Meta Joint Optimization: a Holistic Framework for Noisy-Labeled Visual Recognition
Applied intelligence(2021)
摘要
Collecting large-scale data with clean labels for supervised training is practically challenging. It is easier to collect a dataset with noisy labels, but such noise may degrade the performance of deep neural networks (DNNs). This paper targets at this challenge by wisely leveraging both relatively clean data and relatively noisy data. In this work, we propose meta-joint optimization (MJO), a novel and holistic framework for learning with noisy labels. The framework can jointly learn DNN parameters and correct noisy labels. We first estimate the label quality and conduct data division which dynamically divides the training data into relatively clean data and relatively noisy data. To better optimize the DNN parameters, we regard the relatively noisy data as an unlabeled set and further apply interpolation consistency training in a semi-supervised manner for information reuse of relatively noisy data. For better label optimization, we propose centroid-induced label updating to optimize noisy labels themselves. Concretely, we calculate the centroids of each class based on the relatively clean data and update labels of relatively noisy data. Finally, we conduct experiments on both synthetic and real-world datasets. The results demonstrate the advantageous performance of the proposed method compared to state-of-the-art baselines.
更多查看译文
关键词
Noisy labels,Image classification,Meta learning,Label correction
AI 理解论文
溯源树
样例
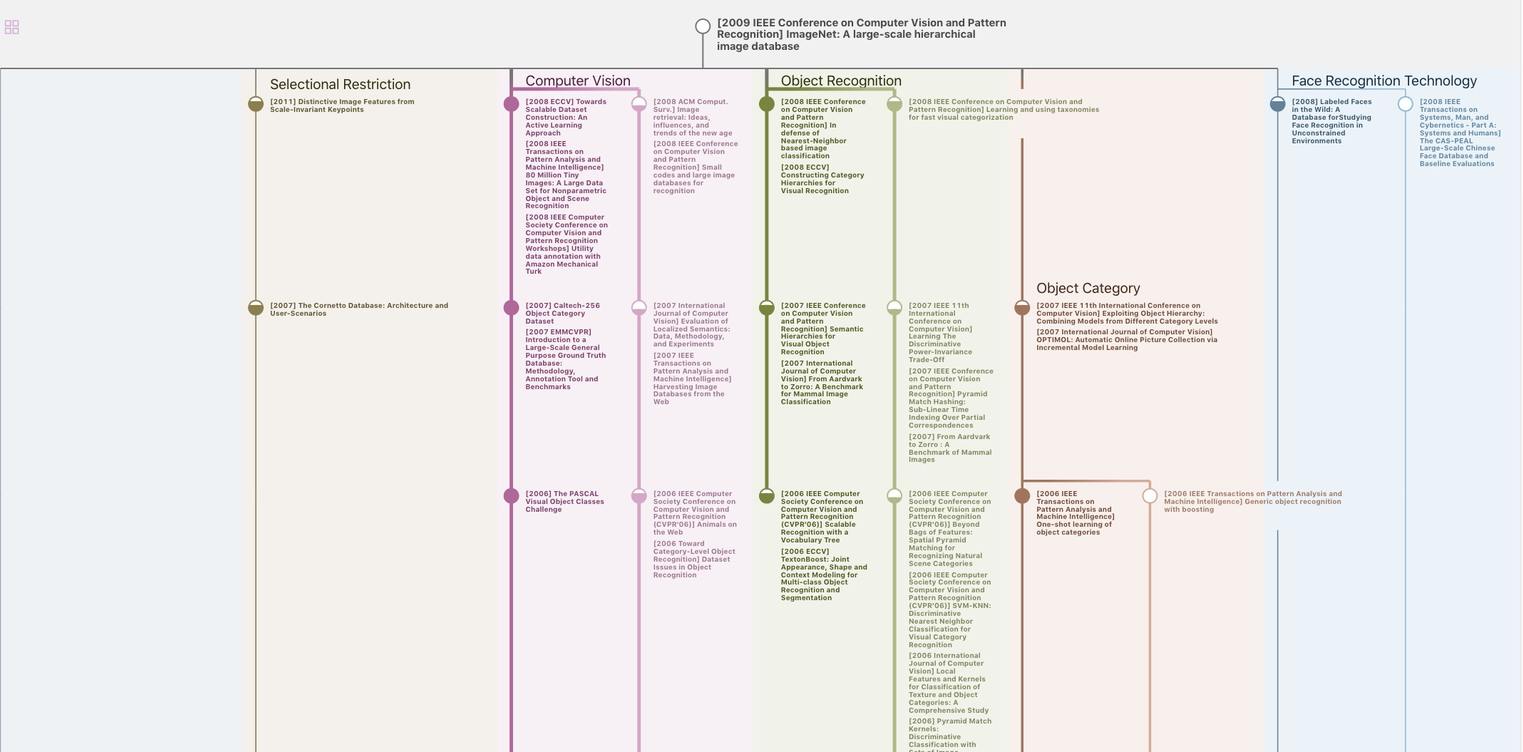
生成溯源树,研究论文发展脉络
Chat Paper
正在生成论文摘要