Analyzing Topic Transitions in Text-Based Social Cascades Using Dual-Network Hawkes Process
ADVANCES IN KNOWLEDGE DISCOVERY AND DATA MINING, PAKDD 2021, PT I(2021)
摘要
We address the problem of modeling bursty diffusion of text-based events over a social network of user nodes. The purpose is to recover, disentangle and analyze overlapping social conversations from the perspective of user-topic preferences, user-user connection strengths and, importantly, topic transitions. For this, we propose a Dual-Network Hawkes Process (DNHP), which executes over a graph whose nodes are user-topic pairs, and closeness of nodes is captured using topic-topic, a user-user, and user-topic interactions. No existing Hawkes Process model captures such multiple interactions simultaneously. Additionally, unlike existing Hawkes Process based models, where event times are generated first, and event topics are conditioned on the event times, the DNHP is more faithful to the underlying social process by making the event times depend on interacting (user, topic) pairs. We develop a Gibbs sampling algorithm for estimating the three network parameters that allows evidence to flow between the parameter spaces. Using experiments over large real collection of tweets by US politicians, we show that the DNHP generalizes better than state of the art models, and also provides interesting insights about user and topic transitions.
更多查看译文
关键词
Network Hawkes process, Generative models, Gibbs sampling
AI 理解论文
溯源树
样例
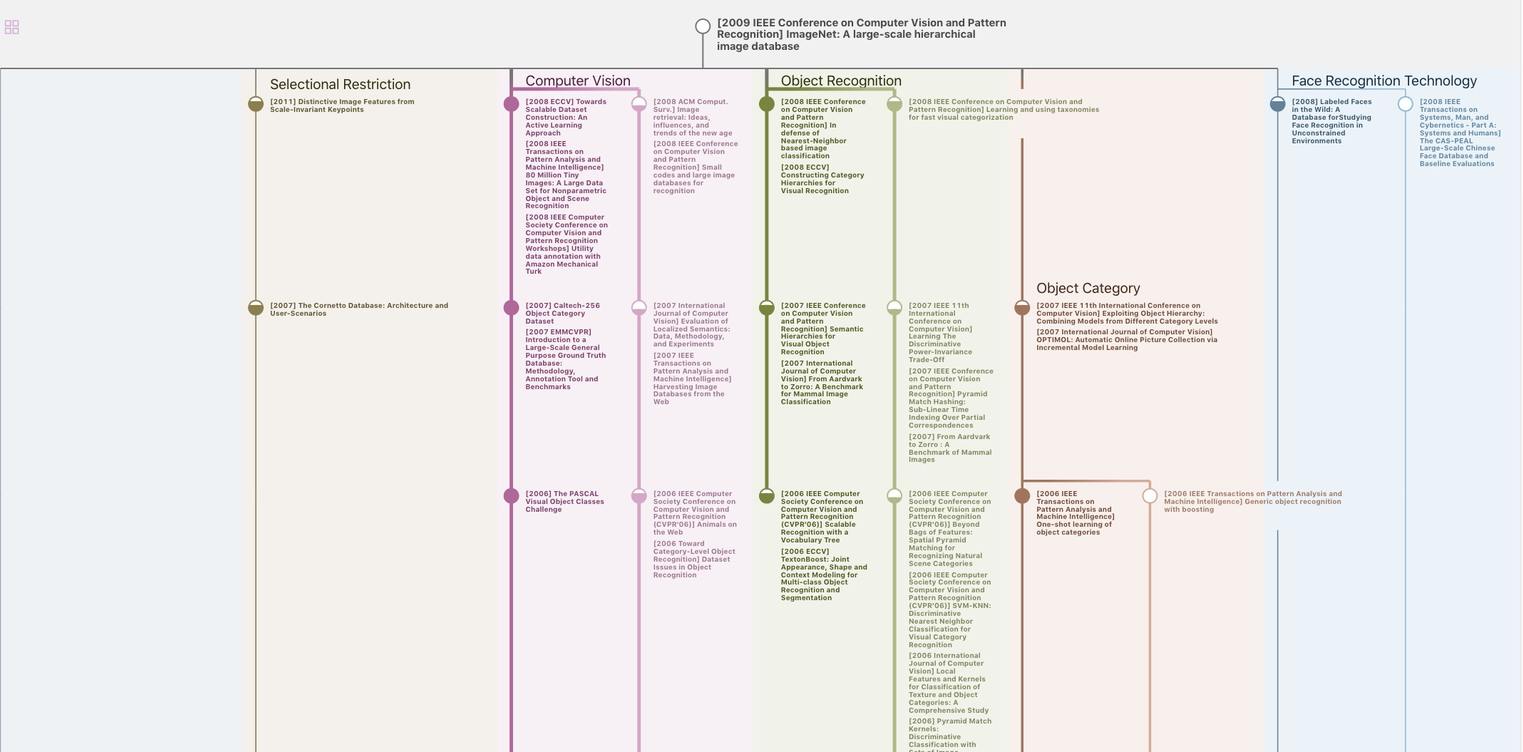
生成溯源树,研究论文发展脉络
Chat Paper
正在生成论文摘要