A low-query black-box adversarial attack based on transferability
Knowledge-Based Systems(2021)
摘要
Artificial intelligence systems suffer from black-box adversarial attacks recently. To prevent this kind of attack, a large amount of researches that reveal the nature of this attack has emerged. However, the query count, success rate, and distortion in the existing works cannot fully satisfy the practical purposes. In this paper, we propose a low-query black-box adversarial attack based on transferability by combining the optimization-based method and the transfer-based method. Our approach aims to improve the black-box attack with a lower number of queries, higher success rate, and lower distortion. In addition, we make full use of surrogate models and optimize the objective function to further improve the performance of our algorithm. We verified our method on MNIST (Lecun and Bottou, 1998) [1], CIFAR-10 (Krizhevsky et al., 2009) [2], and ImageNet (Deng et al. 2009) [3], respectively. Experimental results demonstrate that our method can implement a black-box attack with more than 98.5% success rate and achieve specific distortion with less than 5% queries comparing with other state-of-the-art methods.
更多查看译文
关键词
Adversarial sample,Black-box attack,Transferability,Neural network
AI 理解论文
溯源树
样例
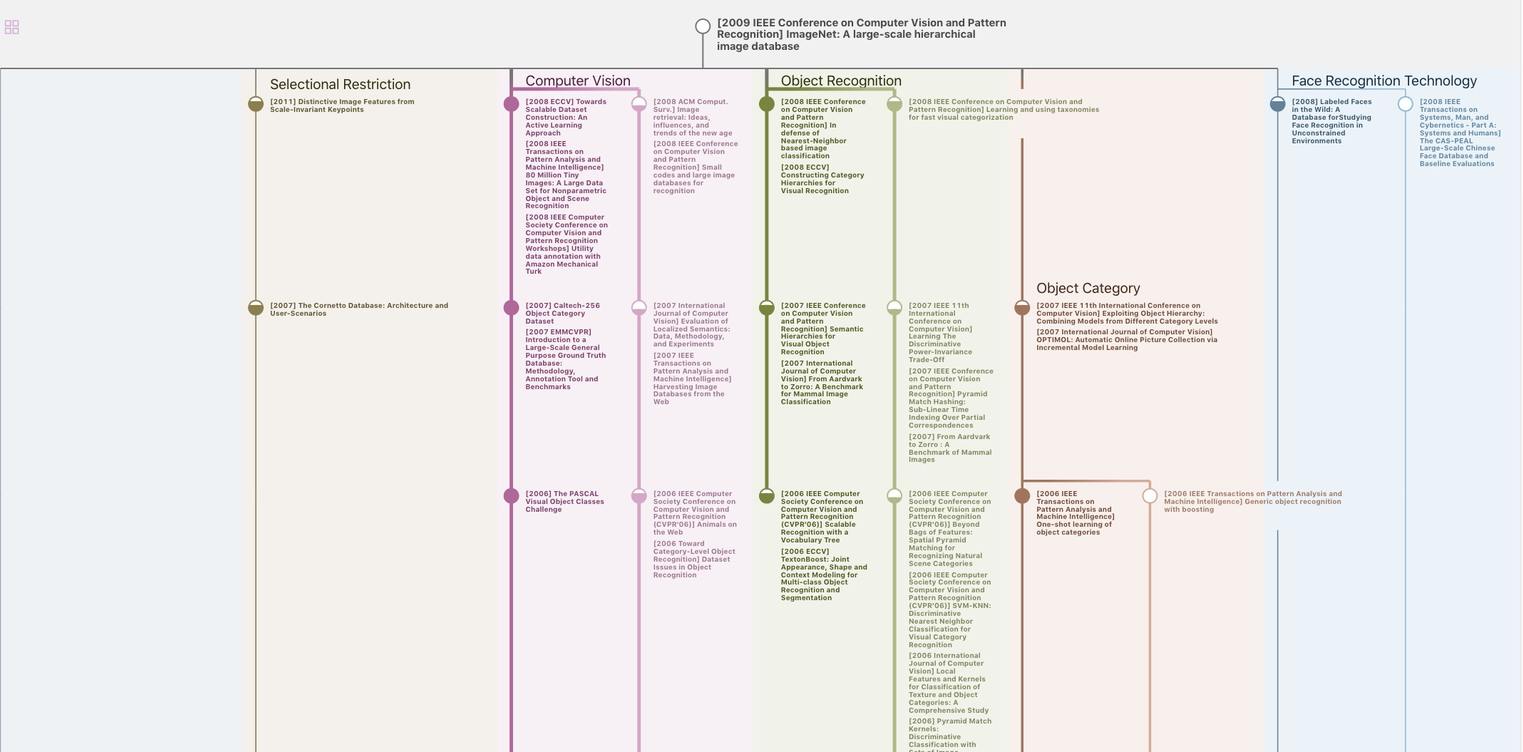
生成溯源树,研究论文发展脉络
Chat Paper
正在生成论文摘要