ICA WITH ORTHOGONALITY CONSTRAINT: IDENTIFIABILITY AND A NEW EFFICIENT ALGORITHM
2021 IEEE INTERNATIONAL CONFERENCE ON ACOUSTICS, SPEECH AND SIGNAL PROCESSING (ICASSP 2021)(2021)
摘要
Given the prevalence of independent component analysis (ICA) for signal processing, many methods for improving the convergence properties of ICA have been introduced. The most utilized methods operate by iterative rotations over pre-whitened data, whereby limiting the space of estimated demixing matrices to those that are orthogonal. However, a proof of the identifiability conditions for orthogonal ICA methods has not yet been presented in the literature. In this paper, we derive the identifiability conditions, starting from the orthogonal ICA maximum likelihood cost function. We then review efficient optimization approaches for orthogonal ICA defined on the Lie group of orthogonal matrices. Afterwards, we derive a new efficient algorithm for orthogonal ICA, by defining a mapping onto a space of constrained matrices which we define as hyper skew-symmetric. Finally, we experimentally demonstrate the advantages of the new algorithm over the pre-existing Lie group methods.
更多查看译文
关键词
Independent Component Analysis, Constrained Optimization, Lie Group Methods, Identifiability
AI 理解论文
溯源树
样例
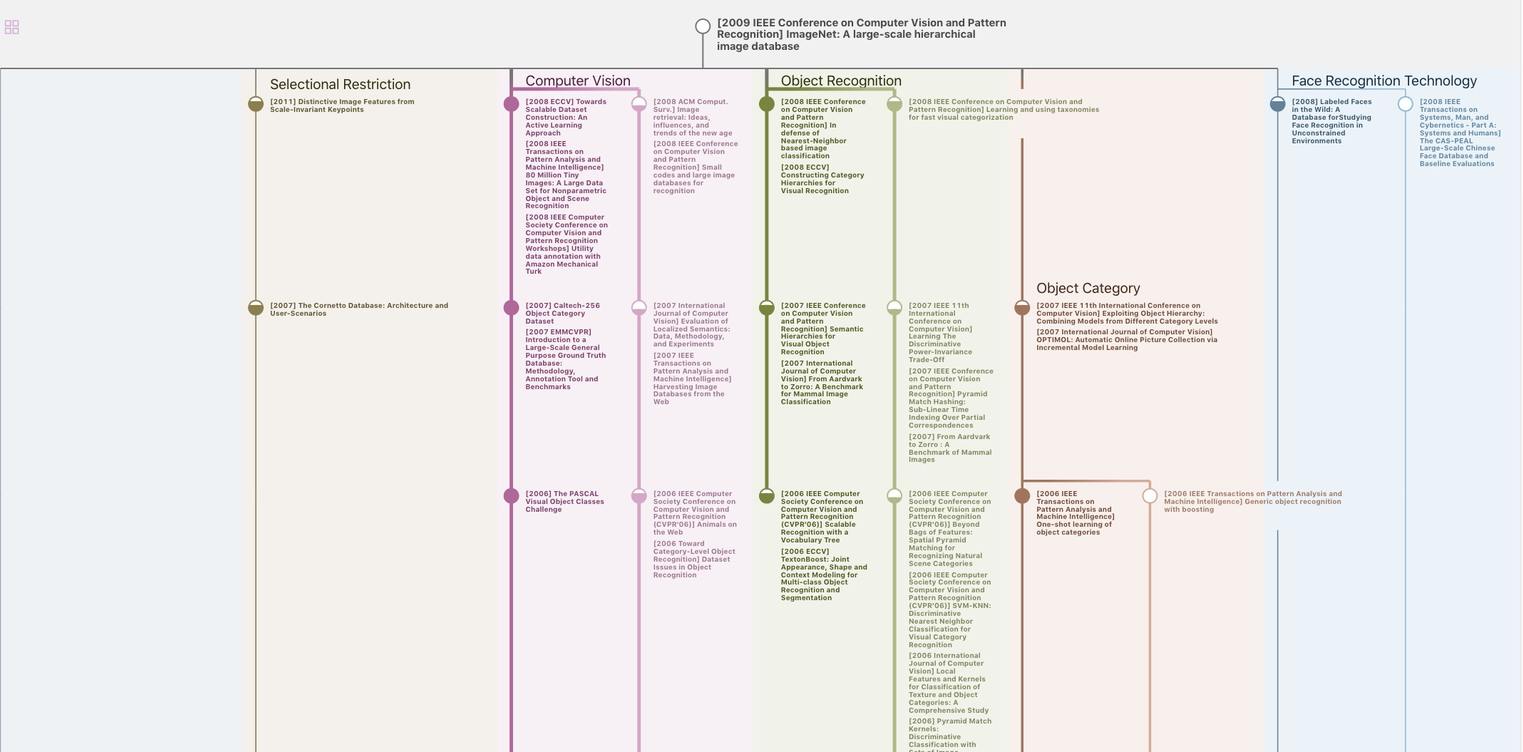
生成溯源树,研究论文发展脉络
Chat Paper
正在生成论文摘要