Enabling hyper-differential sensitivity analysis for ill-posed inverse problems
arxiv(2022)
摘要
Inverse problems constrained by partial differential equations (PDEs) play a critical role in model development and calibration. In many applications, there are multiple uncertain parameters in a model that must be estimated. However, high dimensionality of the parameters and computational complexity of the PDE solves make such problems challenging. A common approach is to reduce the dimension by fixing some parameters (which we will call auxiliary parameters) to a best estimate and use techniques from PDE-constrained optimization to estimate the other parameters. In this article, hyper-differential sensitivity analysis (HDSA) is used to assess the sensitivity of the solution of the PDE-constrained optimization problem to changes in the auxiliary parameters. Foundational assumptions for HDSA require satisfaction of the optimality conditions which are not always practically feasible as a result of ill-posedness in the inverse problem. We introduce novel theoretical and computational approaches to justify and enable HDSA for ill-posed inverse problems by projecting the sensitivities on likelihood informed subspaces and defining a posteriori updates. Our proposed framework is demonstrated on a nonlinear multi-physics inverse problem motivated by estimation of spatially heterogenous material properties in the presence of spatially distributed parametric modeling uncertainties.
更多查看译文
关键词
sensitivity,inverse
AI 理解论文
溯源树
样例
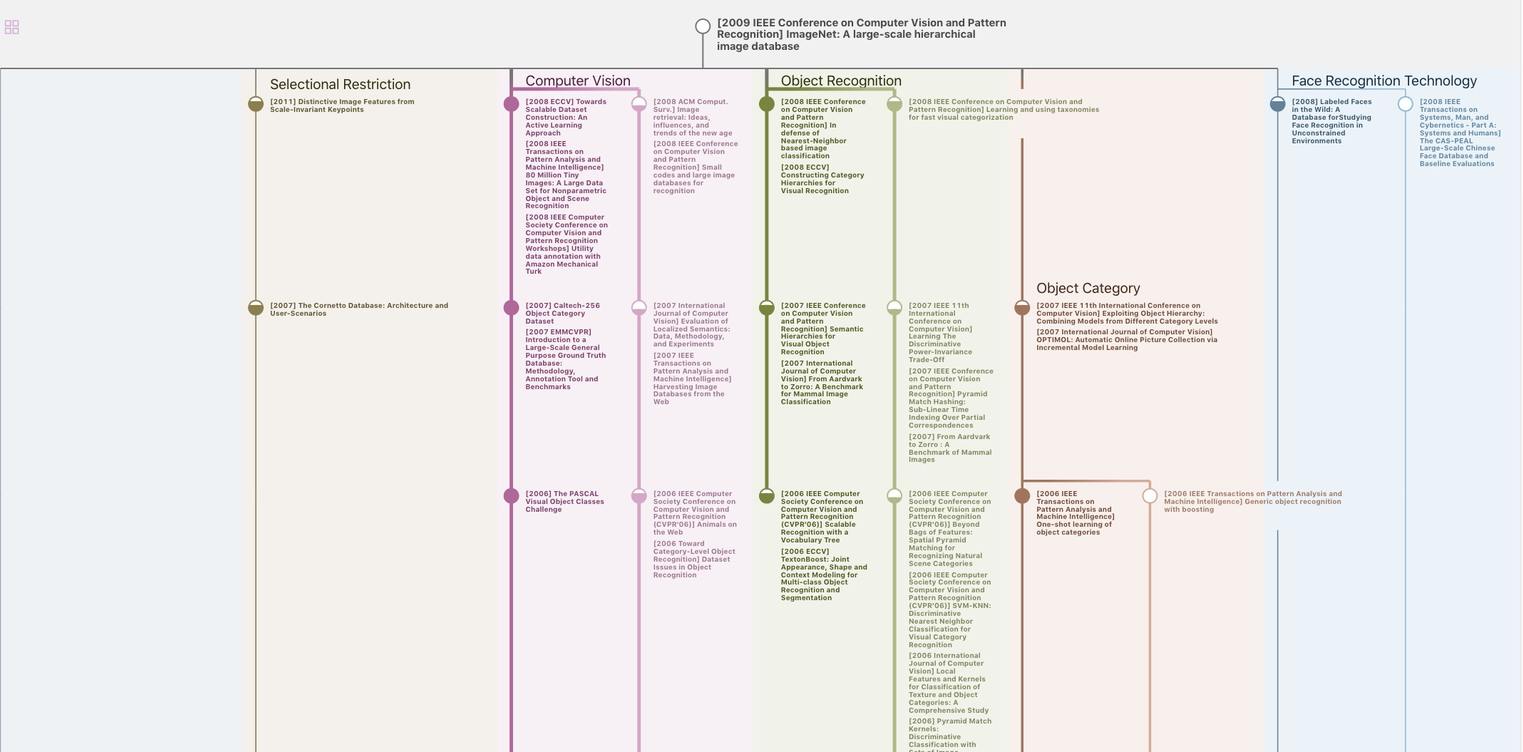
生成溯源树,研究论文发展脉络
Chat Paper
正在生成论文摘要