Hierarchical Reinforcement Learning for Scarce Medical Resource Allocation with Imperfect Information
Knowledge Discovery and Data Mining(2021)
摘要
ABSTRACTFacing the outbreak of COVID-19, shortage in medical resources becomes increasingly outstanding. Therefore, efficient strategies for medical resource allocation are urgently called for. Reinforcement learning (RL) is powerful for decision making, but three key challenges exist in solving this problem via RL: (1) complex situation and countless choices for decision making in the real world; (2) only imperfect information are available due to the latency of pandemic spreading; (3) limitations on conducting experiments in real world since we cannot set pandemic outbreaks arbitrarily. In this paper, we propose a hierarchical reinforcement learning method with a corresponding training algorithm. We design a decomposed action space to deal with the countless choices to ensure efficient and real time strategies. We also design a recurrent neural network based framework to utilize the imperfect information obtained from the environment. We build a pandemic spreading simulator based on real world data, serving as the experimental platform. We conduct extensive experiments and the results show that our method outperforms all the baselines, which reduces infections and deaths by 14.25% on average.
更多查看译文
关键词
Hierarchical reinforcement learning, medical resource allocation, imperfect information, COVID-19 pandemic
AI 理解论文
溯源树
样例
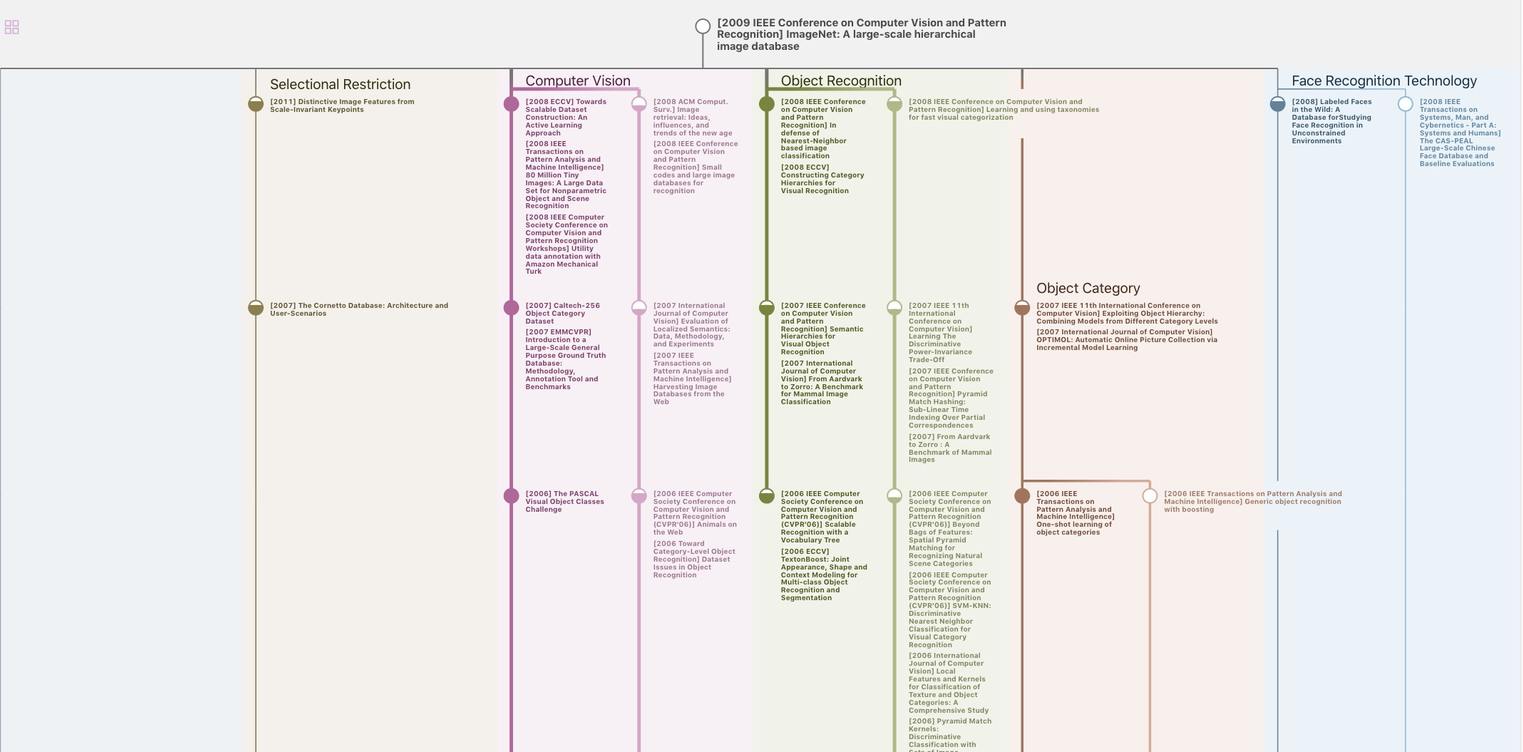
生成溯源树,研究论文发展脉络
Chat Paper
正在生成论文摘要