Contextual Bandit Applications in a Customer Support Bot
KDD 2021(2021)
摘要
Virtual support agents have grown in popularity as a way for businesses to provide better and more accessible customer service. Some challenges in this domain include ambiguous user queries as well as changing support topics and user behavior (non-stationarity). We do, however, have access to partial feedback provided by the user (clicks, surveys, and other events) which can be leveraged to improve the user experience. Adaptable learning techniques, like contextual bandits, are a natural fit for this problem setting. In this paper, we discuss real-world implementations of contextual bandits (CB) for the Microsoft virtual agent. It includes intent disambiguation based on neural-linear bandits (NLB) and contextual recommendations based on a collection of multi-armed bandits (MAB). Our solutions have been deployed to production and have improved key business metrics of the Microsoft virtual agent, as confirmed by A/B experiments. Results include a relative increase of over 12% in problem resolution rate and relative decrease of over 4% in escalations to a human operator. While our current use cases focus on intent disambiguation and contextual recommendation for support bots, we believe our methods can be extended to other domains.
更多查看译文
关键词
multi-armed bandits,contextual bandits,chat bots
AI 理解论文
溯源树
样例
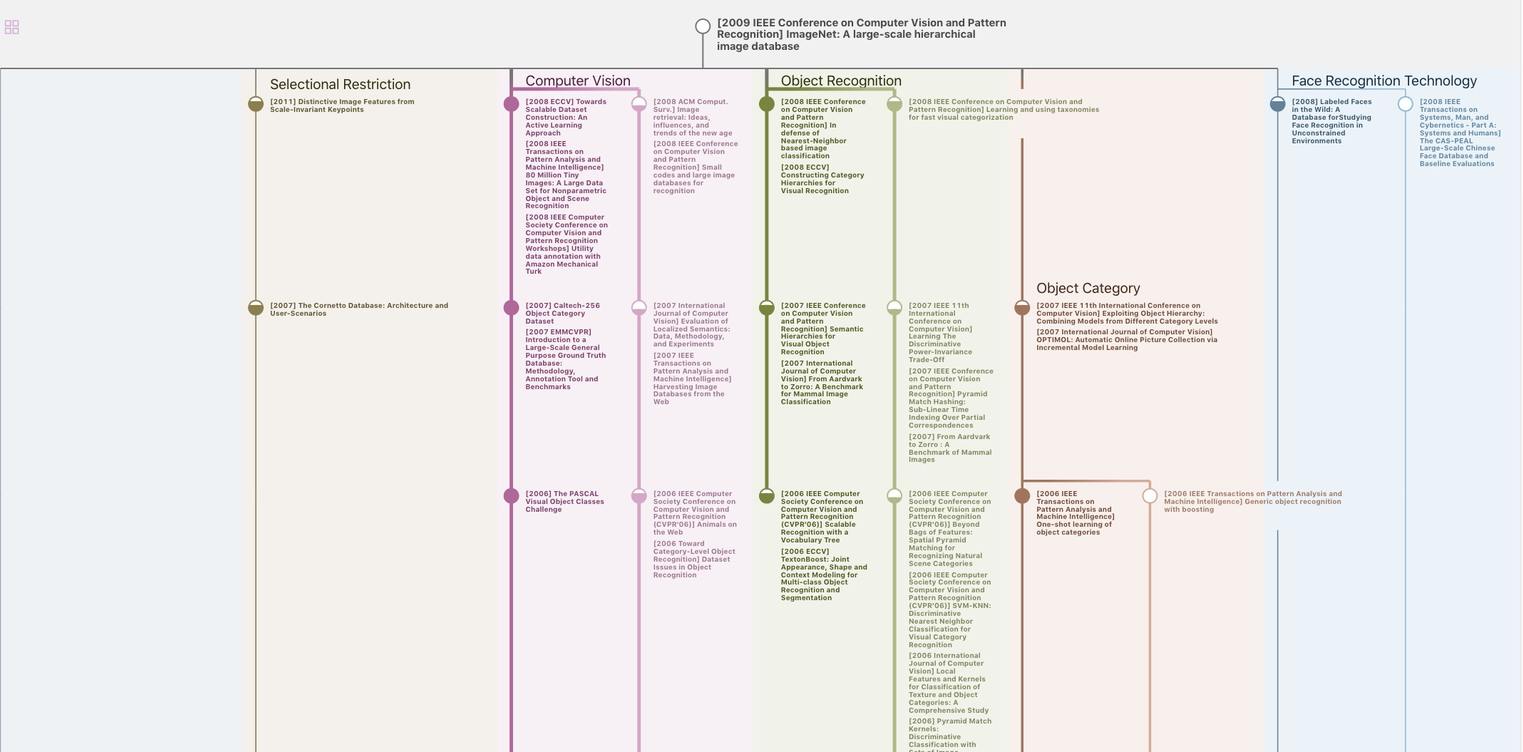
生成溯源树,研究论文发展脉络
Chat Paper
正在生成论文摘要