Robustness Testing of AI Systems - A Case Study for Traffic Sign Recognition.
AIAI(2021)
Abstract
In the last years, AI systems, in particular neural networks, have seen a tremendous increase in performance, and they are now used in a broad range of applications. Unlike classical symbolic AI systems, neural networks are trained using large data sets and their inner structure containing possibly billions of parameters does not lend itself to human interpretation. As a consequence, it is so far not feasible to provide broad guarantees for the correct behaviour of neural networks during operation if they process input data that significantly differ from those seen during training. However, many applications of AI systems are security- or safety-critical, and hence require obtaining statements on the robustness of the systems when facing unexpected events, whether they occur naturally or are induced by an attacker in a targeted way. As a step towards developing robust AI systems for such applications, this paper presents how the robustness of AI systems can be practically examined and which methods and metrics can be used to do so. The robustness testing methodology is described and analysed for the example use case of traffic sign recognition in autonomous driving.
MoreTranslated text
Key words
traffic sign recognition,ai systems,robustness
AI Read Science
Must-Reading Tree
Example
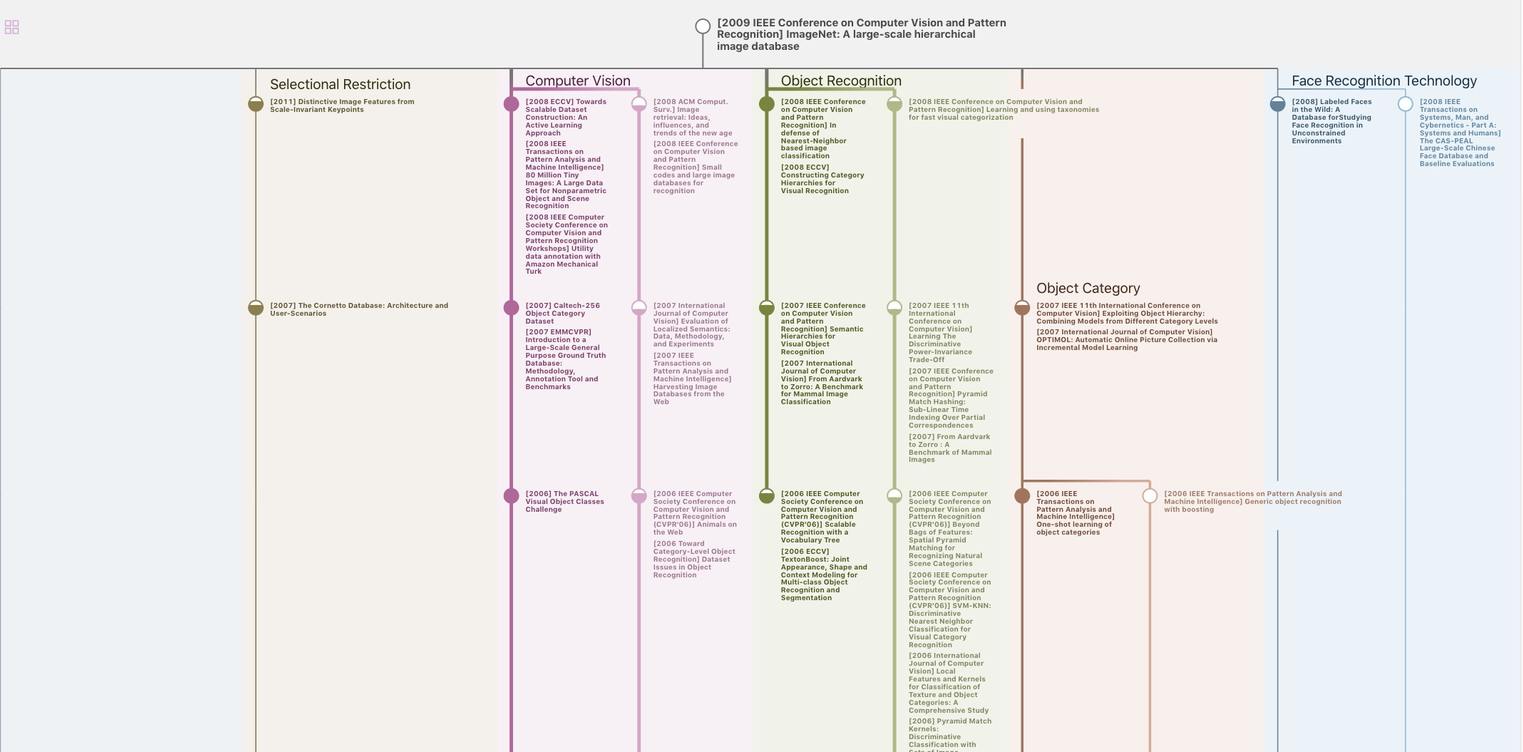
Generate MRT to find the research sequence of this paper
Chat Paper
Summary is being generated by the instructions you defined