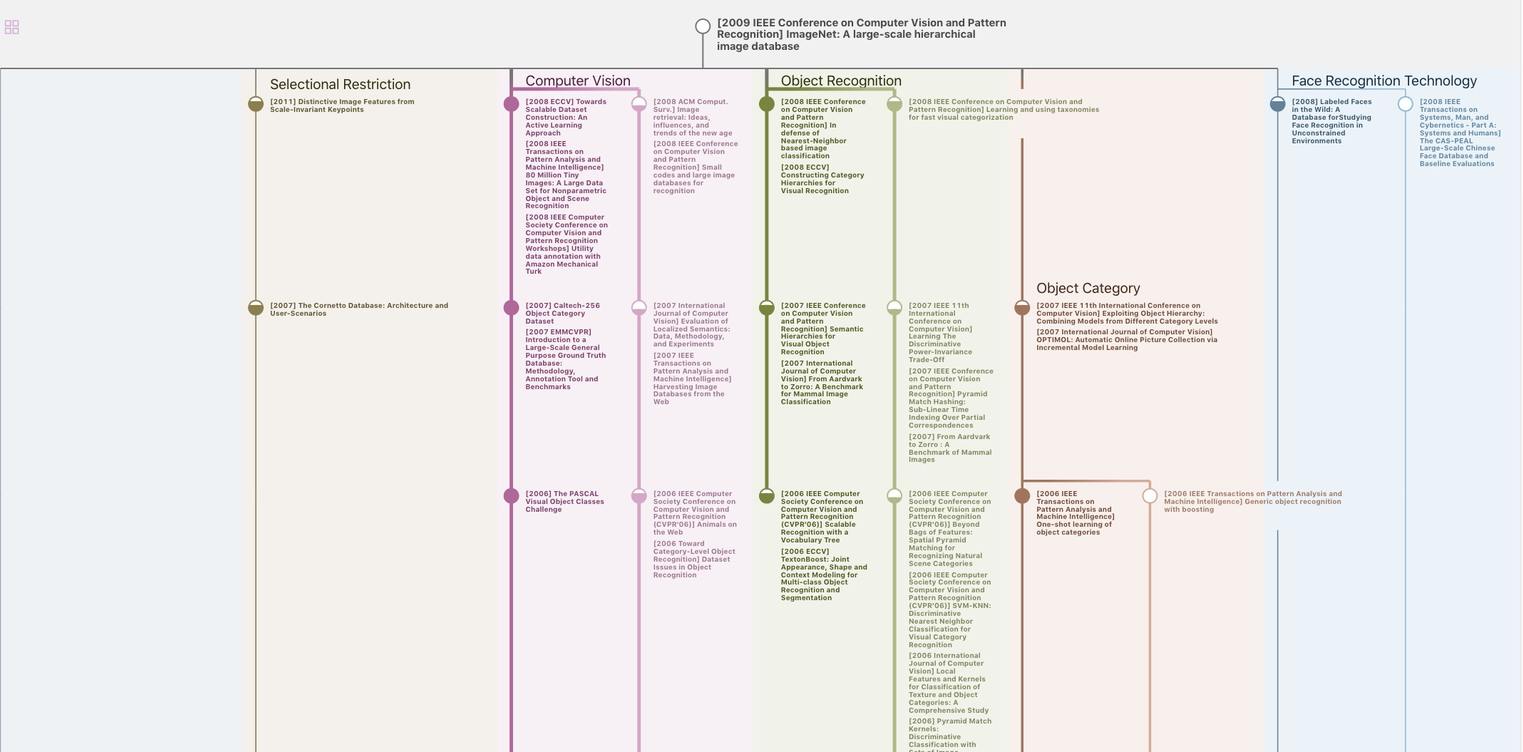
生成溯源树,研究论文发展脉络
Chat Paper
正在生成论文摘要