Day-Ahead Optimal Strategy For Commercial Air-Conditioning Load Under Time-Of-Use And Demand Pricing Plan
INTERNATIONAL TRANSACTIONS ON ELECTRICAL ENERGY SYSTEMS(2021)
摘要
We study the optimal operation of air-conditioning loads for commercial consumers based on the time-of-use (TOU) ($/kWh) and demand ($/kW) pricing plan. The problem is formulated as a dynamic program that aims to adjust consumer needs for electricity charges and comfort flexibly. Moreover, the Monte Carlo method is used to imitate the environmental uncertainties due to predicted outdoor temperatures. To determine the day-ahead temperature schedules for air-conditioning loads, a quantum-behaved particle swarm optimization based on space compression strategy (QPSO-SC) is presented in this paper. Space compression and particle re-initialization by chaotic initialization enhance the performance of the QPSO-SC. Finally, we test the proposed method for the TOU and demand pricing plan from Duke Energy. Thermostatical, daily energy charge saving, and monthly charge saving strategies are first compared, and the QPSO-SC is further compared with the particle swarm optimization, genetic algorithm, differential evolution, and other optimization algorithms. From the extensive simulations, the QPSO-SC is observed to be capable of yielding higher-quality solutions stably and efficiently than the other optimization algorithms; the proposed optimal strategy can also achieve a better balance between electricity charge and customer comfort, considering environmental uncertainties.
更多查看译文
关键词
air‐, conditioning loads, customer comfort, environmental uncertainties, TOU and demand pricing plan, QPSO‐, SC
AI 理解论文
溯源树
样例
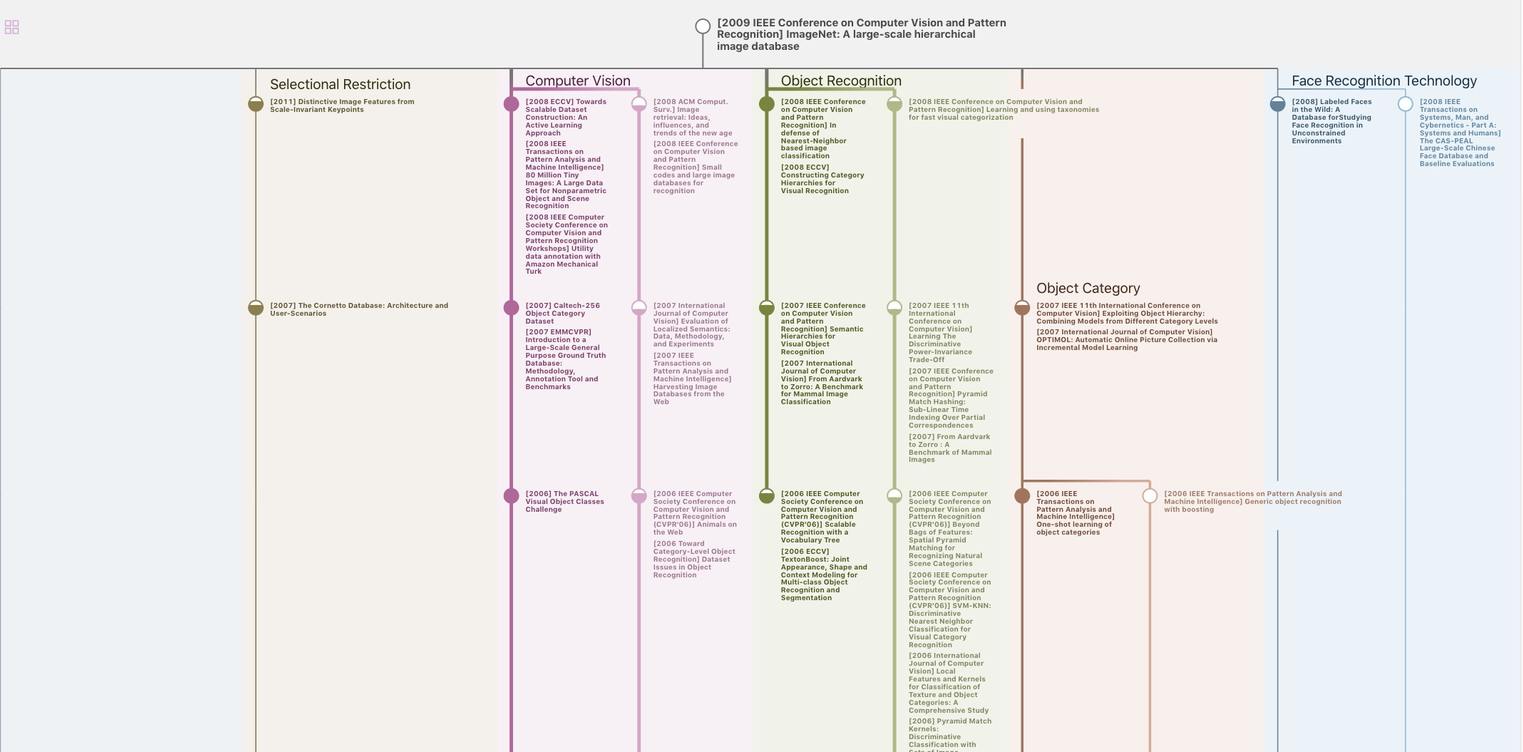
生成溯源树,研究论文发展脉络
Chat Paper
正在生成论文摘要