Implicit Acceleration and Feature Learning inInfinitely Wide Neural Networks with Bottlenecks
arxiv(2021)
摘要
We analyze the learning dynamics of infinitely wide neural networks with a finite sized bottle-neck. Unlike the neural tangent kernel limit, a bottleneck in an otherwise infinite width network al-lows data dependent feature learning in its bottle-neck representation. We empirically show that a single bottleneck in infinite networks dramatically accelerates training when compared to purely in-finite networks, with an improved overall performance. We discuss the acceleration phenomena by drawing similarities to infinitely wide deep linear models, where the acceleration effect of a bottleneck can be understood theoretically.
更多查看译文
关键词
infinitely wide neural networks,bottlenecks,feature learning,neural networks
AI 理解论文
溯源树
样例
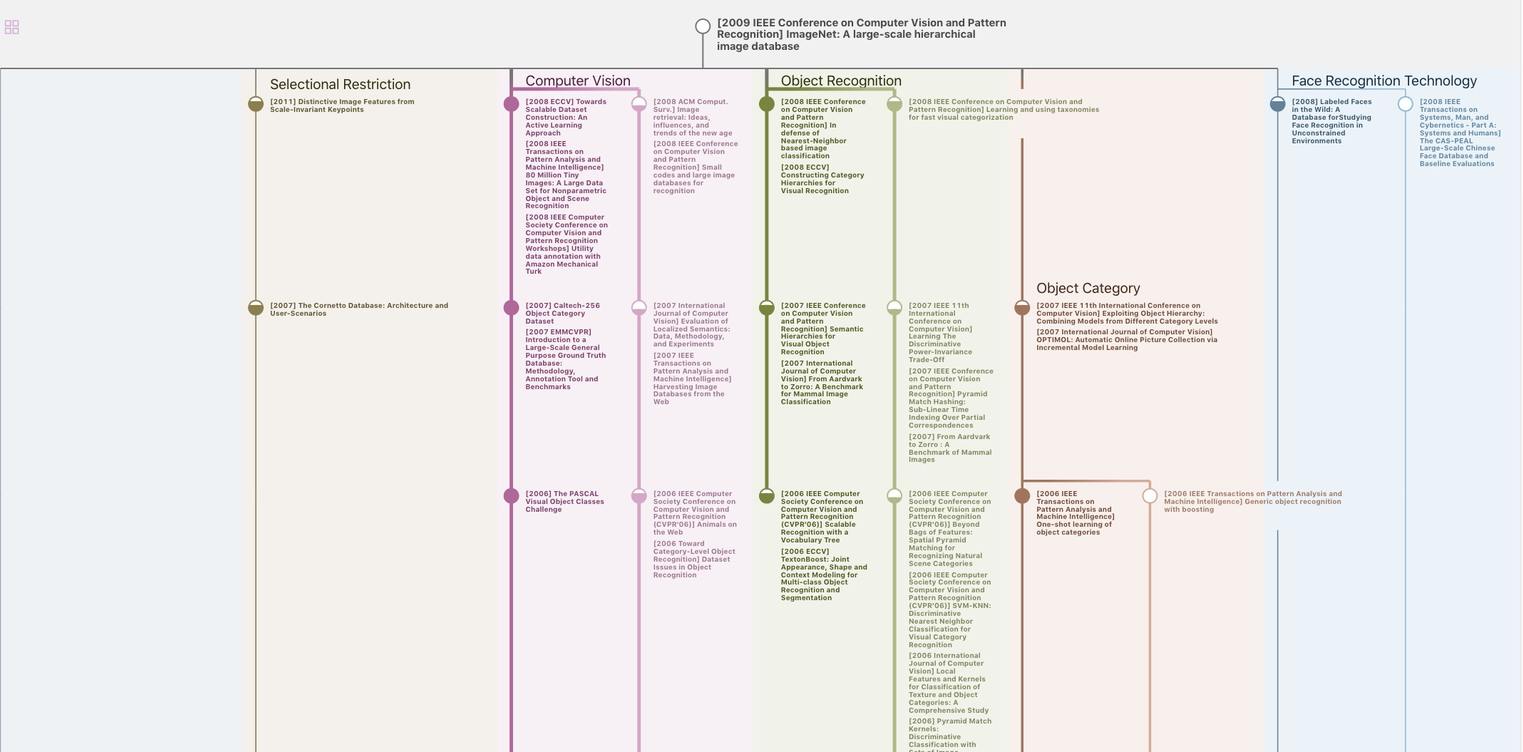
生成溯源树,研究论文发展脉络
Chat Paper
正在生成论文摘要