Direct linkage discovery with empirical linkage learning
Genetic and Evolutionary Computation Conference(2021)
摘要
ABSTRACTProblem decomposition is an important part of many state-of-the-art Evolutionary Algorithms (EAs). The quality of the decomposition may be decisive for the EA effectiveness and efficiency. Therefore, in this paper, we focus on the recent proposition of Linkage Learning based on Local Optimization (3LO). 3LO is an empirical linkage learning (ELL) technique and is proven never to report the false linkage. False linkage is one of the possible linkage defects and occurs when linkage marks two independent genes as a dependent. Although thanks to the problem decomposition quality, the use of 3LO may lead to excellent results, its main disadvantage is its high computational cost. This disadvantage makes 3LO not applicable to state-of-the-art EAs that originally employed Statistical-based Linkage Learning (SLL) and frequently update the linkage information. Therefore, we propose the Direct Linkage Empirical Discovery technique (DLED) that preserves 3LO advantages, reduces its costs, and we prove that it is precise in recognizing the direct linkage. The concept of direct linkage, which we identify in this paper, is related to the quality of the decomposition of overlapping problems. The results show that incorporating DLED in three significantly different state-of-the-art EAs may lead to promising results.
更多查看译文
关键词
Genetic Algorithms, Linkage Learning, Model Building, Empirical linkage learning
AI 理解论文
溯源树
样例
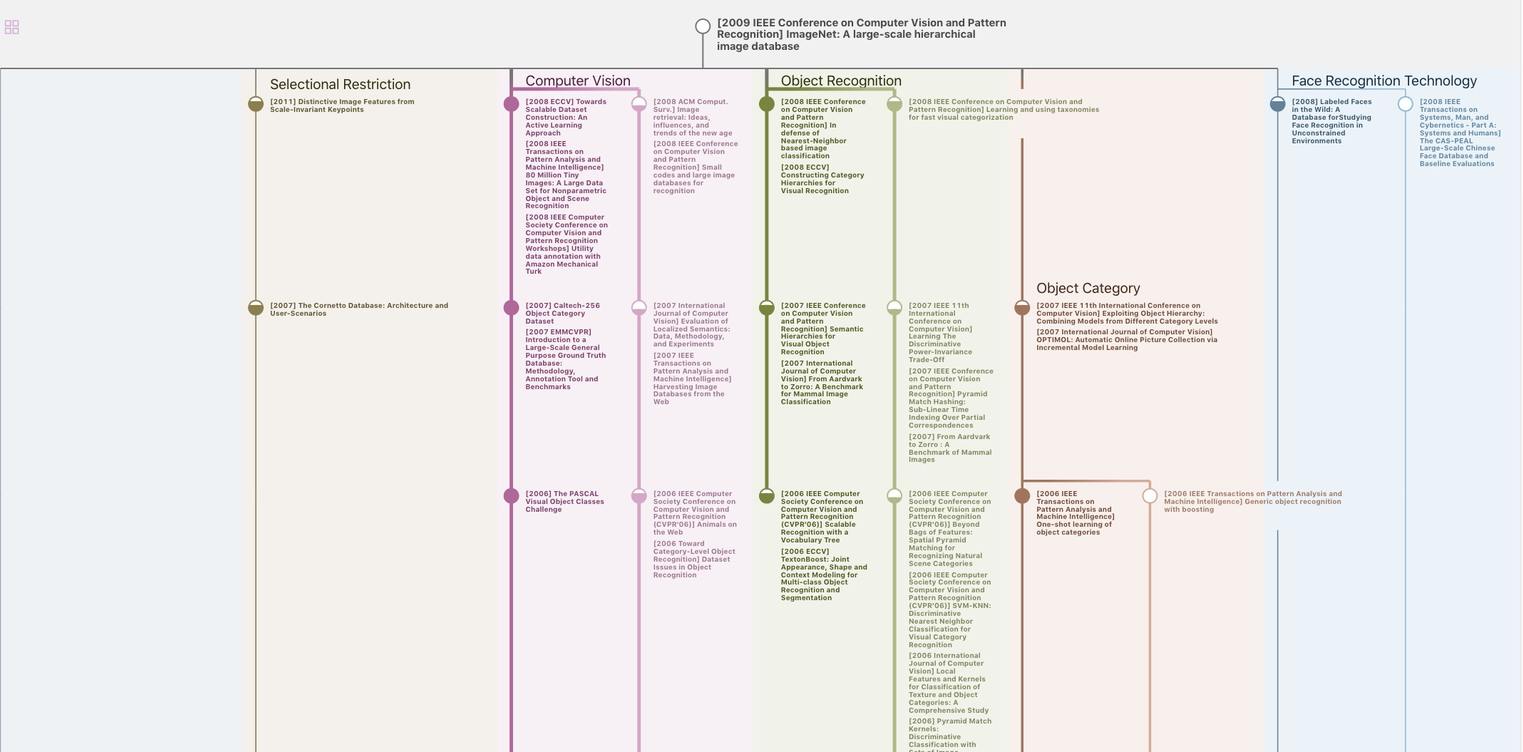
生成溯源树,研究论文发展脉络
Chat Paper
正在生成论文摘要