A new active learning approach for global optimization of atomic clusters
Theoretical Chemistry Accounts(2021)
摘要
In catalysis, an accurate structural elucidation of molecules, atomic clusters, nanoparticles and solid surfaces is required to understand chemical processes. Therefore, an efficient and automatic structure determination for these systems is of great benefit since it requires a global search within huge chemical spaces. In this work, we propose a new active learning (AL) method intended for atomic clusters that uses different supervised machine learning techniques and their uncertainties to decide the promising non-observed (virtual) structures to be evaluated from quantum calculations. The method was developed for structural elucidation of (I) clusters where all atomic coordinates are allowed to change in a continuous chemical space and (II) doped ones where the atoms exchange in a sufficiently rigid structure, thus, a discrete search space where all cluster descriptors are known. Particularly for case I, a genetic algorithm operator was used to create the unknown virtual structures (and then their descriptors) from the observed ones to improve the performance of the AL search. The proposed AL was applied to the global optimization of heteronuclear (Al 4 Si 7 and 4Al@Si 11 ) and homonuclear (Na 20 ) clusters using self-consistent charge density-functional tight-binding (SCC-DFTB), where a new repulsion parameter was developed to reproduce isomers evaluated from high-level calculations. The performance of the Gaussian process and artificial neural network algorithms was evaluated together with several uncertainty quantification methods: from Gaussian process, K-fold cross-validation and nonparametric bootstrap (BS) resampling. The efficiency of the AL was compared to conventional global optimization methods, such as random search and a genetic algorithm. The results show that the AL can find efficiently the global minimum of atomic clusters.
更多查看译文
关键词
Clusters,Machine learning,Active learning,Efficient global optimization,SCC-DFTB
AI 理解论文
溯源树
样例
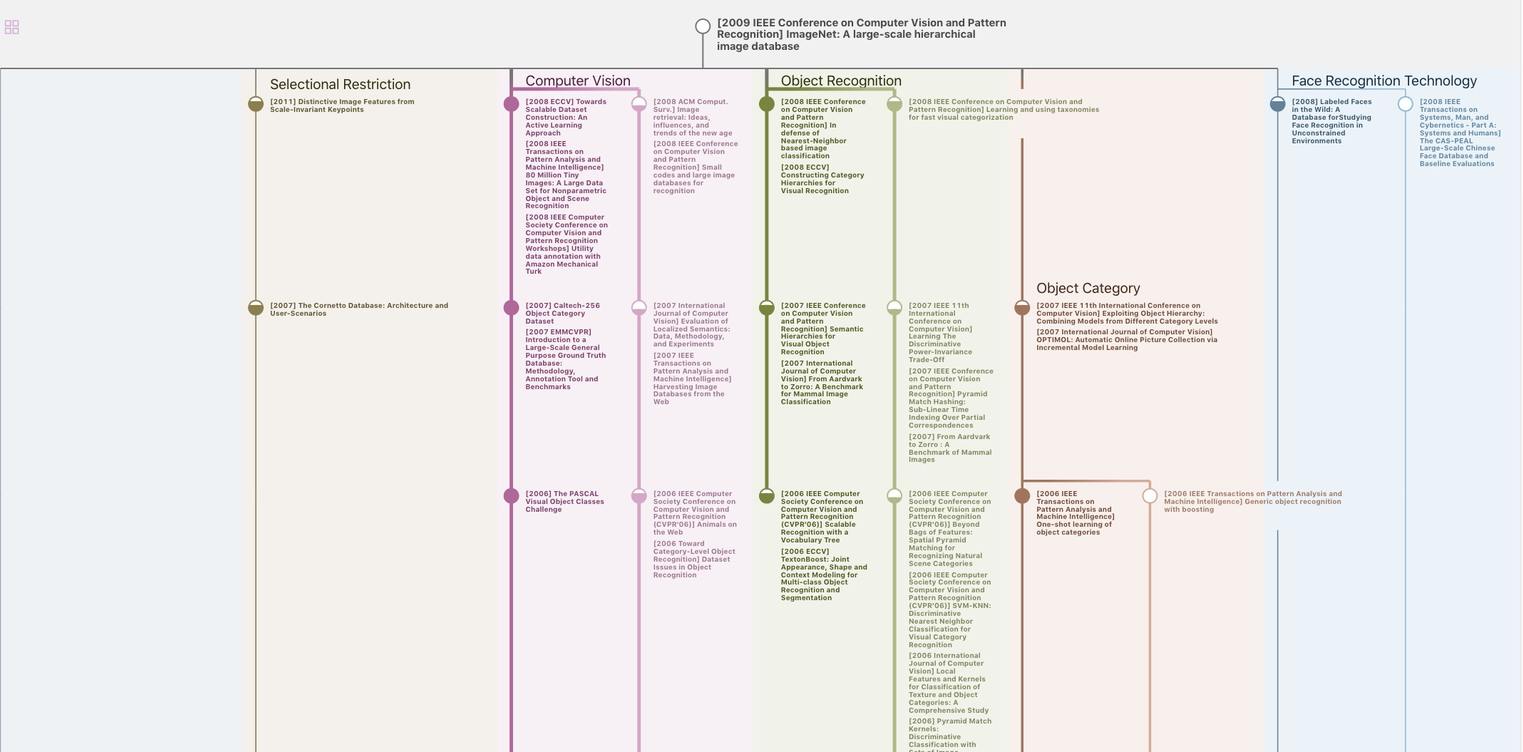
生成溯源树,研究论文发展脉络
Chat Paper
正在生成论文摘要