Reinforced Anchor Knowledge Graph Generation for News Recommendation Reasoning
Knowledge Discovery and Data Mining(2021)
摘要
ABSTRACTNews recommendation systems play a key role in online news reading service. Knowledge graphs (KG), which contain comprehensive structural knowledge, are well known for their potential to enhance both accuracy and explainability. While existing works intensively study using KG to improve news recommendation accuracy, using KG for news recommendation reasoning has not been fully explored. A few works such as KPRN [18], [22] and ADAC [25] have discussed knowledge reasoning in some other recommendation domains such as music or movie, but their methods are not practical for the news. How to make reasoning scalable to generic KGs, easy to deploy for real-time serving and meanwhile elastic for both recall and ranking stages remains an open question. In this paper, we fill the research gap by proposing a novel recommendation reasoning paradigm AnchorKG. For each article, AnchorKG generates a compact Anchor Knowledge G raph, which corresponds to a subset of entities and their k-hop neighbors in the KG, restoring the most important knowledge information of the article. On one hand, the anchor graph can be used to enhance the latent representation of the article. On the other hand, the interaction between two anchor graphs can be used for reasoning. We develop a reinforcement learning-based framework to train the anchor graph generator, in which there are three major components, including the joint learning of recommendation and reasoning, sophisticated reward signals, and a warm-up learning stage. We conduct experiments on one public dataset and one private dataset. Results demonstrate that the AnchorKG framework not only improves recommendation accuracy, but also provides high quality knowledge-aware reasoning. We release the source code at https://github.com/danyang-liu/AnchorKG .
更多查看译文
关键词
news recommender, knowledge graph, recommendation reasoning
AI 理解论文
溯源树
样例
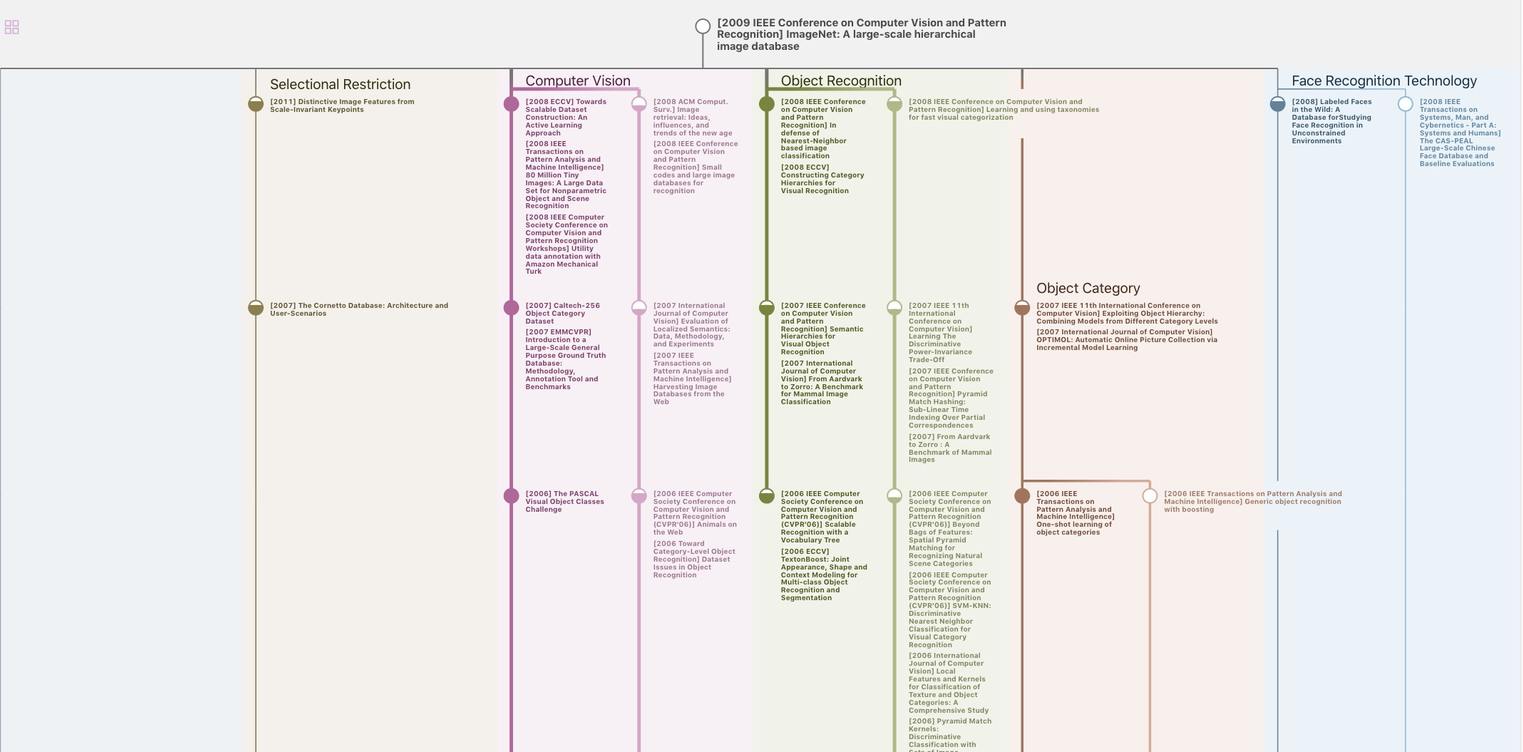
生成溯源树,研究论文发展脉络
Chat Paper
正在生成论文摘要