Zero-Shot Recognition Via Optimal Transport
2021 IEEE WINTER CONFERENCE ON APPLICATIONS OF COMPUTER VISION WACV 2021(2021)
摘要
We propose an optimal transport (OT) framework for generalized zero-shot learning (GZSL), seeking to distinguish samples for both seen and unseen classes, with the assist of auxiliary attributes. The discrepancy between features and attributes is minimized by solving an optimal transport problem. Specifically, we build a conditional generative model to generate features from seen-class attributes, and establish an optimal transport between the distribution of the generated features and that of the real features. The generative model and the optimal transport are optimized iteratively with an attribute-based regularizer, that further enhances the discriminative power of the generated features. A classifier is learned based on the features generated for both the seen and unseen classes. In addition to generalized zero-shot learning, our framework is also applicable to standard and transductive ZSL problems. Experiments show that our optimal transport-based method outperforms state-of-the-art methods on several benchmark datasets.
更多查看译文
关键词
zero-shot recognition,generalized zero-shot learning,auxiliary attributes,optimal transport problem,conditional generative model,seen-class attributes,attribute-based regularizer,optimal transport-based method,GZSL,transductive ZSL problems
AI 理解论文
溯源树
样例
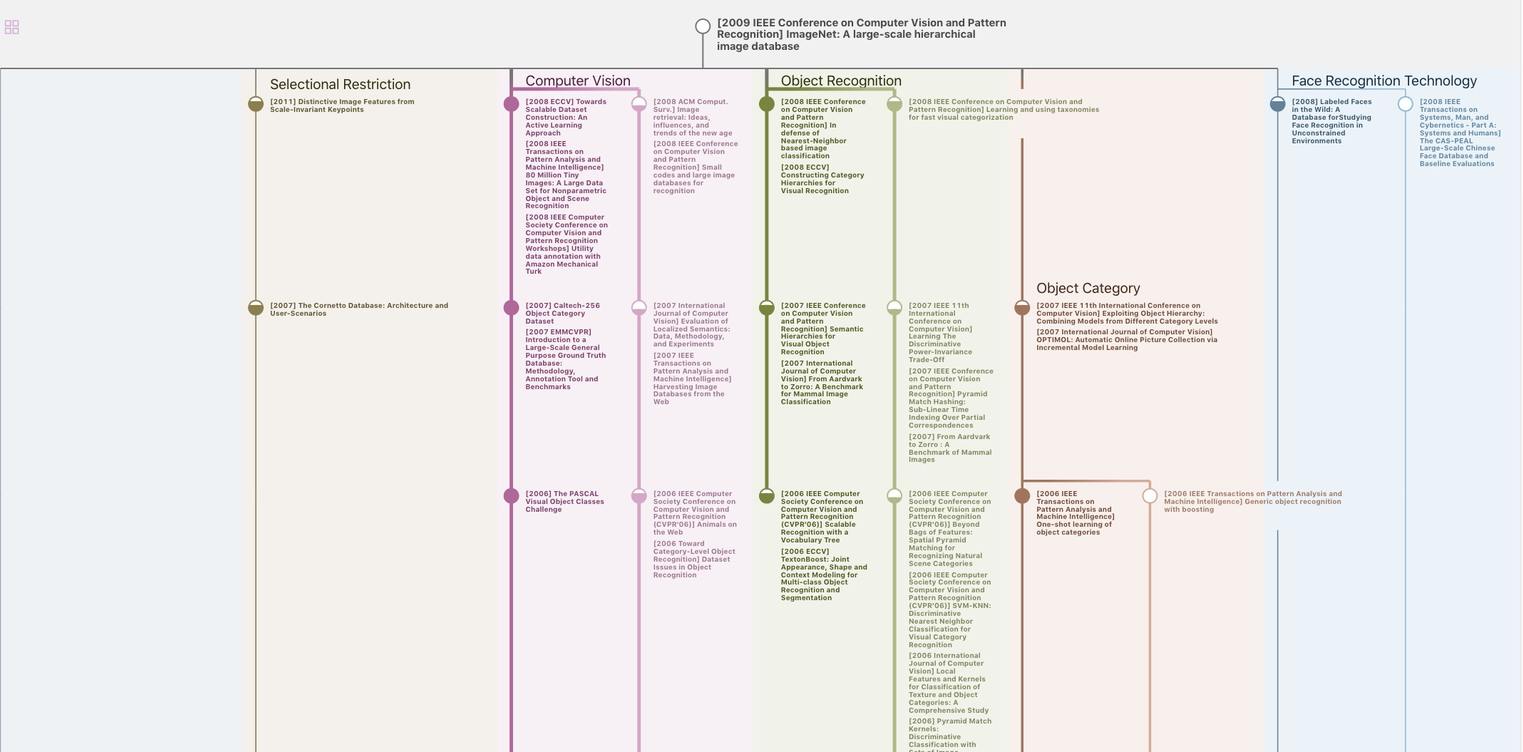
生成溯源树,研究论文发展脉络
Chat Paper
正在生成论文摘要