Development of LSTM Networks for Predicting Viscoplasticity With Effects of Deformation, Strain Rate, and Temperature History
JOURNAL OF APPLIED MECHANICS-TRANSACTIONS OF THE ASME(2021)
摘要
In this paper, long short-term memory (LSTM) networks are used in an original way to model the behavior of a viscoplastic material solicited under changing loading conditions. The material behavior is dependent on the history effects of plasticity which can be visible during strain rate jumps or temperature changes. Due to their architecture and internal state (memory), the LSTM networks have the ability to remember past data to update their current state, unlike the traditional artificial neural networks (ANNs) which fail to capture history effects. Specific LSTM networks are designed and trained to reproduce the complex behavior of a viscoplastic solder alloy subjected to strain rate jumps, temperature changes, or loading-unloading cycles. The training data sets are numerically generated using the constitutive viscoplastic law of Anand which is very popular for describing solder alloys. The Anand model serves also as a reference to evaluate the performances of the LSTM networks on new data. It is demonstrated that this class of networks is remarkably well suited for replicating the history plastic effects under all the tested loading conditions.
更多查看译文
关键词
deep learning,viscoplastic behavior,plasticity history,LSTM network,memory,data-driven modeling,computational mechanics,constitutive modeling of materials,mechanical properties of materials,stress analysis
AI 理解论文
溯源树
样例
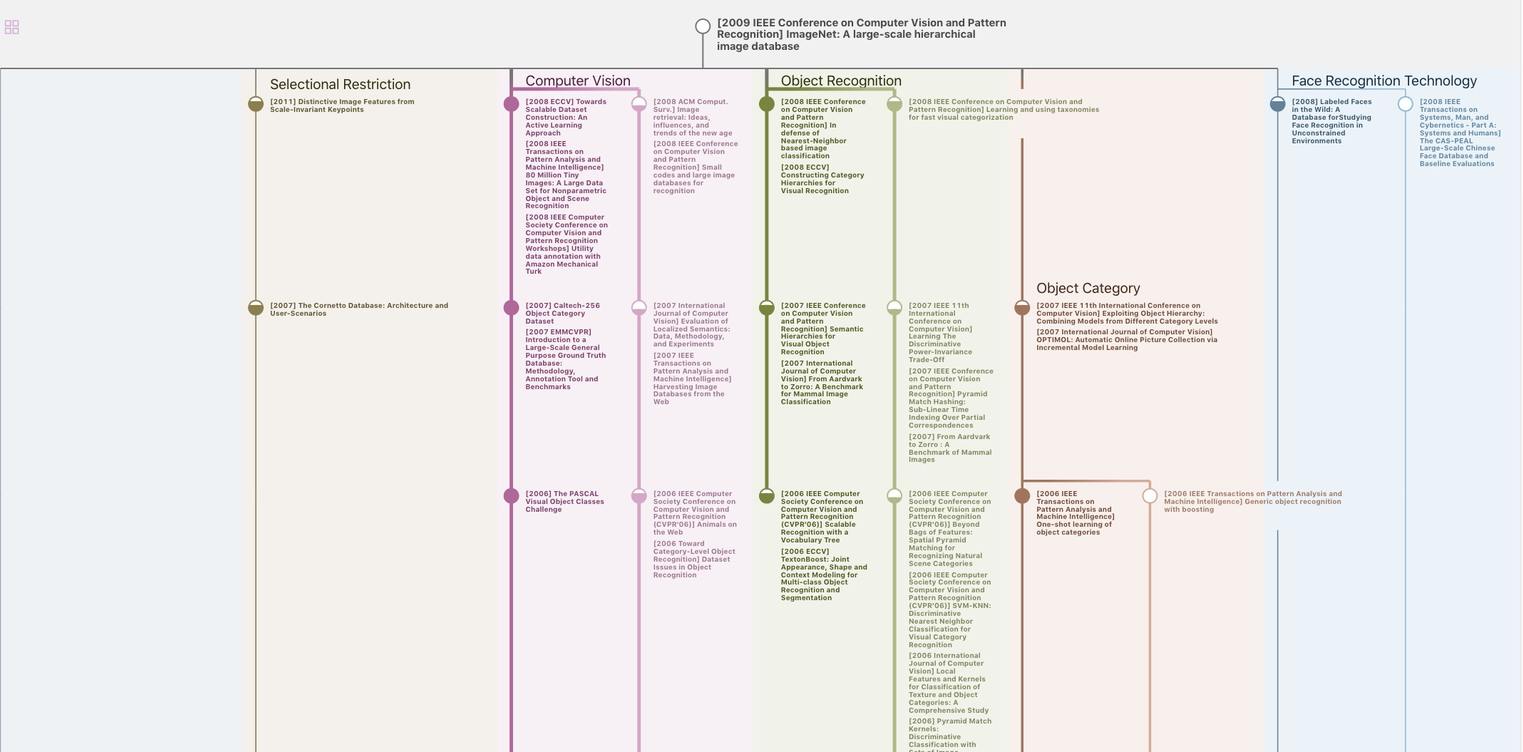
生成溯源树,研究论文发展脉络
Chat Paper
正在生成论文摘要