Estimating MRI Image Quality via Image Reconstruction Uncertainty
arxiv(2021)
摘要
Quality control (QC) in medical image analysis is time-consuming and laborious, leading to increased interest in automated methods. However, what is deemed suitable quality for algorithmic processing may be different from human-perceived measures of visual quality. In this work, we pose MR image quality assessment from an image reconstruction perspective. We train Bayesian CNNs using a heteroscedastic uncertainty model to recover clean images from noisy data, providing measures of uncertainty over the predictions. This framework enables us to divide data corruption into learnable and non-learnable components and leads us to interpret the predictive uncertainty as an estimation of the achievable recovery of an image. Thus, we argue that quality control for visual assessment cannot be equated to quality control for algorithmic processing. We validate this statement in a multi-task experiment combining artefact recovery with uncertainty prediction and grey matter segmentation. Recognising this distinction between visual and algorithmic quality has the impact that, depending on the downstream task, less data can be excluded based on ``visual quality" reasons alone.
更多查看译文
关键词
mri image quality,image reconstruction uncertainty
AI 理解论文
溯源树
样例
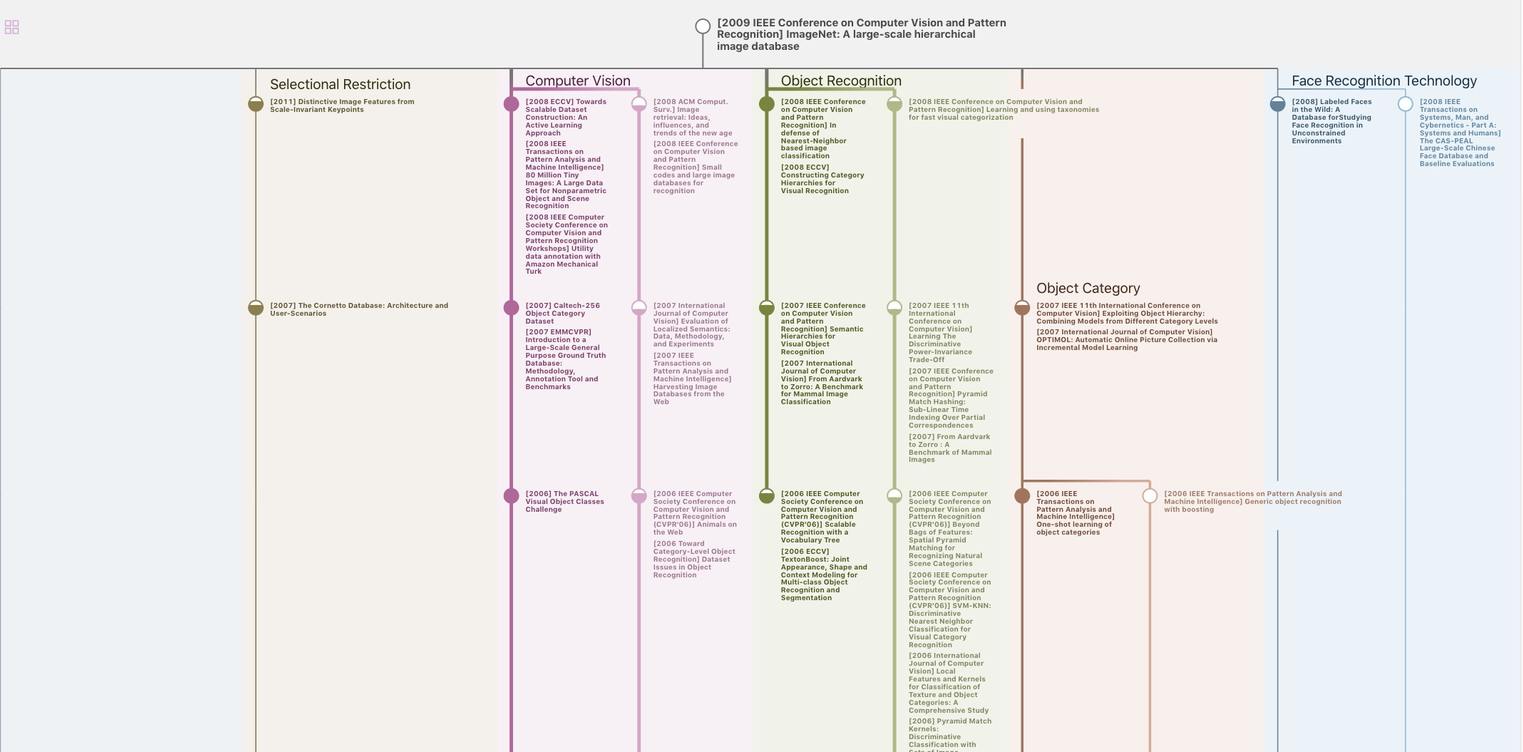
生成溯源树,研究论文发展脉络
Chat Paper
正在生成论文摘要