Multilingual Transfer of Acoustic Word Embeddings Improves When Training on Languages Related to the Target Zero-Resource Language.
Interspeech(2021)
摘要
Acoustic word embedding models map variable duration speech segments to fixed dimensional vectors, enabling efficient speech search and discovery. Previous work explored how embeddings can be obtained in zero-resource settings where no labelled data is available in the target language. The current best approach uses transfer learning: a single supervised multilingual model is trained using labelled data from multiple well-resourced languages and then applied to a target zero-resource language (without fine-tuning). However, it is still unclear how the specific choice of training languages affect downstream performance. Concretely, here we ask whether it is beneficial to use training languages related to the target. Using data from eleven languages spoken in Southern Africa, we experiment with adding data from different language families while controlling for the amount of data per language. In word discrimination and query-by-example search evaluations, we show that training on languages from the same family gives large improvements. Through finer-grained analysis, we show that training on even just a single related language gives the largest gain. We also find that adding data from unrelated languages generally doesn't hurt performance.
更多查看译文
关键词
acoustic word embeddings,zero-resource speech processing,transfer learning,languages of Southern Africa
AI 理解论文
溯源树
样例
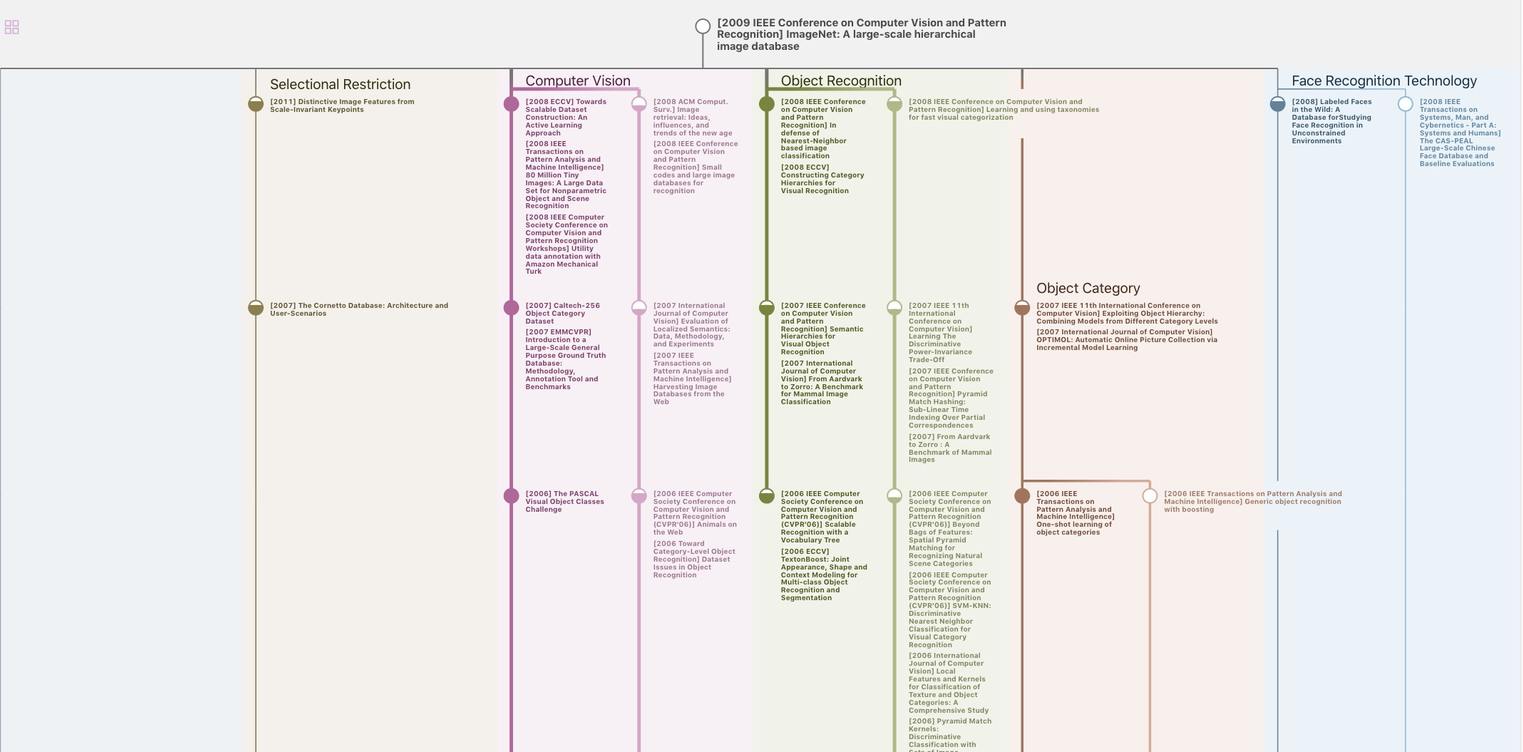
生成溯源树,研究论文发展脉络
Chat Paper
正在生成论文摘要