Meta-adapter: efficient cross-lingual adaptation with meta-learning
2021 IEEE INTERNATIONAL CONFERENCE ON ACOUSTICS, SPEECH AND SIGNAL PROCESSING (ICASSP 2021)(2021)
Abstract
Transfer learning from a multilingual model has shown favorable results on low-resource automatic speech recognition (ASR). However, full-model fine-tuning generates a separate model for every target language and is not suitable for deploying and maintaining in production. The key challenge lies in how to efficiently extend the pre-trained model with fewer parameters. In this paper, we propose to combine the adapter module with meta-learning algorithms to achieve high recognition performance under low-resource settings and improve the parameter-efficiency of the model. Extensive experiments show that our methods can achieve comparable or even superior recognition rates than the state-of-the-art baselines on low-resource languages, especially under very-low-resource conditions, with a significantly smaller model profile.
MoreTranslated text
Key words
speech recognition,low-resource,cross-lingual,efficient adaptation,meta-learning
AI Read Science
Must-Reading Tree
Example
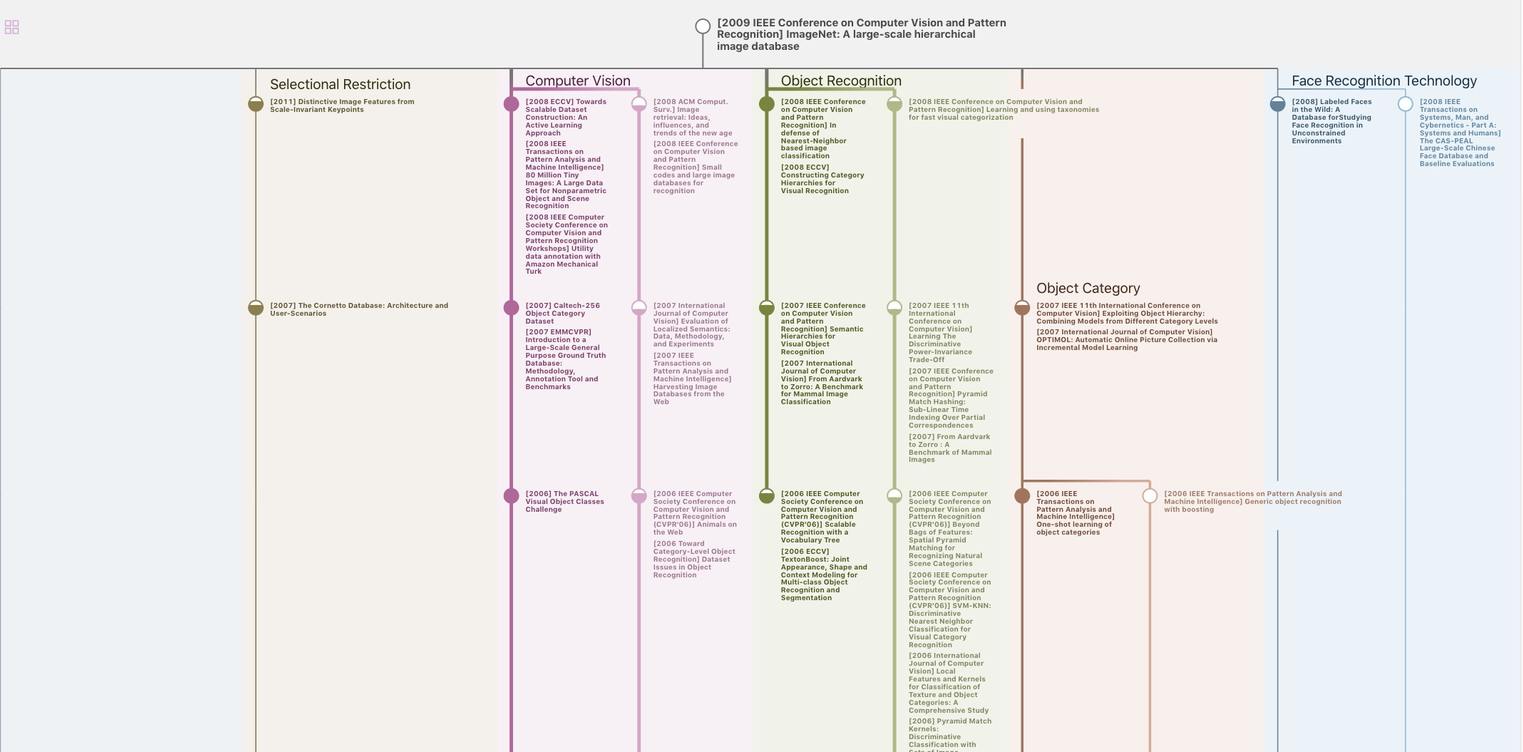
Generate MRT to find the research sequence of this paper
Chat Paper
Summary is being generated by the instructions you defined