Jigsaw: A Data Storage and Query Processing Engine for Irregular Table Partitioning
International Conference on Management of Data(2021)
摘要
ABSTRACTThe physical data layout significantly impacts performance when database systems access cold data. In addition to the traditional row store and column store designs, recent research proposes to partition tables hierarchically, starting from either horizontal or vertical partitions and then determining the best partitioning strategy on the other dimension independently for each partition. All these partitioning strategies naturally produce rectangular partitions. Coarse-grained rectangular partitioning reads unnecessary data when a table cannot be partitioned along one dimension for all queries. Fine-grained rectangular partitioning produces many small partitions which negatively impacts I/O performance and possibly introduces a high tuple reconstruction overhead. This paper introduces Jigsaw, a system that employs a novel partitioning strategy that creates partitions with arbitrary shapes, which we refer to as irregular partitions. The traditional tuple-at-a-time or operator-at-a-time query processing models cannot fully leverage the advantages of irregular partitioning, because they may repeatedly read a partition due to its irregular shape. Jigsaw introduces a partition-at-a-time evaluation strategy to avoid repeated accesses to an irregular partition. We implement and evaluate Jigsaw on the HAP and TPC-H benchmarks and find that irregular partitioning is up to 4.2×faster than a columnar layout for moderately selective queries. Compared with the columnar layout, irregular partitioning only transfers 21% of the data to complete the same query.
更多查看译文
关键词
Irregular partitioning
AI 理解论文
溯源树
样例
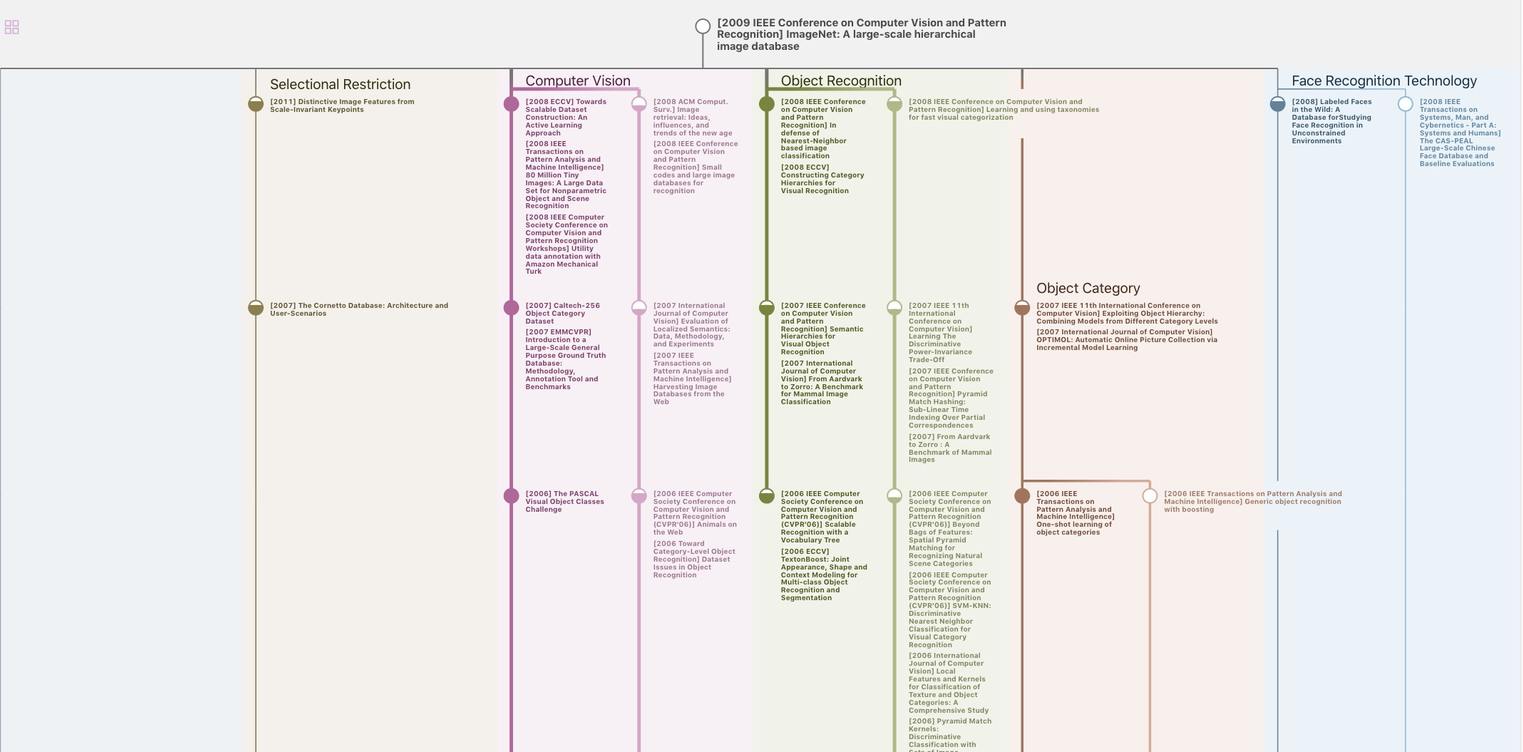
生成溯源树,研究论文发展脉络
Chat Paper
正在生成论文摘要