Longitudinal prediction of hospital-acquired acute kidney injury in COVID-19: a two-center study
INFECTION(2021)
Abstract
Background To investigate the temporal characteristics of clinical variables of hospital-acquired acute kidney injury (AKI) in COVID-19 patients and to longitudinally predict AKI onset. Methods There were 308 hospital-acquired AKI and 721 non-AKI (NAKI) COVID-19 patients from Stony Brook Hospital (New York, USA) data, and 72 hospital-acquired AKI and 303 NAKI COVID-19 patients from Tongji Hospital (Wuhan, China). Demographic, comorbidities, and longitudinal (3 days before and 3 days after AKI onset) clinical variables were used to compute odds ratios for and longitudinally predict hospital-acquired AKI onset. Results COVID-19 patients with AKI were more likely to die than NAKI patients (31.5% vs 6.9%, adjusted p < 0.001, OR = 4.67 [95% CI 3.1, 7.0], Stony Brook data). AKI developed on average 3.3 days after hospitalization. Procalcitonin was elevated prior to AKI onset ( p < 0.05), peaked, and remained elevated ( p < 0.05). Alanine aminotransferase, aspartate transaminase, ferritin, and lactate dehydrogenase peaked the same time as creatinine, whereas d -dimer and brain natriuretic peptide peaked a day later. C-reactive protein, white blood cell and lymphocyte showed group differences − 2 days prior ( p < 0.05). Top predictors were creatinine, procalcitonin, white blood cells, lactate dehydrogenase, and lymphocytes. They predicted AKI onset with areas under curves (AUCs) of 0.78, 0.66, and 0.56 at 0, − 1, and − 2 days prior, respectively. When tested on the Tongji Hospital data, the AUCs were 0.80, 0.79, and 0.77, respectively. Conclusions Time-locked longitudinal data provide insight into AKI progression. Commonly clinical variables reasonably predict AKI onset a few days prior. This work may lead to earlier recognition of AKI and treatment to improve clinical outcomes.
MoreTranslated text
Key words
SARS-CoV-2, AKI, d-Dimer, Lactate dehydrogenase, Multiorgan failure, Cytokine storm, Predictive model, Chronic kidney disease
AI Read Science
Must-Reading Tree
Example
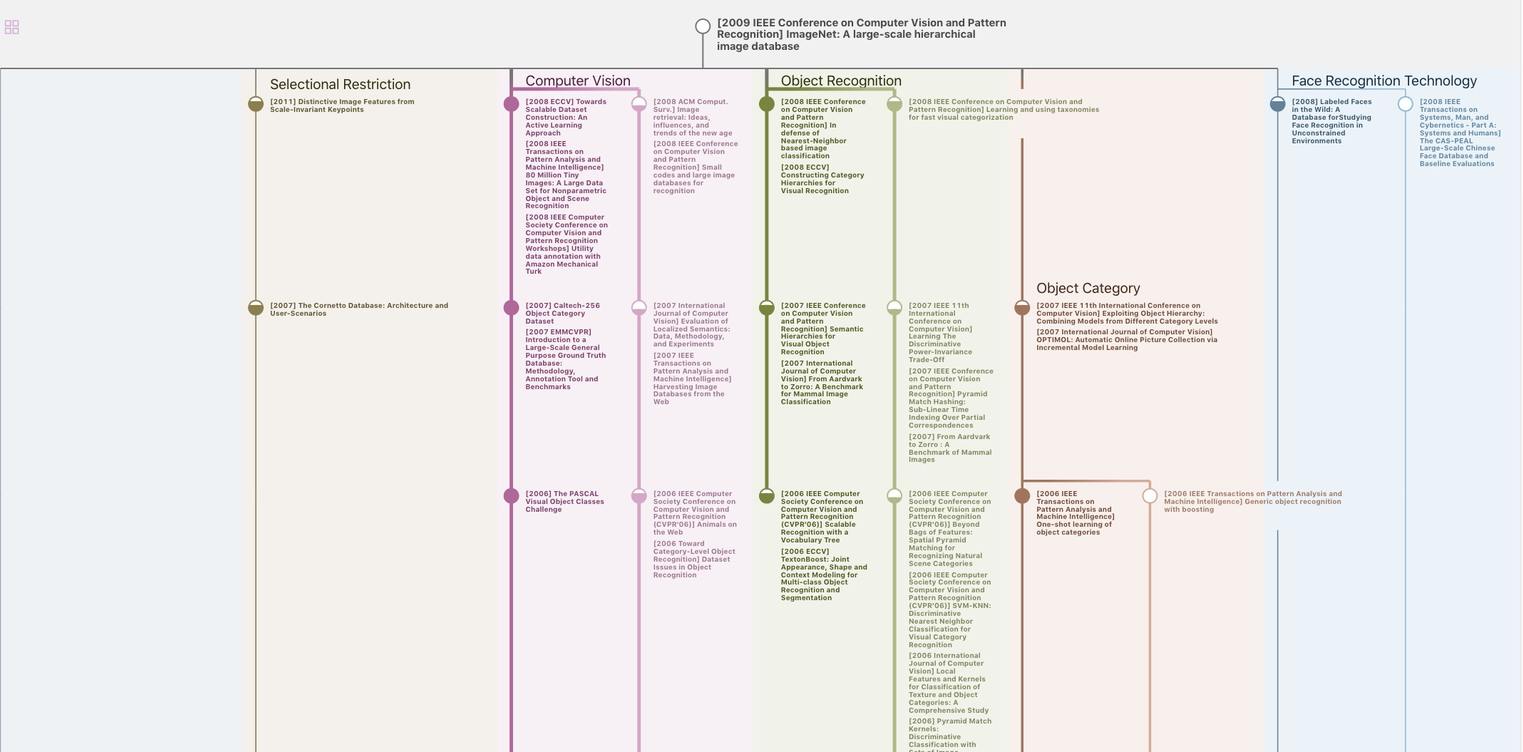
Generate MRT to find the research sequence of this paper
Chat Paper
Summary is being generated by the instructions you defined