Leveraging Machine Learning Approaches To Estimate The Impact Of Thermostat Setpoints On Individual Household Gas Consumption
2021 22ND IEEE INTERNATIONAL CONFERENCE ON INDUSTRIAL TECHNOLOGY (ICIT)(2021)
摘要
Given the world's current climate change challenge and residential gas consumption being a major end-use of energy, people more than ever need to minimize their household's energy footprint. Personalised, actionable advice can give people tips on which actions they can take to reduce residential energy usage, such as lowering the thermostat temperature. For this advice to be relevant it is important to understand the quantitative impact of thermostat setpoints on daily gas usage for each individual household. In this article, this impact is estimated by comparing three machine learning approaches.Linear regression, deep learning and gradient boosting machine are applied to a multi-dimensional time series dataset for 300 Dutch households. The three approaches are compared based on three metrics: root mean square error (RMSE), explainability and scalability. The results of the best model (gradient boosting machine) are explained using a technique called SHapley Additive exPlanations (SHAP). This interpretation method can quantify the contribution of all inputs, among which thermostat setpoints, to the daily gas usage prediction of the model for different individual households.This article adds to the current state of the art by focusing on the impact of influenceable thermostat setpoints, as opposed to less actionable factors such as house size, insulation status of the house and weather. By applying SHAP, the personal impact and differences between individual households are estimated, in contrast to only learning trends. Moreover, a machine learning model, trained on a representative dataset, is applicable at scale to other households for estimating a personal, quantified impact of setpoint choices.
更多查看译文
关键词
energy conservation, heating, thermostats, buildings, machine learning algorithms, big data applications
AI 理解论文
溯源树
样例
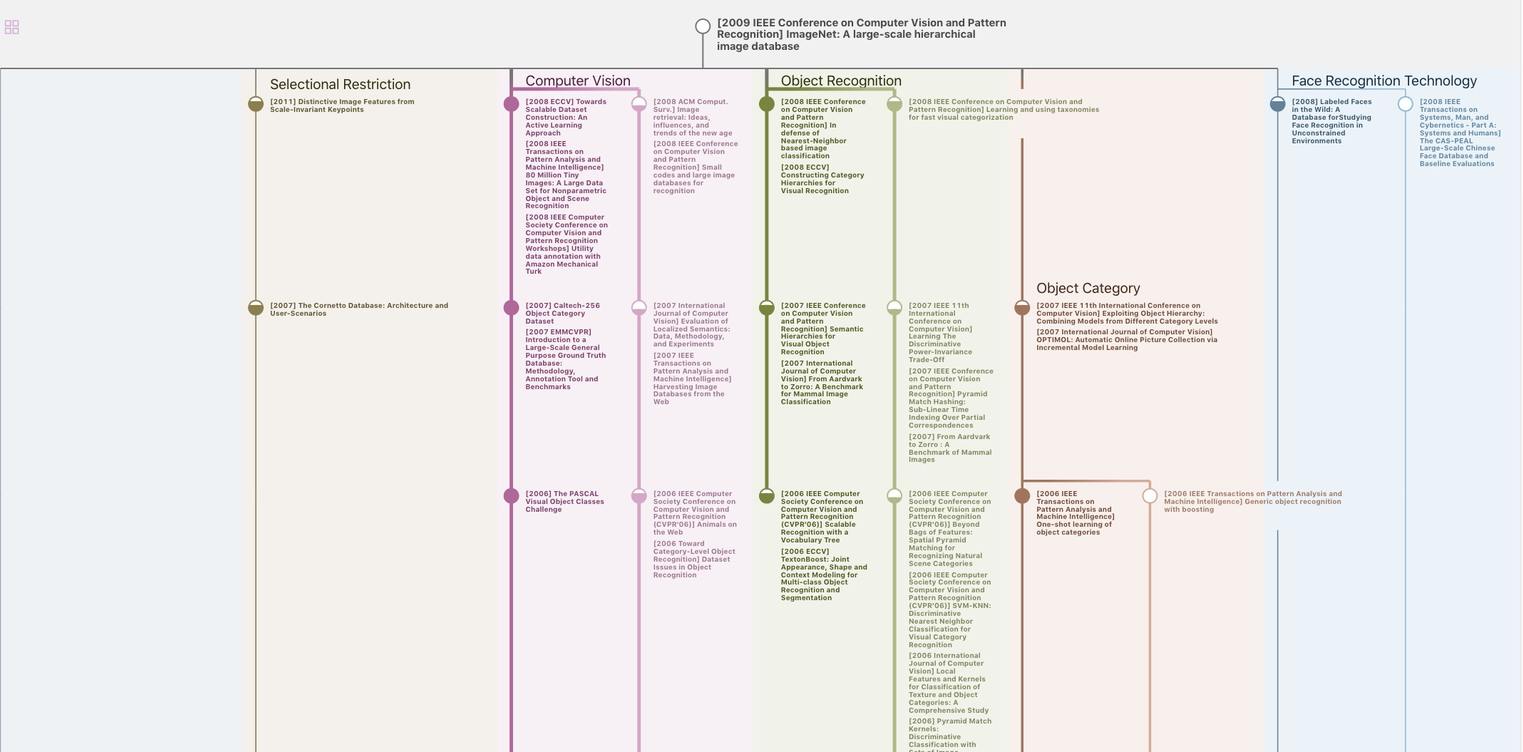
生成溯源树,研究论文发展脉络
Chat Paper
正在生成论文摘要