A Photonic-Circuits-Inspired Compact Network: Toward Real-Time Wireless Signal Classification at the Edge
arxiv(2021)
摘要
Machine learning (ML) methods are ubiquitous in wireless communication systems and have proven powerful for applications including radio-frequency (RF) fingerprinting, automatic modulation classification, and cognitive radio. However, the large size of ML models can make them difficult to implement on edge devices for latency-sensitive downstream tasks. In wireless communication systems, ML data processing at a sub-millisecond scale will enable real-time network monitoring to improve security and prevent infiltration. In addition, compact and integratable hardware platforms which can implement ML models at the chip scale will find much broader application to wireless communication networks. Toward real-time wireless signal classification at the edge, we propose a novel compact deep network that consists of a photonic-hardware-inspired recurrent neural network model in combination with a simplified convolutional classifier, and we demonstrate its application to the identification of RF emitters by their random transmissions. With the proposed model, we achieve 96.32% classification accuracy over a set of 30 identical ZigBee devices when using 50 times fewer training parameters than an existing state-of-the-art CNN classifier. Thanks to the large reduction in network size, we demonstrate real-time RF fingerprinting with 0.219 ms latency using a small-scale FPGA board, the PYNQ-Z1.
更多查看译文
关键词
compact network,wireless,photonic-circuits-inspired,real-time
AI 理解论文
溯源树
样例
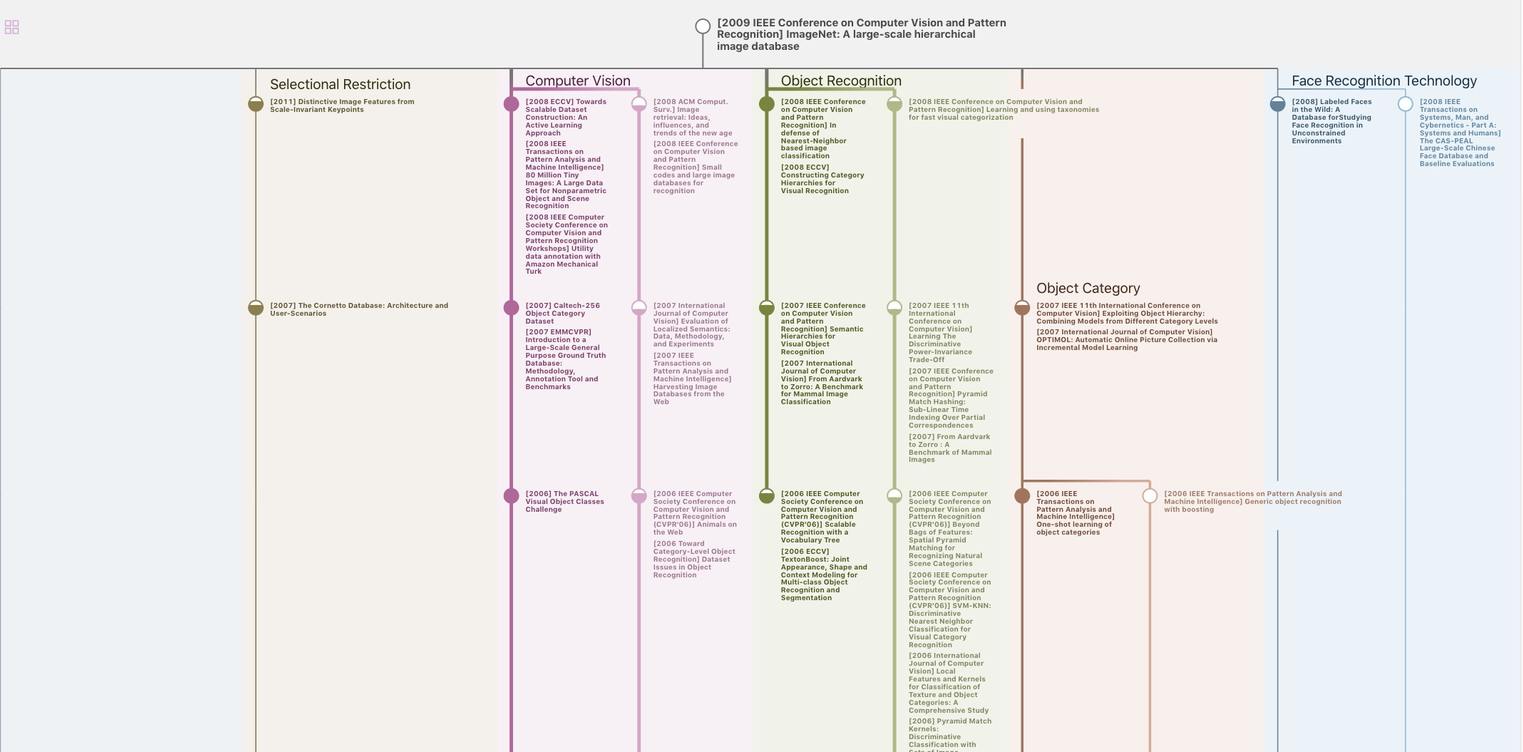
生成溯源树,研究论文发展脉络
Chat Paper
正在生成论文摘要