FDeblur-GAN: Fingerprint Deblurring using Generative Adversarial Network
2021 IEEE International Joint Conference on Biometrics (IJCB)(2021)
Abstract
While working with fingerprint images acquired from crime scenes, mobile cameras, or low-quality sensors, it becomes difficult for automated identification systems to verify the identity due to image blur and distortion. We propose a fingerprint deblurring model FDeblur-GAN, based on the conditional Generative Adversarial Networks (cGANs) and multi-stage framework of the stack GAN. Additionally, we integrate two auxiliary sub-networks into the model for the deblurring task. The first sub-network is a ridge extractor model. It is added to generate ridge maps to ensure that fingerprint information and minutiae are preserved in the deblurring process and prevent the model from generating erroneous minutiae. The second sub-network is a verifier that helps the generator to preserve the ID information during the generation process. Using a database of blurred fingerprints and corresponding ridge maps, the deep network learns to deblur from the input blurry samples. We evaluate the proposed method in combination with two different fingerprint matching algorithms. We achieved an accuracy of 95.18% on our fingerprint database for the task of matching deblurred and ground truth fingerprints.
MoreTranslated text
Key words
image blur,image distortion,stack GAN,input blurry samples,cGANs,multistage framework,FDeblur-GAN,fingerprint deblurring model,automated identification systems,low-quality sensors,mobile cameras,crime scenes,fingerprint images,conditional generative adversarial network,ground truth fingerprints,deblurred truth fingerprints,fingerprint database,fingerprint matching algorithms,deep network,ridge maps,generation process,deblurring process,minutiae,fingerprint information,ridge extractor model,auxiliary subnetworks
AI Read Science
Must-Reading Tree
Example
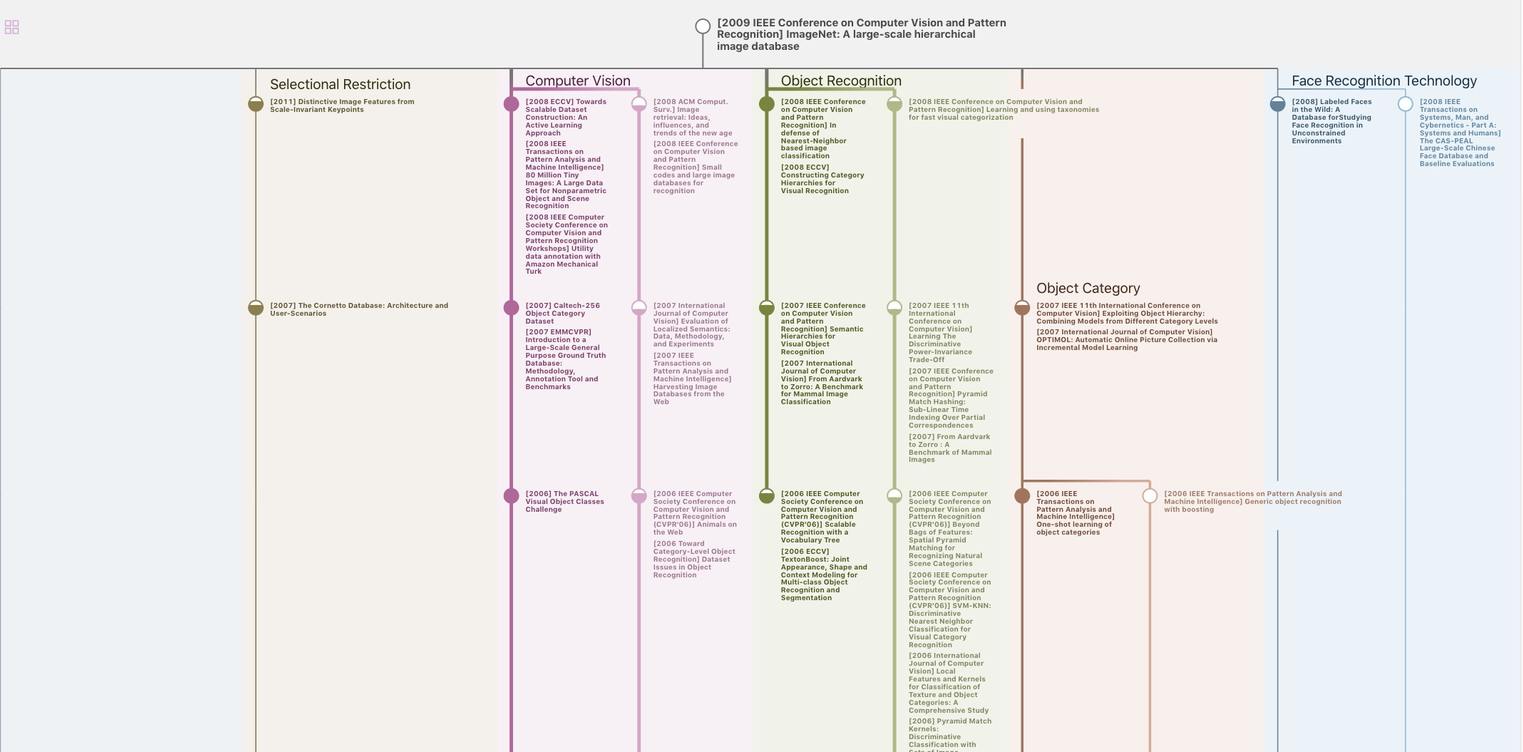
Generate MRT to find the research sequence of this paper
Chat Paper
Summary is being generated by the instructions you defined