FEDERATED ALGORITHM WITH BAYESIAN APPROACH: OMNI-FEDGE
2021 IEEE INTERNATIONAL CONFERENCE ON ACOUSTICS, SPEECH AND SIGNAL PROCESSING (ICASSP 2021)(2021)
摘要
In this paper, we consider the problem of Federated Learning (FL) under non-i.i.d data setting. We provide an improved estimate of the empirical loss at each node by using a weighted average of losses across nodes with a penalty term. These uneven weights to different nodes are assigned by taking a novel Bayesian approach to the problem where the problem of learning for each device/node is cast as maximizing the likelihood of a joint distribution. This joint distribution is for losses of nodes obtained by using data across devices for a given neural network of a node. We then provide a PAC learning guarantee on the objective function which reveals that the true average risk is no more than the proposed objective and the error term. We leverage this guarantee to propose an algorithm called Omni-Fedge. Using MNIST and Fashion MNIST datasets, we show that the performance of the proposed algorithm is significantly better than existing algorithms.
更多查看译文
关键词
Federated Learning, Neural Network, Bayesian Approach, Distributed Machine Learning, PAC Learning
AI 理解论文
溯源树
样例
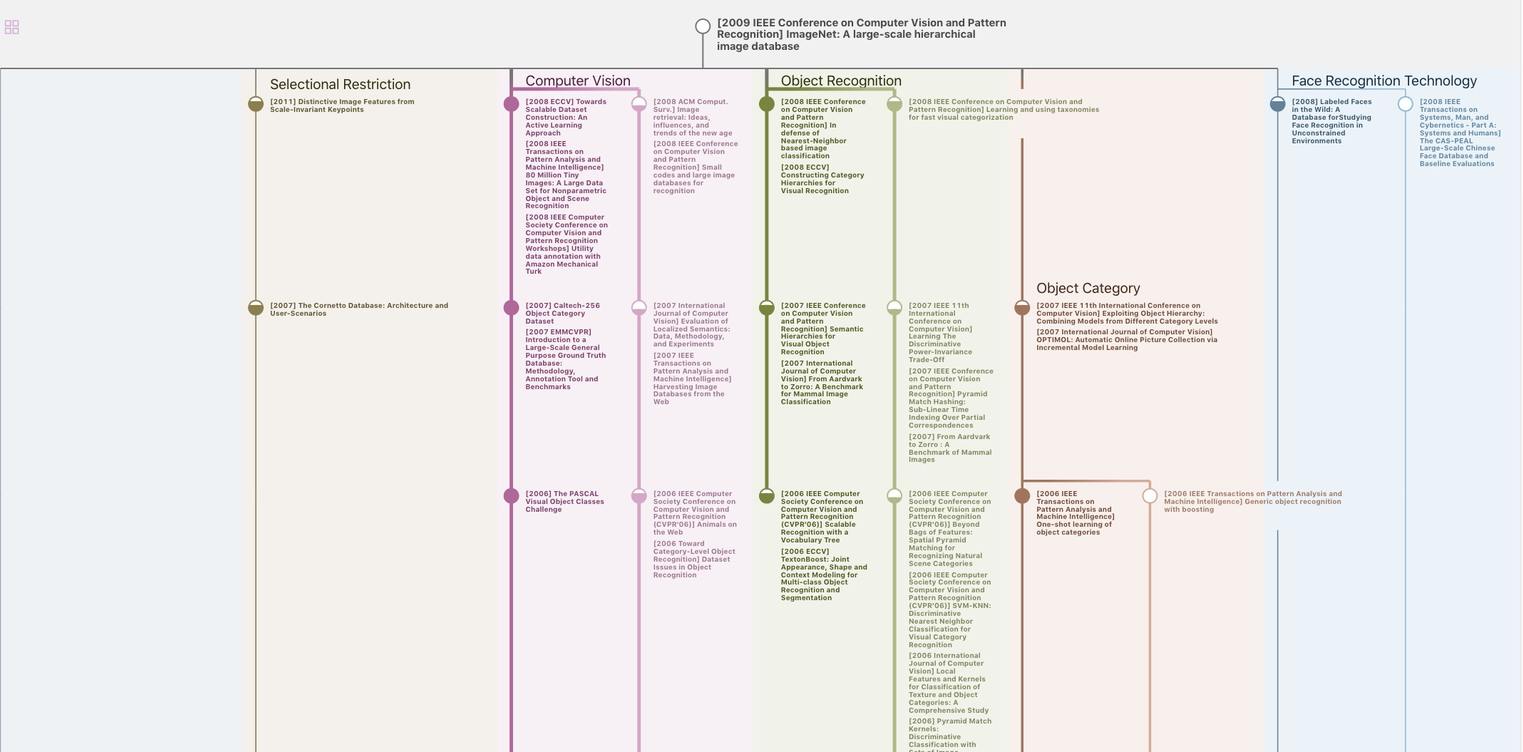
生成溯源树,研究论文发展脉络
Chat Paper
正在生成论文摘要