Multivariate Time Series Anomaly Detection and Interpretation using Hierarchical Inter-Metric and Temporal Embedding
KDD(2021)
摘要
ABSTRACTAnomaly detection is a crucial task for monitoring various status (i.e., metrics) of entities (e.g., manufacturing systems and Internet services), which are often characterized by multivariate time series (MTS). In practice, it's important to precisely detect the anomalies, as well as to interpret the detected anomalies through localizing a group of most anomalous metrics, to further assist the failure troubleshooting. In this paper, we propose InterFusion, an unsupervised method that simultaneously models the inter-metric and temporal dependency for MTS. Its core idea is to model the normal patterns inside MTS data through hierarchical Variational AutoEncoder with two stochastic latent variables, each of which learns low-dimensional inter-metric or temporal embeddings. Furthermore, we propose an MCMC-based method to obtain reasonable embeddings and reconstructions at anomalous parts for MTS anomaly interpretation. Our evaluation experiments are conducted on four real-world datasets from different industrial domains (three existing and one newly published dataset collected through our pilot deployment of InterFusion). InterFusion achieves an average anomaly detection F1-Score higher than 0.94 and anomaly interpretation performance of 0.87, significantly outperforming recent state-of-the-art MTS anomaly detection methods.
更多查看译文
关键词
Anomaly Detection, Multivariate Time Series, Hierarchical Structure, Inter-metric and Temporal Embedding
AI 理解论文
溯源树
样例
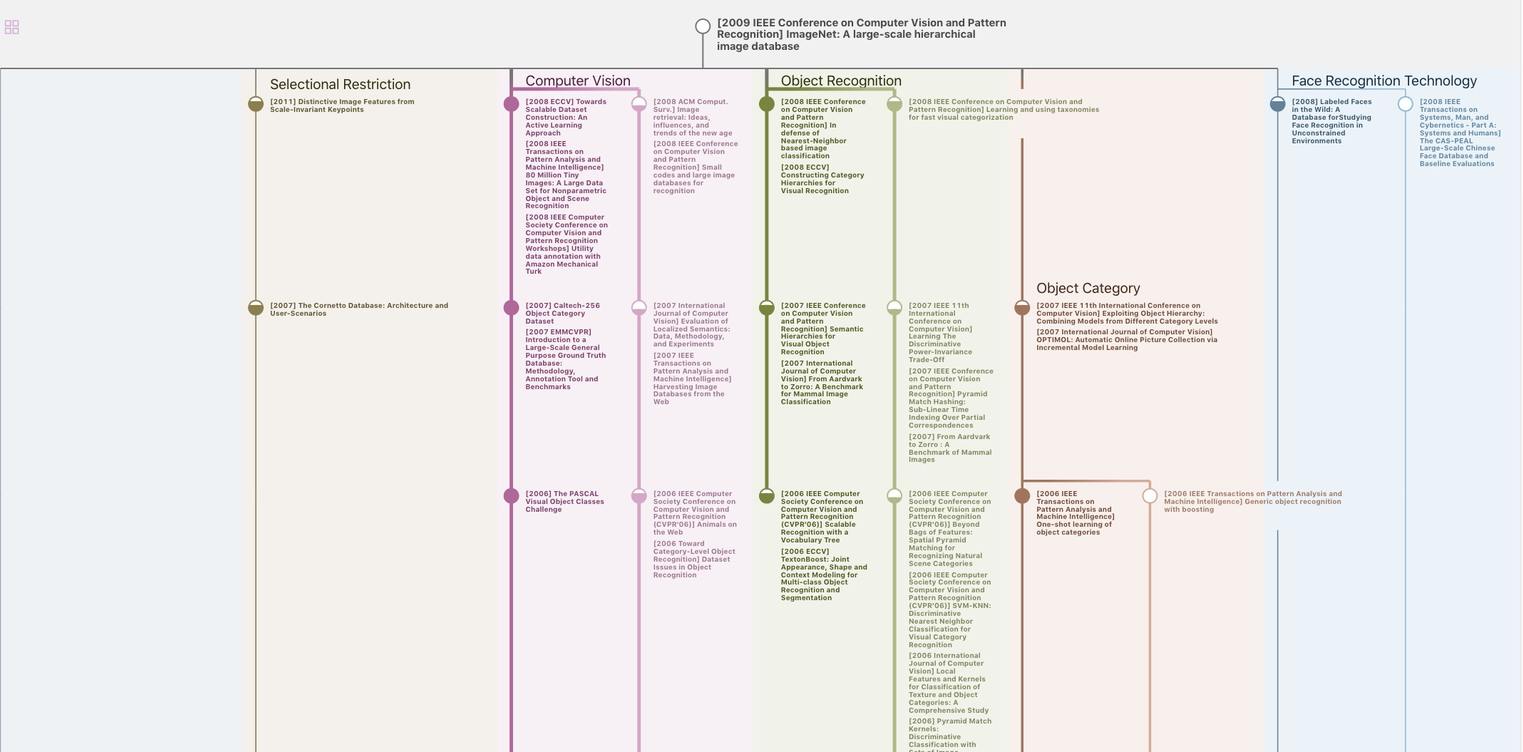
生成溯源树,研究论文发展脉络
Chat Paper
正在生成论文摘要