De-aliased Seismic Data Interpolation Using Conditional Wasserstein Generative Adversarial Networks
Computers & Geosciences(2021)
Key words
De-aliased seismic data interpolation,Conditional generative adversarial network,Wasserstein distance
AI Read Science
Must-Reading Tree
Example
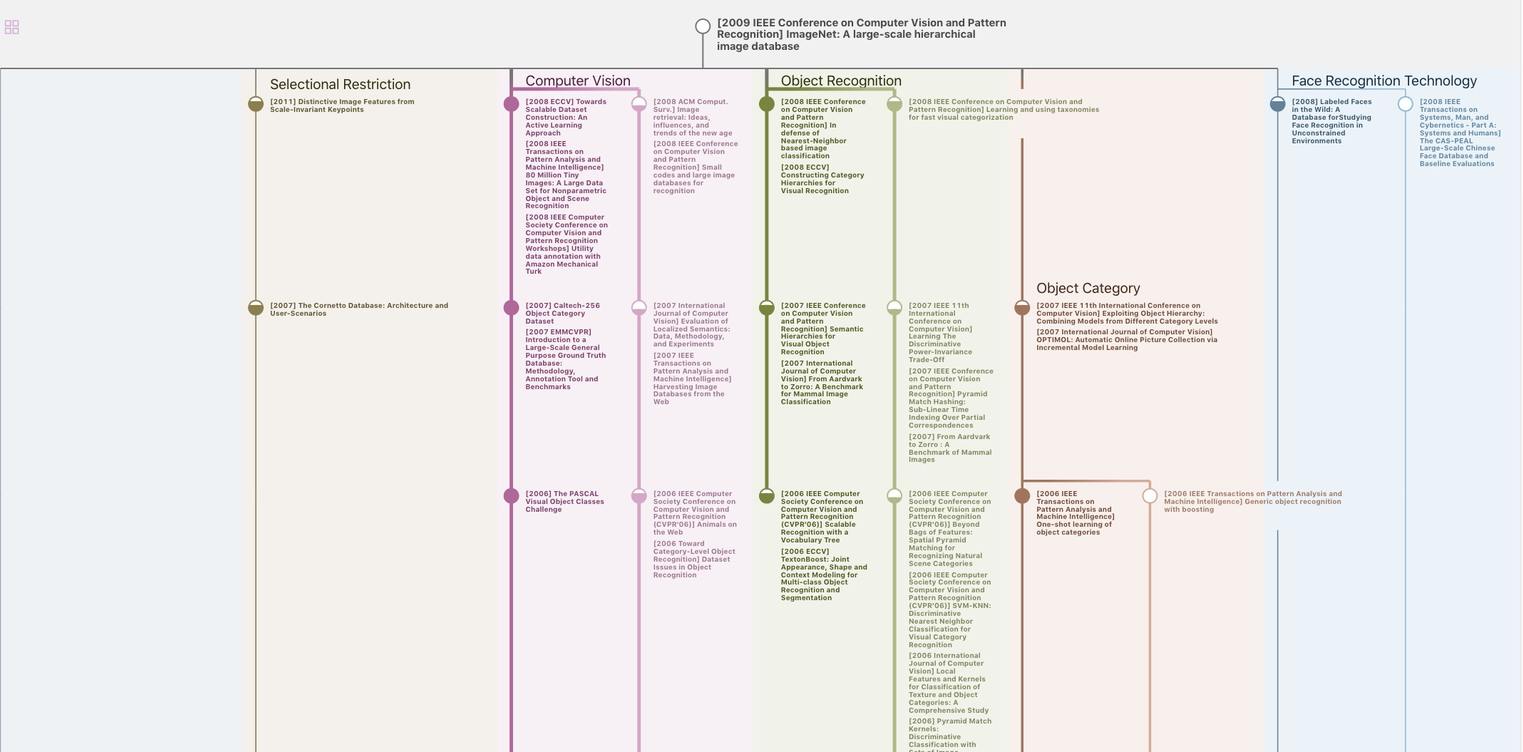
Generate MRT to find the research sequence of this paper
Chat Paper
Summary is being generated by the instructions you defined