Quantum convolutional neural network for image classification
2020 8th International Conference on Digital Home (ICDH)(2022)
摘要
In this paper we propose two scale-inspired local feature extraction methods based on Quantum Convolutional Neural Network (QCNN) in the Tensorflow quantum framework for binary image classification. The image data is properly downscaled with Multi-scale Entanglement Renormalization Ansatz and Box-counting based fractal features before fed into the QCNN’s quantum circuits for state preparation, quantum convolution and quantum pooling. Quantum classifiers with one QCNN and two hybrid Quantum-classical QCNN models have been trained with a breast cancer dataset, and their performance are compared against that of a classic CNN. The results show that the proposed QCNN with the proposed feature extraction methods outperformed the classic CNN in terms of recognition accuracy. It is interesting to find that image bit-plane slicing has a similar internal mechanism to that of the Ising phase transition. This observation motivates us to explore the correlation between the chaotic nature of image and the classification performance enhancement by QCNN classifiers. It also implies that the pixels of the image and the Ising chaology particles share some similar patterns and are apt to classification.
更多查看译文
关键词
Quantum convolutional neural network,MERA circuit,Image classification,Box-counting,Tensorflow quantum
AI 理解论文
溯源树
样例
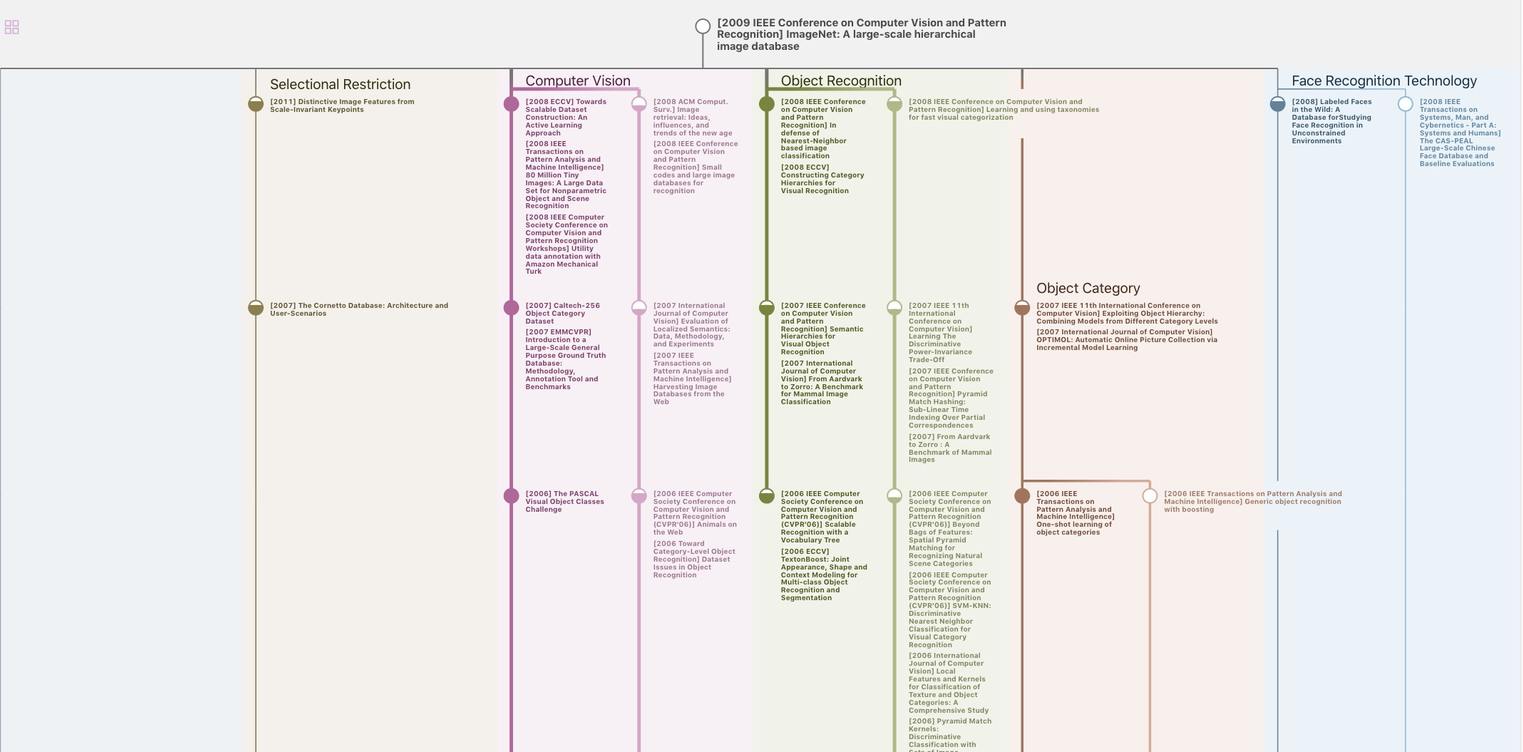
生成溯源树,研究论文发展脉络
Chat Paper
正在生成论文摘要