FedCM: Federated Learning with Client-level Momentum
arxiv(2021)
摘要
Federated Learning is a distributed machine learning approach which enables model training without data sharing. In this paper, we propose a new federated learning algorithm, Federated Averaging with Client-level Momentum (FedCM), to tackle problems of partial participation and client heterogeneity in real-world federated learning applications. FedCM aggregates global gradient information in previous communication rounds and modifies client gradient descent with a momentum-like term, which can effectively correct the bias and improve the stability of local SGD. We provide theoretical analysis to highlight the benefits of FedCM. We also perform extensive empirical studies and demonstrate that FedCM achieves superior performance in various tasks and is robust to different levels of client numbers, participation rate and client heterogeneity.
更多查看译文
关键词
federated learning,client-level
AI 理解论文
溯源树
样例
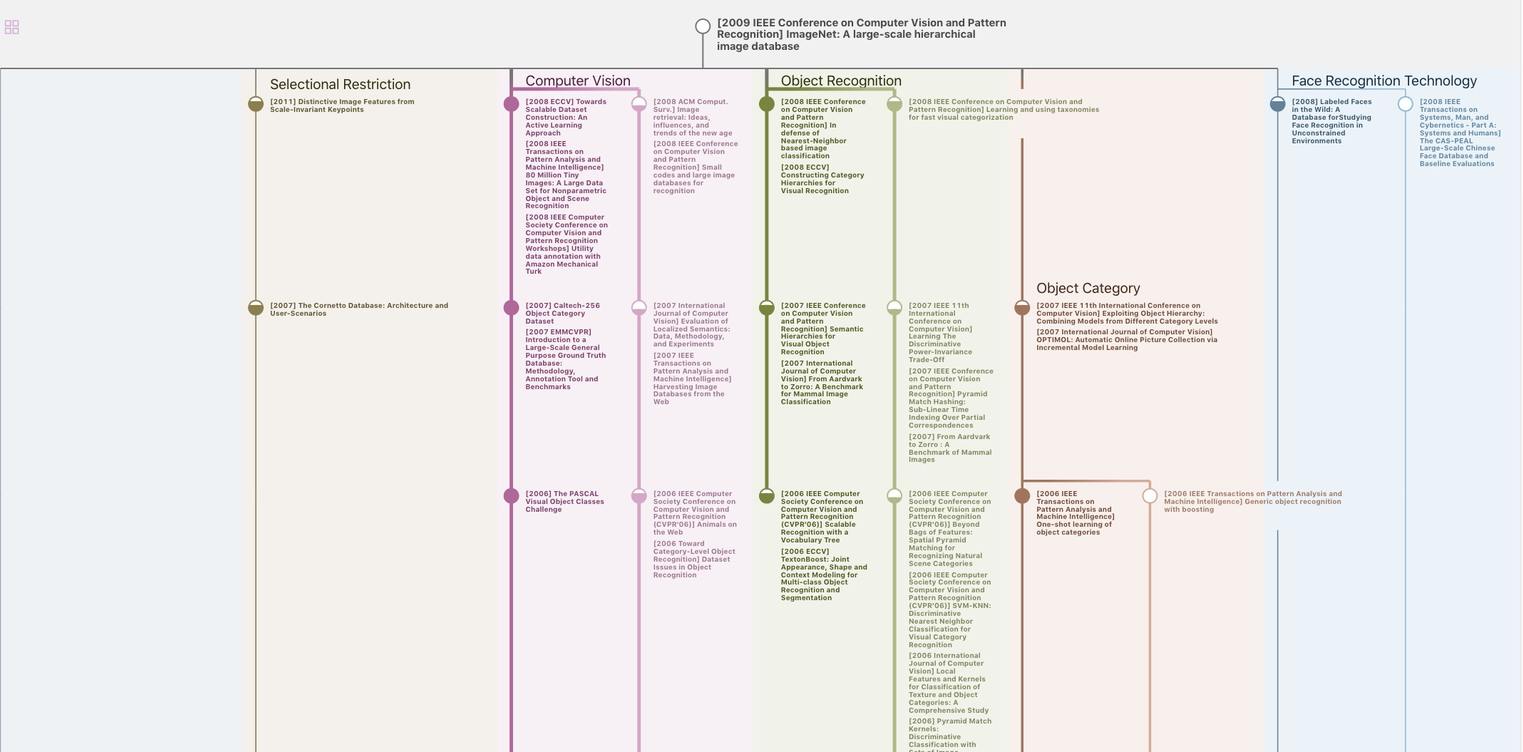
生成溯源树,研究论文发展脉络
Chat Paper
正在生成论文摘要