Melt Pool Segmentation For Additive Manufacturing: A Generative Adversarial Network Approach
COMPUTERS & ELECTRICAL ENGINEERING(2021)
Abstract
Additive manufacturing (AM) is a popular manufacturing technique which is broadly exploited in rapid prototyping and fabricating components with complex geometries. To ensure the stability of the AM process, it is of critical importance to obtain high-quality thermal images by using image processing techniques. In this paper, a novel image processing method is put forward with aim to improve the contrast ratio of the thermal images for image segmentation. To be specific, an image-enhancement generative adversarial network (IEGAN) is developed, where a new objective function is designed for the training process. To verify the superiority and feasibility of the proposed IEGAN, the thermal images captured from an AM process are utilized for image segmentation. Experiment results demonstrate that the developed IEGAN outperforms the original GAN in improving the contrast ratio of the thermal images.
MoreTranslated text
Key words
Additive manufacturing, Generative adversarial network, Defect detection, Image processing, Image segmentation, Thermal image
AI Read Science
Must-Reading Tree
Example
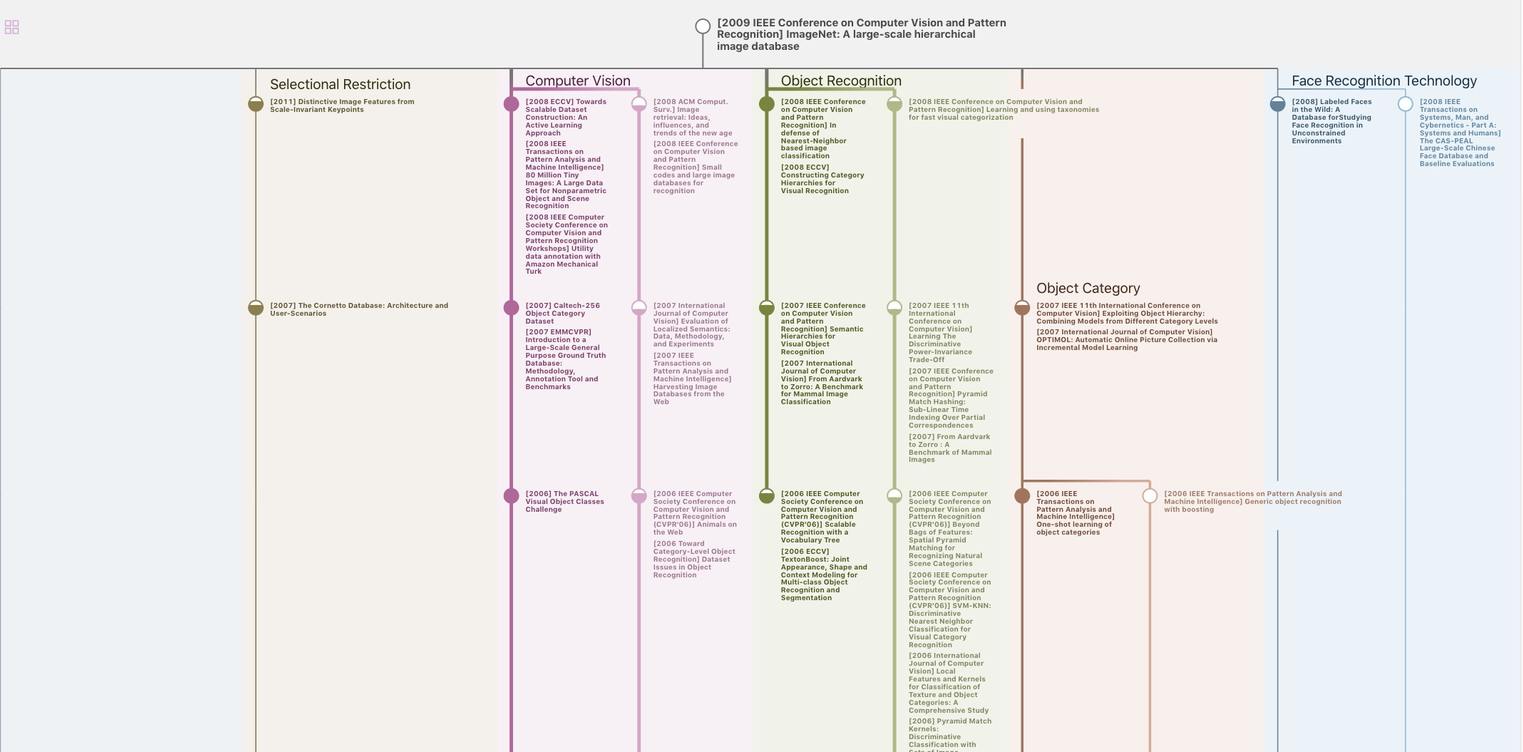
Generate MRT to find the research sequence of this paper
Chat Paper
Summary is being generated by the instructions you defined