Classifying Regions of High Model Error Within a Data-Driven RANS Closure: Application to Wind Turbine Wakes
arXiv (Cornell University)(2022)
Abstract
Data-driven Reynolds-averaged Navier–Stokes (RANS) turbulence closures are increasing seen as a viable alternative to general-purpose RANS closures, when LES reference data is available—also in wind-energy. Parsimonious closures with few, simple terms have advantages in terms of stability, interpret-ability, and execution speed. However experience suggests that closure model corrections need be made only in limited regions—e.g. in the near-wake of wind turbines and not in the majority of the flow. A parsimonious model therefore must find a middle ground between precise corrections in the wake, and zero corrections elsewhere. We attempt to resolve this impasse by introducing a classifier to identify regions needing correction, and only fit and apply our model correction there. We observe that such classifier-based models are significantly simpler (with fewer terms) than models without a classifier, and have similar accuracy, but are more prone to instability. We apply our framework to three flows consisting of multiple wind-turbines in neutral conditions with interacting wakes.
MoreTranslated text
Key words
wind,high model error,data-driven
AI Read Science
Must-Reading Tree
Example
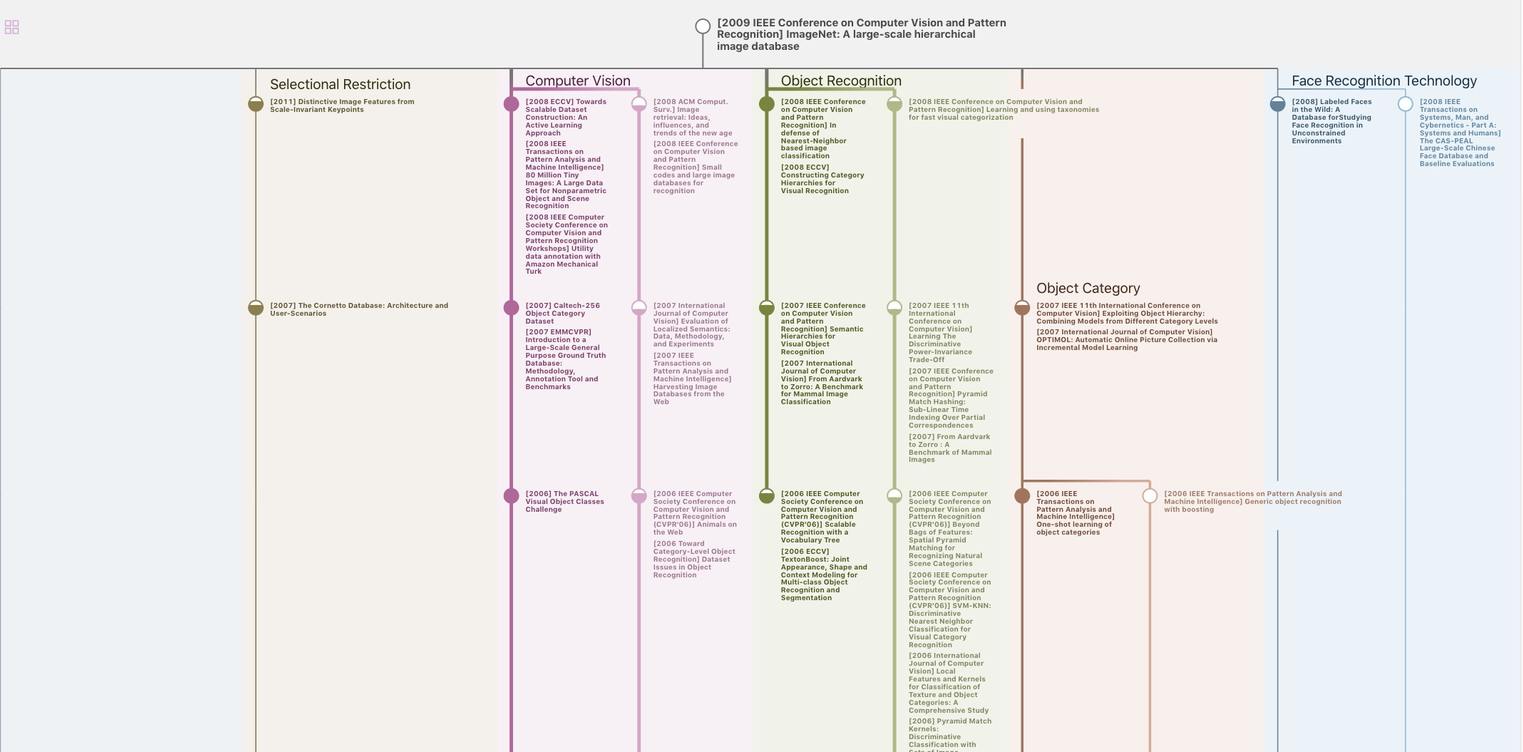
Generate MRT to find the research sequence of this paper
Chat Paper
Summary is being generated by the instructions you defined