Unsupervised Meta-Domain Adaptation For Fashion Retrieval
2021 IEEE WINTER CONFERENCE ON APPLICATIONS OF COMPUTER VISION (WACV 2021)(2021)
摘要
Cross-domain fashion item retrieval naturally arises when unconstrained consumer images are used to query for fashion items in a collection of high-quality photographs provided by retailers. To perform this task, approaches typically leverage both consumer and shop domains from a given dataset to learn a domain invariant representation, allowing these images of different nature to be directly compared. When consumer images are not available beforehand, such training is impossible. In this paper, we focus on this challenging and yet practical scenario, and we propose instead to leverage representations learned for cross-domain retrieval from another source dataset and to adapt them to the target dataset for this particular setting. More precisely, we bypass the lack of consumer images and directly target the more challenging meta-domain gap which occurs between consumer images and shop images, independently of their dataset. Assuming that datasets share some similar fashion items, we cluster their shop images and leverage the clusters to automatically generate pseudo-labels. Those are used to associate consumer and shop images across datasets, which in turn allows to learn meta-domain-invariant representations suitable for cross-domain retrieval in the target dataset. The features and code are available at https:// github. com/vivoutlaw/UDMA.
更多查看译文
关键词
cross-domain retrieval,source dataset,target dataset,challenging meta-domain gap,shop images,similar fashion items,meta-domain-invariant representations,unsupervised meta-domain adaptation,fashion retrieval,cross-domain fashion item retrieval,unconstrained consumer images,high-quality photographs,leverage both consumer,domain invariant representation,challenging scenario,yet practical scenario,leverage representations
AI 理解论文
溯源树
样例
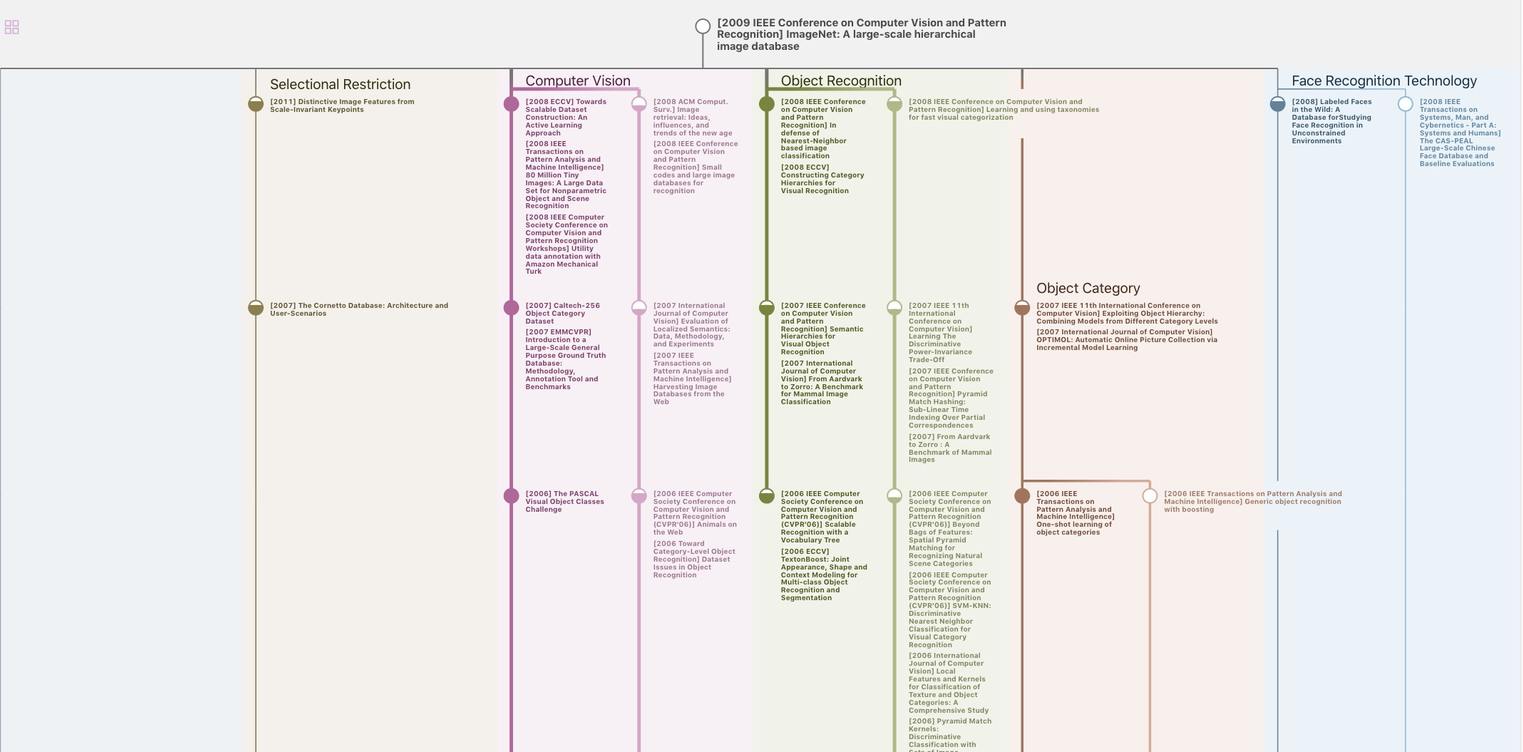
生成溯源树,研究论文发展脉络
Chat Paper
正在生成论文摘要