Regret-Optimal Estimation and Control
IEEE TRANSACTIONS ON AUTOMATIC CONTROL(2023)
Abstract
In this article, we consider estimation and control in linear dynamical systems from the perspective of regret minimization. Unlike most prior work in this area, we focus on the problem of designing causal state estimators and causal controllers, which compete against a clairvoyant noncausal policy, instead of the best policy selected in hindsight from some fixed parametric class. We show that regret-optimal filters and regret-optimal controllers can be derived in state space form using operator-theoretic techniques from robust control. Our results can be viewed as extending traditional robust estimation and control, which focuses on minimizing worst-case cost, to minimizing worst-case regret. We propose regret-optimal analogs of model-predictive control and the extended Kalman filter for systems with nonlinear dynamics and present numerical experiments which show that these algorithms can significantly outperform standard approaches to estimation and control.
MoreTranslated text
Key words
Estimation,Costs,Heuristic algorithms,Minimization,Standards,Benchmark testing,Prediction algorithms,Filtering,machine learning,robust control
AI Read Science
Must-Reading Tree
Example
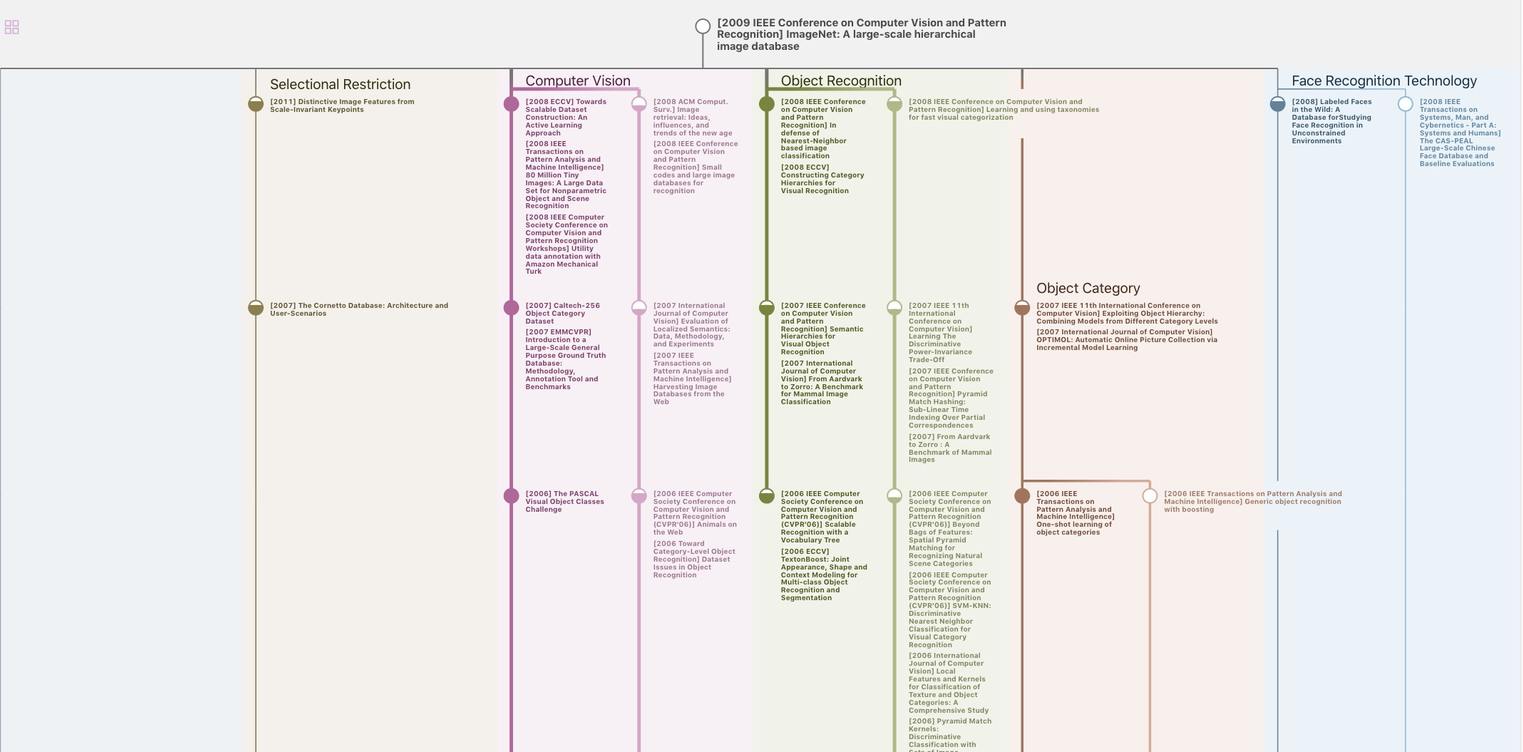
Generate MRT to find the research sequence of this paper
Chat Paper
Summary is being generated by the instructions you defined