Dualsr: Zero-Shot Dual Learning For Real-World Super-Resolution
2021 IEEE WINTER CONFERENCE ON APPLICATIONS OF COMPUTER VISION (WACV 2021)(2021)
摘要
Advanced methods for single image super-resolution (SISR) based upon Deep learning have demonstrated a remarkable reconstruction performance on downscaled images. However, for real-world low-resolution images (e.g. images captured straight from the camera) they often generate blurry images and highlight unpleasant artifacts. The main reason is the training data that does not reflect the real-world super-resolution problem. They train the network using images downsampled with an ideal (usually bicubic) kernel. However, for real-world images the degradation process is more complex and can vary from image to image. This paper proposes a new dual-path architecture (DualSR) that learns an image-specific low-to-high resolution mapping using only patches of the input test image. For every image, a downsampler learns the degradation process using a generative adversarial network, and an upsampler learns to super-resolve that specific image. In the DualSR architecture, the upsampler and downsampler are trained simultaneously and they improve each other using cycle consistency losses. For better visual quality and eliminating undesired artifacts, the upsampler is constrained by a masked interpolation loss. On standard benchmarks with unknown degradation kernels, DualSR outperforms recent blind and non-blind super-resolution methods in term of SSIM and generates images with higher perceptual quality. On real-world LR images it generates visually pleasing and artifact free results.
更多查看译文
关键词
zero-shot dual learning,single image super-resolution,deep learning,remarkable reconstruction performance,downscaled images,real-world low-resolution images,blurry images,real-world super-resolution problem,dual-path architecture,image-specific low-to-high resolution mapping,generative adversarial network,DualSR architecture,visual quality,nonblind super-resolution methods,real-world LR images,cycle consistency losses
AI 理解论文
溯源树
样例
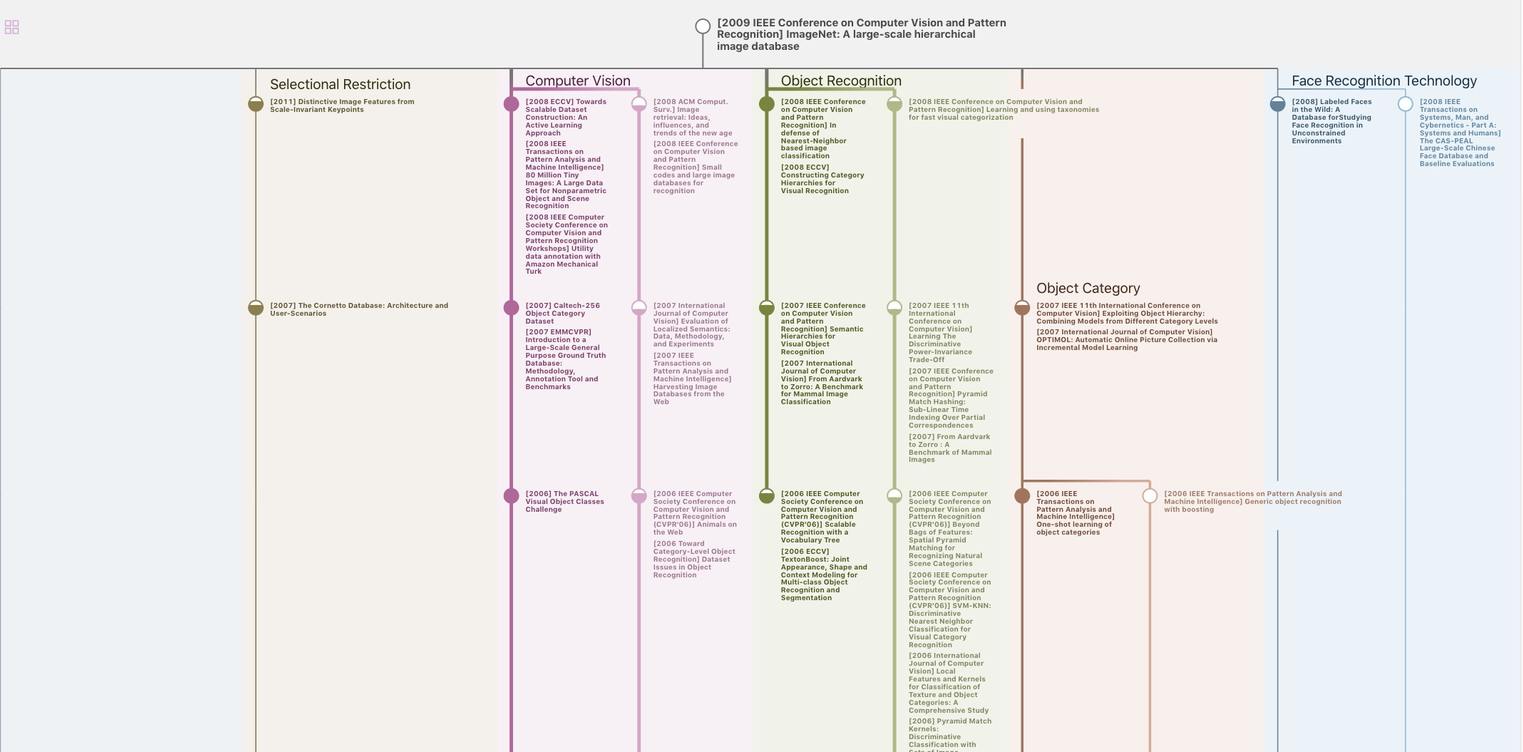
生成溯源树,研究论文发展脉络
Chat Paper
正在生成论文摘要