Bler-Based Adaptive Q-Learning For Efficient Random Access In Noma-Based Mmtc Networks
2021 IEEE 93RD VEHICULAR TECHNOLOGY CONFERENCE (VTC2021-SPRING)(2021)
摘要
The ever-increasing number of machine-type communications (MTC) devices and the limited available radio resources are leading to a crucial issue of radio access network (RAN) congestion in upcoming 5G and beyond wireless networks. Thus, it is crucial to investigate novel techniques to minimize RAN congestion in massive MTC (mMTC) networks while taking the underlying short-packet communications (SPC) into account. In this paper, we propose an adaptive Q-learning (AQL) algorithm based on block error rate (BLER), an important metric in SPC, for a non-orthogonal multiple access (NOMA) based mMTC system. The proposed method aims to efficiently accommodate MTC devices to the available random access (RA) slots in order to significantly reduce the possible collisions, and subsequently to enhance the system throughput. Furthermore, in order to obtain more practical insights on the system design, the scenario of imperfect successive interference cancellation (ISIC) is considered as compared to the widely-used perfect SIC assumption. The performance of the proposed AQL method is compared with the recent Q-learning solutions in the literature in terms of system throughput over a range of parameters such as the number of devices, blocklength, and residual interference caused by ISIC, along with its convergence evaluation. Our simulation results illustrate the superiority of the proposed method over the existing techniques, in the scenarios where the number of devices is higher than the number of available RA time-slots.
更多查看译文
关键词
BLER, MTC, NOMA, Q-Learning, short-packet communications
AI 理解论文
溯源树
样例
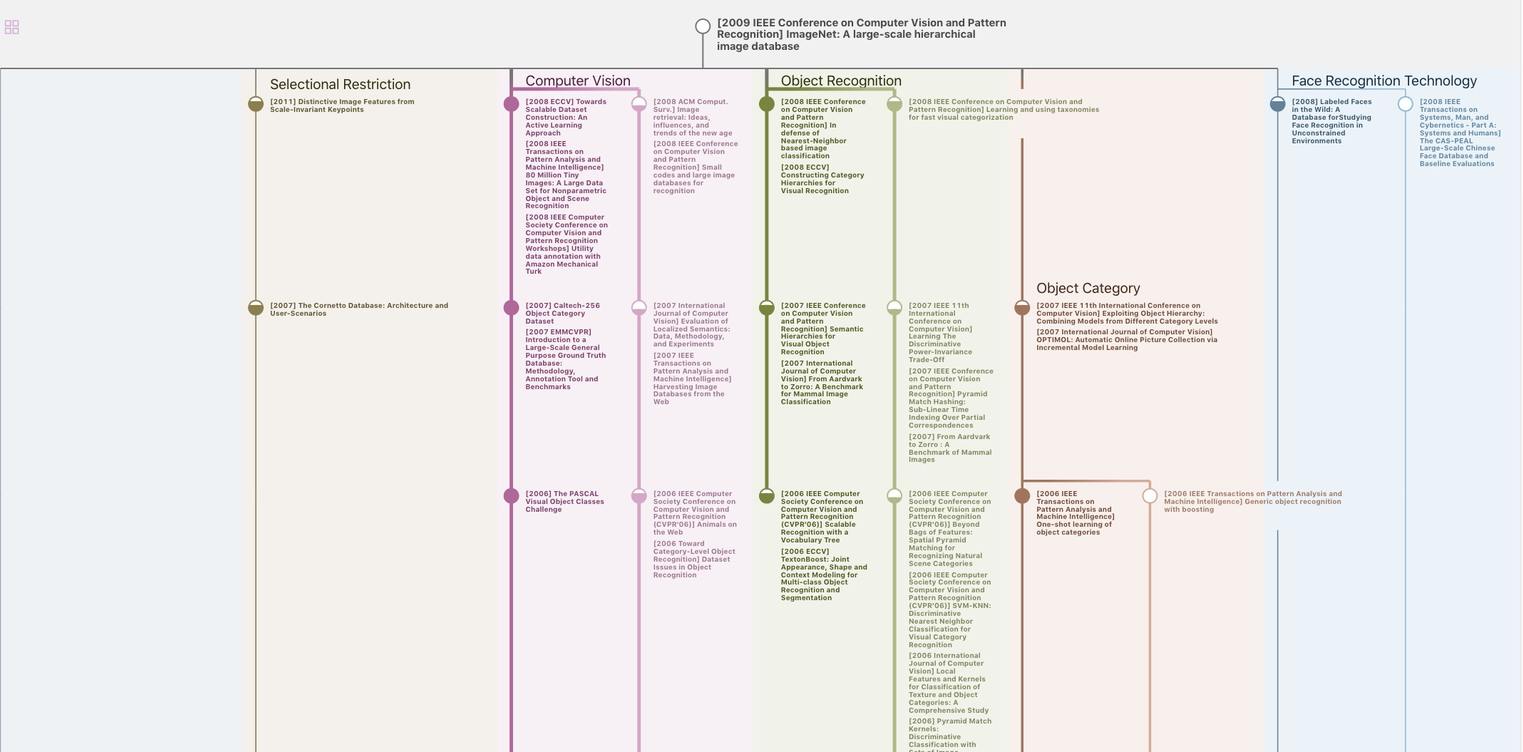
生成溯源树,研究论文发展脉络
Chat Paper
正在生成论文摘要