A Neural Network-Based Hybrid Framework for Least-Squares Inversion of Transient Electromagnetic Data
IEEE TRANSACTIONS ON GEOSCIENCE AND REMOTE SENSING(2022)
摘要
Inversion of large-scale time-domain transient electromagnetic (TEM) surveys is computationally expensive and time-consuming. The calculation of partial derivatives for the Jacobian matrix is by far the most computationally intensive task, as this requires calculation of a significant number of forward responses. We propose to accelerate the inversion process by predicting partial derivatives using an artificial neural network. Network training data for resistivity models for a broad range of geological settings are generated by computing partial derivatives as symmetric differences between two forward responses. Given that certain applications have larger tolerances for modeling inaccuracy and varying degrees of flexibility throughout the different phases of interpretation, we present four inversion schemes that provide a tunable balance between computational time and inversion accuracy when modeling TEM datasets. We improve speed and maintain accuracy with a hybrid framework, where the neural network derivatives are used initially and switched to full numerical derivatives in the final iterations. We also present a full neural network solution where neural network forward and derivatives are used throughout the inversion. In a least-squares inversion framework, a speedup factor exceeding 70 is obtained on the calculation of derivatives, and the inversion process is expedited similar to 36 times when the full neural network solution is used. Field examples show that the full nonlinear inversion and the hybrid approach gives identical results, whereas the full neural network inversion results in higher deviation but provides a reasonable indication about the overall subsurface geology.
更多查看译文
关键词
Forward modeling, inverse modeling, Jacobian matrix, neural networks, transient electromagnetics (TEM)
AI 理解论文
溯源树
样例
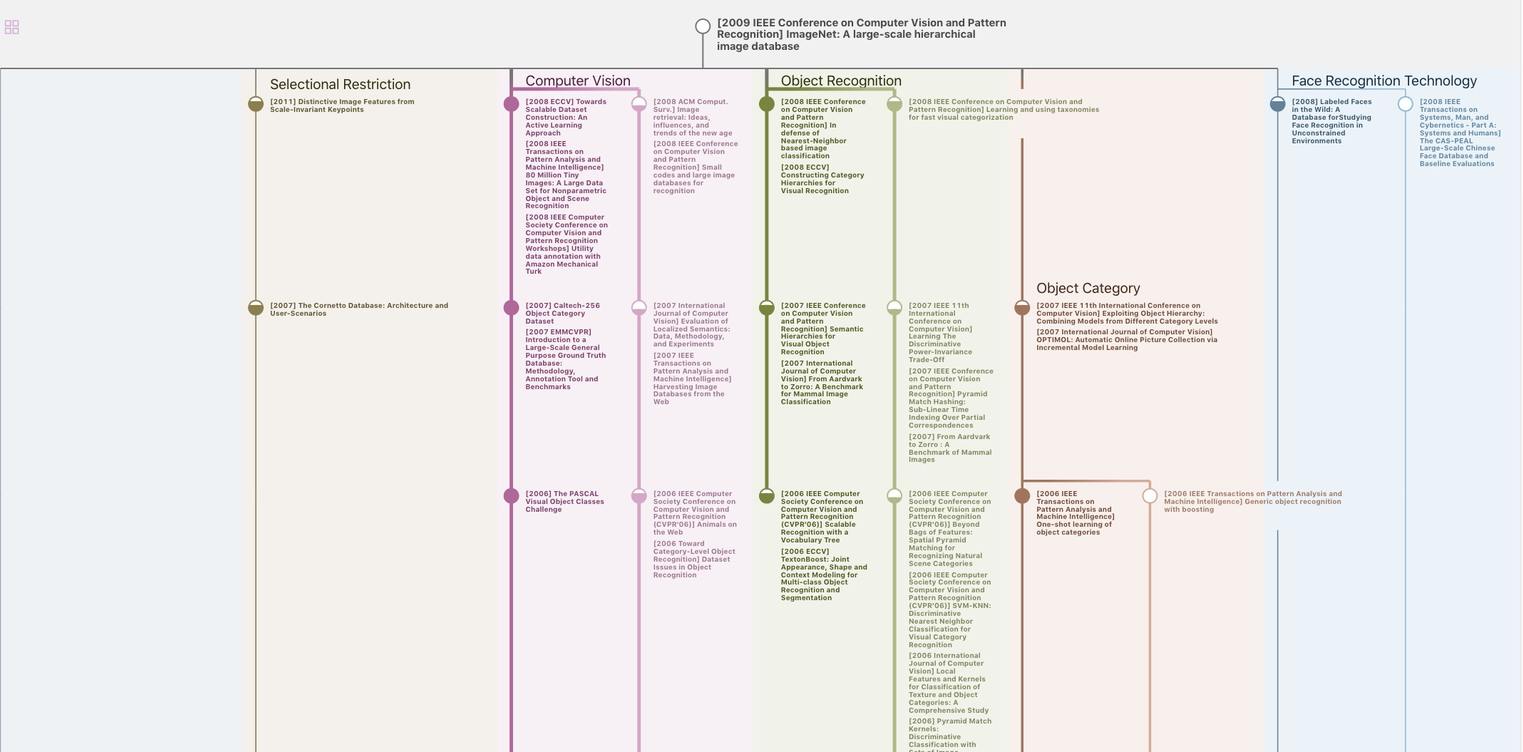
生成溯源树,研究论文发展脉络
Chat Paper
正在生成论文摘要