Tolerating Stuck-at Fault and Variation in Resistive Edge Inference Engine via Weight Mapping
GLSVLSI(2021)
摘要
ABSTRACTThere is an increasing demand for running neural network inference on edge devices. Memristor crossbar array (MCA) based accelerators can be used to accelerate neural networks on edge devices. However, reliability issues in memristors, such as stuck-at faults (SAF) and variations, lead to weight deviation of neural networks and therefore have severe influence on inference accuracy. In this work, we focus on reliability issues for edge devices. We formulate the reliability problem as a 0-1 programming problem, based on the analysis of sum weight variation (SWV). In order to solve the problem, we simplify the problem with an approximation - different columns have the same weights - based on our observation of the weight distribution. Then we propose an effective mapping method to solve the simplified problem. The experimental results show that our proposed method can recover 95% accuracy considering SAF defects and can increase by up to 60% accuracy in variation σ=0.4.
更多查看译文
AI 理解论文
溯源树
样例
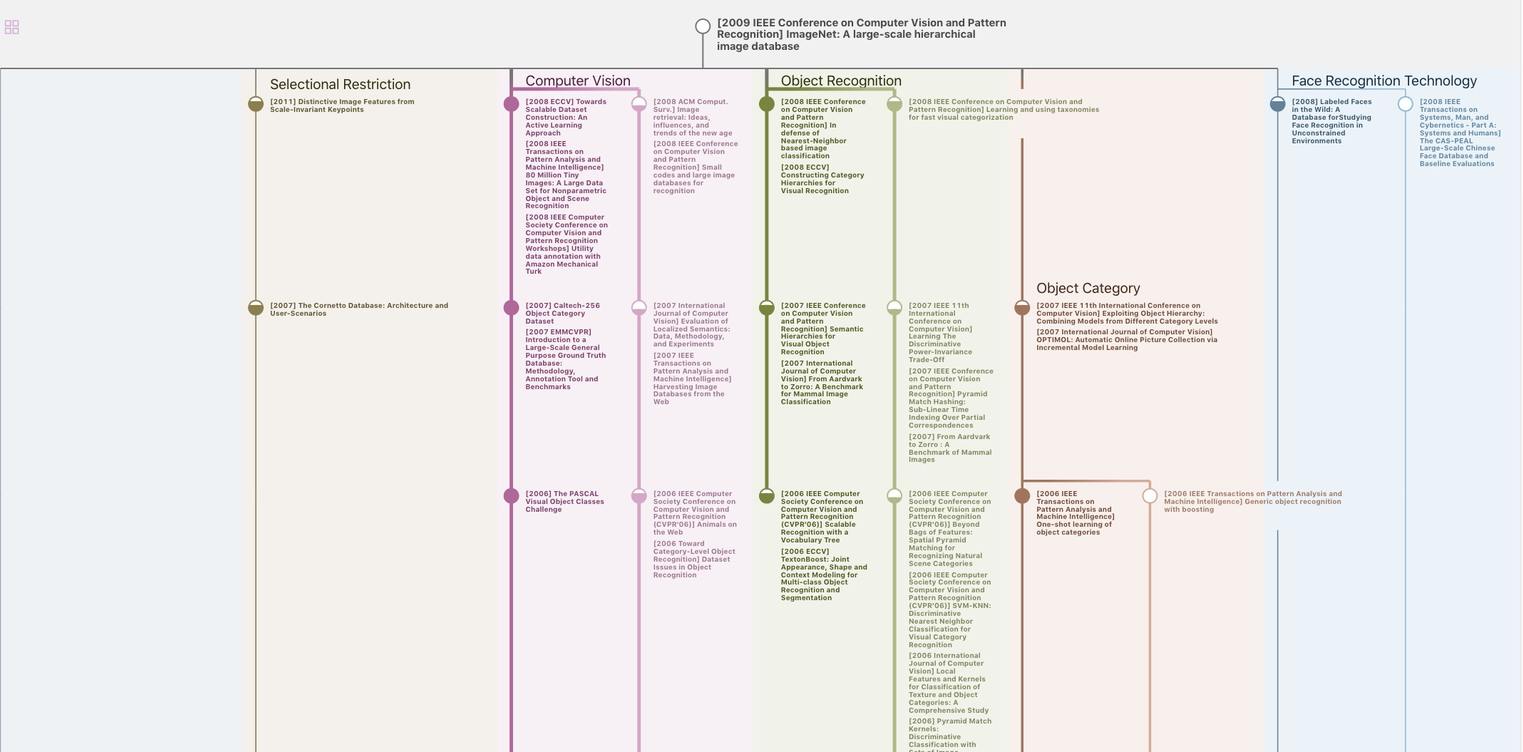
生成溯源树,研究论文发展脉络
Chat Paper
正在生成论文摘要