Preference Amplification in Recommender Systems
Knowledge Discovery and Data Mining(2021)
摘要
ABSTRACTRecommender systems have become increasingly accurate in suggesting content to users, resulting in users primarily consuming content through recommendations. This can cause the user's interest to narrow toward the recommended content, something we refer to as preference amplification. While this can contribute to increased engagement, it can also lead to negative experiences such as lack of diversity and echo chambers. We propose a theoretical framework for studying such amplification in a matrix factorization based recommender system. We model the dynamics of the system, where users interact with the recommender systems and gradually "drift'' toward the recommended content, with the recommender system adapting, based on user feedback, to the updated preferences. We study the conditions under which preference amplification manifests, and validate our results with simulations. Finally, we evaluate mitigation strategies that prevent the adverse effects of preference amplification and present experimental results using a real-world large-scale video recommender system showing that by reducing exposure to potentially objectionable content we can increase user engagement by up to 2%.
更多查看译文
关键词
Recommender systems, echo chambers, filter bubbles, fixed point
AI 理解论文
溯源树
样例
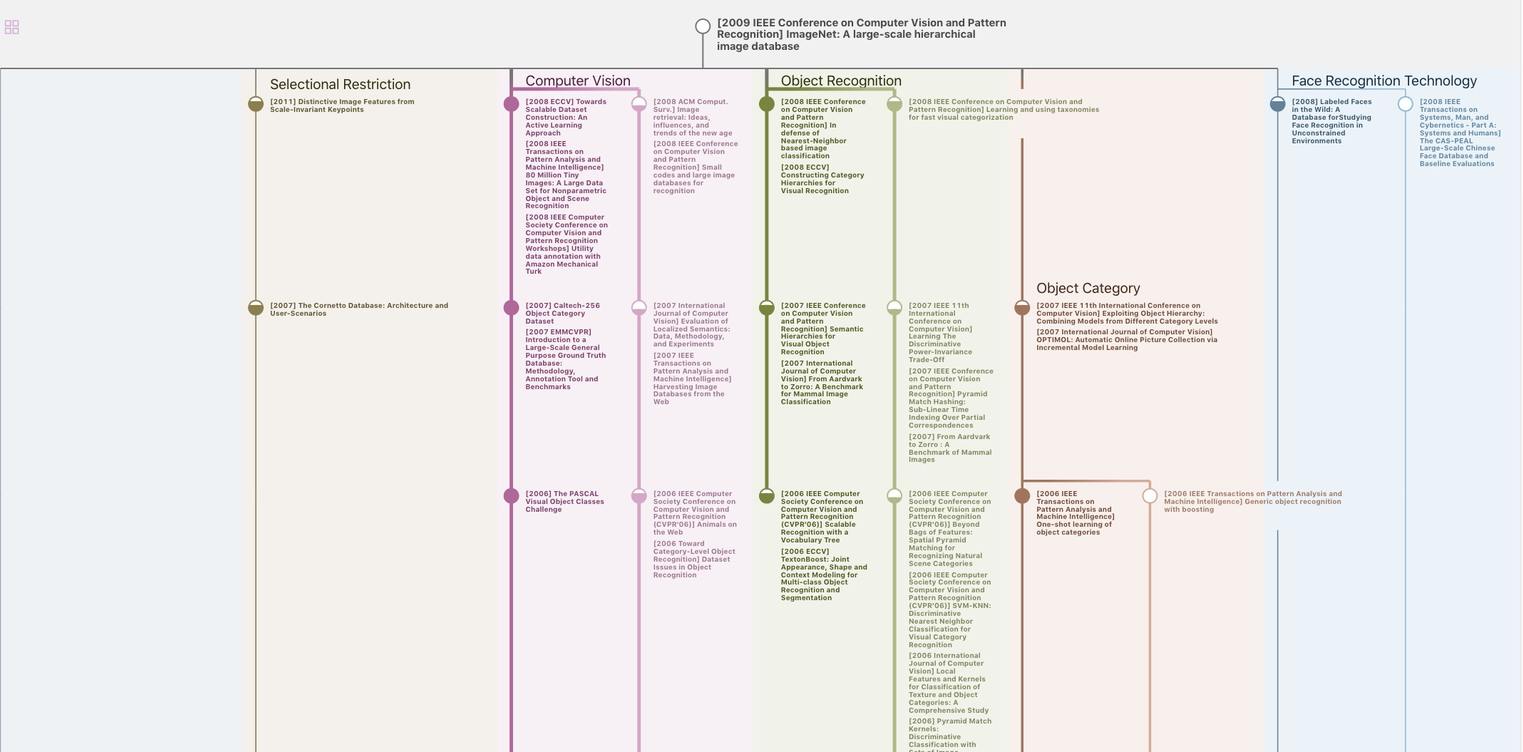
生成溯源树,研究论文发展脉络
Chat Paper
正在生成论文摘要