Model-Advantage Optimization for Model-Based Reinforcement Learning
CoRR(2021)
摘要
Model-based Reinforcement Learning (MBRL) algorithms have been traditionally designed with the goal of learning accurate dynamics of the environment. This introduces a mismatch between the objectives of model-learning and the overall learning problem of finding an optimal policy. Value-aware model learning, an alternative model-learning paradigm to maximum likelihood, proposes to inform model-learning through the value function of the learnt policy. While this paradigm is theoretically sound, it does not scale beyond toy settings. In this work, we propose a novel value-aware objective that is an upper bound on the absolute performance difference of a policy across two models. Further, we propose a general purpose algorithm that modifies the standard MBRL pipeline -- enabling learning with value aware objectives. Our proposed objective, in conjunction with this algorithm, is the first successful instantiation of value-aware MBRL on challenging continuous control environments, outperforming previous value-aware objectives and with competitive performance w.r.t. MLE-based MBRL approaches.
更多查看译文
关键词
models,learning,model-advantage,value-aware,model-based
AI 理解论文
溯源树
样例
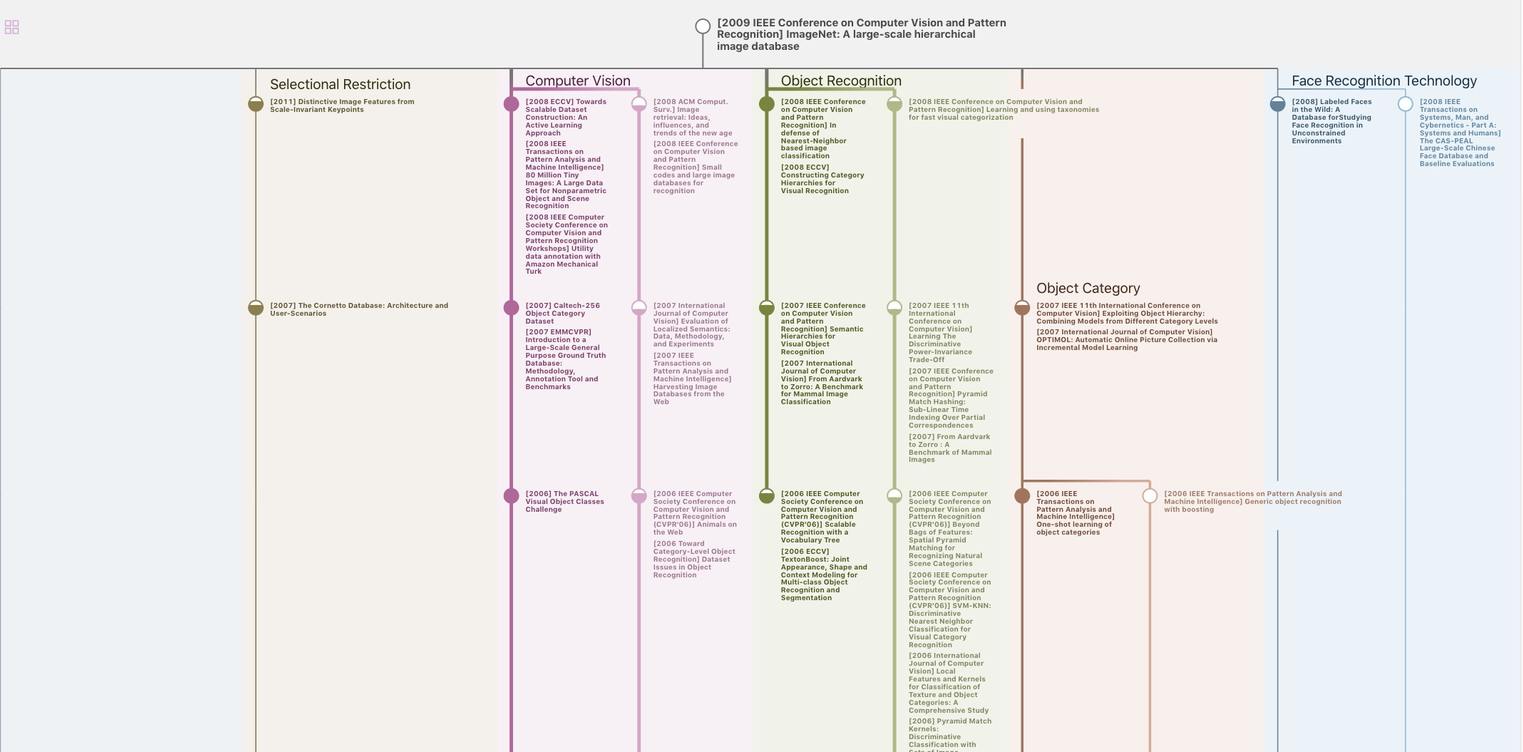
生成溯源树,研究论文发展脉络
Chat Paper
正在生成论文摘要