Analysis Of Autoencoders For Network Intrusion Detection
SENSORS(2021)
摘要
As network attacks are constantly and dramatically evolving, demonstrating new patterns, intelligent Network Intrusion Detection Systems (NIDS), using deep-learning techniques, have been actively studied to tackle these problems. Recently, various autoencoders have been used for NIDS in order to accurately and promptly detect unknown types of attacks (i.e., zero-day attacks) and also alleviate the burden of the laborious labeling task. Although the autoencoders are effective in detecting unknown types of attacks, it takes tremendous time and effort to find the optimal model architecture and hyperparameter settings of the autoencoders that result in the best detection performance. This can be an obstacle that hinders practical applications of autoencoder-based NIDS. To address this challenge, we rigorously study autoencoders using the benchmark datasets, NSL-KDD, IoTID20, and N-BaIoT. We evaluate multiple combinations of different model structures and latent sizes, using a simple autoencoder model. The results indicate that the latent size of an autoencoder model can have a significant impact on the IDS performance.
更多查看译文
关键词
IDS, NIDS, ML-NIDS, autoencoders, deep-learning models, model design, unsupervised learning Algorithms, IoT
AI 理解论文
溯源树
样例
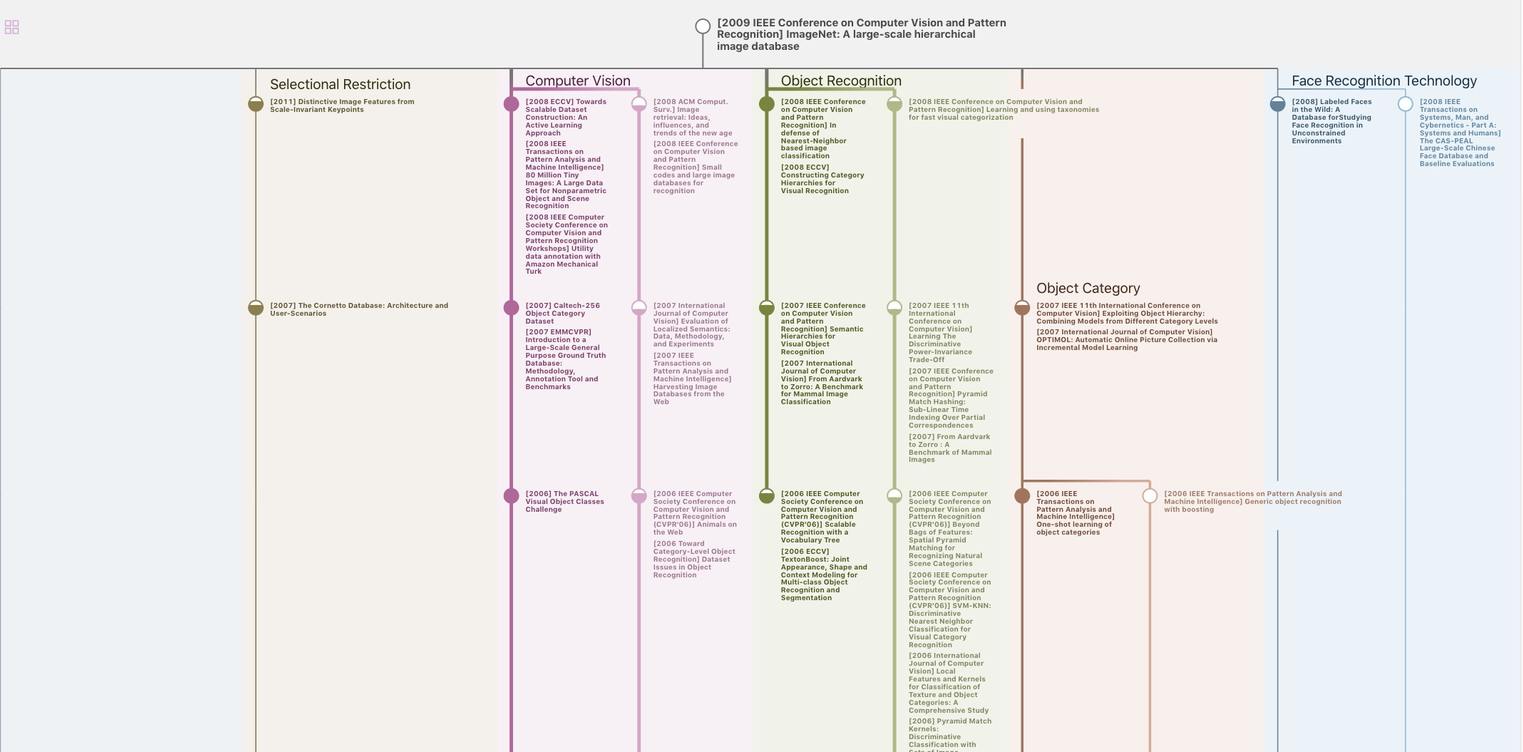
生成溯源树,研究论文发展脉络
Chat Paper
正在生成论文摘要