Using Population Crossover Trials To Improve The Decision Process Regarding Treatment Individualization In N-Of-1 Trials
STATISTICS IN MEDICINE(2021)
摘要
Healthcare researchers are showing renewed interest in the utilization of N-of-1 clinical trials for the individualization of pharmacological treatments. Here, we propose a frequentist approach to conducting treatment individualization in N-of-1 trials that we call "partial empirical Bayes." We infer the most beneficial treatment for the patient from combining the information provided by a previously conducted population crossover trial with individual patient data. We propose a method for estimating an optimal number of treatment cycles and investigate the statistical conditions under which N-of-1 trials are more beneficial than traditional clinical approaches. We represent the patient population with a random-coefficients linear model and calculate estimators of posttreatment individual disease severities. We show the estimators' consistency under the most common N-of-1 designs and examine their prediction errors and performance with small numbers of patient's responses. We demonstrate by simulating new patients that our approach is equivalent or superior to both the common clinical practice of recommending the on-average best treatment for all patients and the common individualization method that simply compares average responses to the tested treatments. We conclude that some situations exist in which individualization with N-of-1 trials is highly beneficial while other situations exist in which individualization may be unfruitful.
更多查看译文
关键词
crossover trials, empirical Bayes, individual treatment benefits, N-of-1 trials, random effects linear models, variance components
AI 理解论文
溯源树
样例
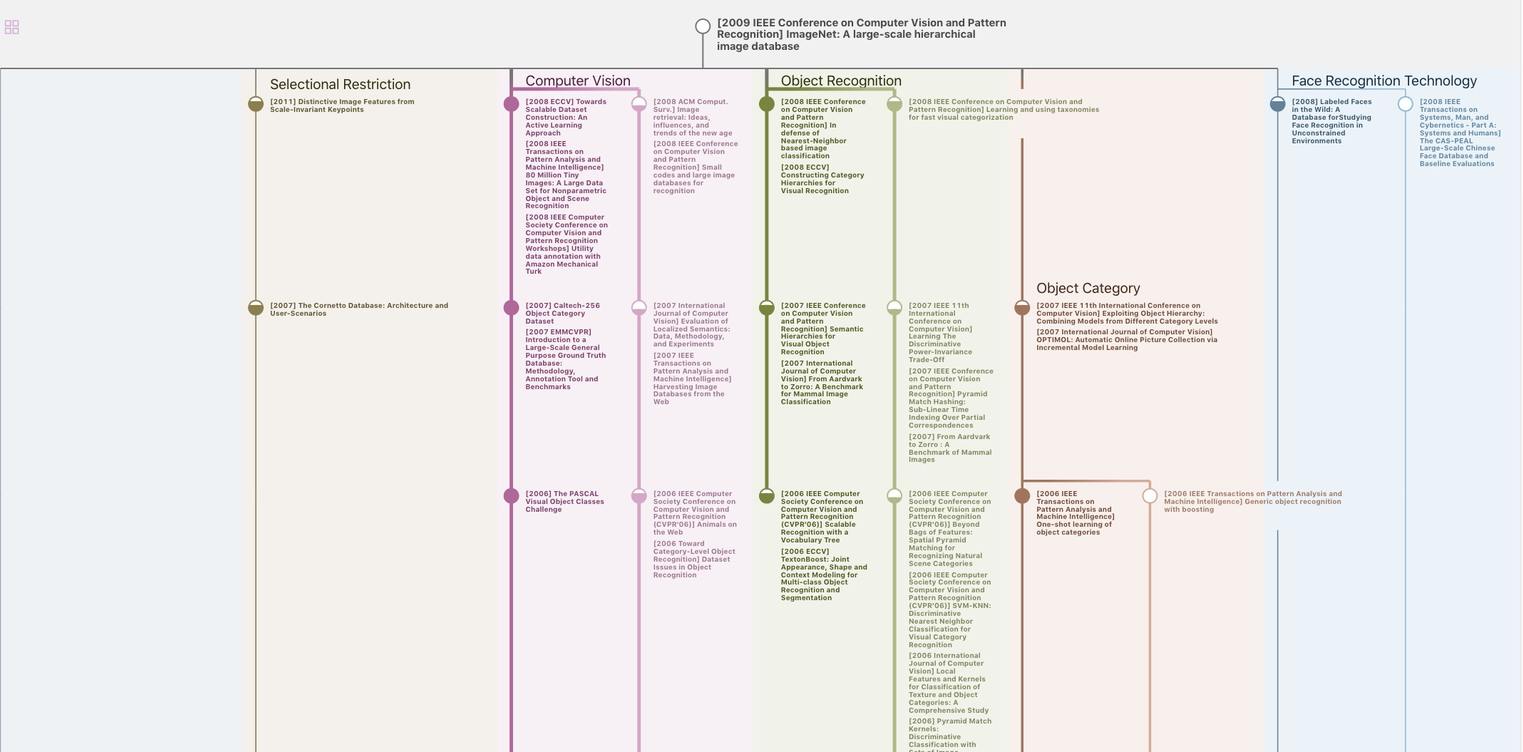
生成溯源树,研究论文发展脉络
Chat Paper
正在生成论文摘要