URLLC Resource Slicing and Scheduling in 5G Vehicular Edge Computing
2021 IEEE 93RD VEHICULAR TECHNOLOGY CONFERENCE (VTC2021-SPRING)(2021)
摘要
The 5th generation (5G) mobile network technology is accelerating the development of autonomous vehicles by significantly shortening the communication latency and improving the reliability of network connection and transmission. However, as the number of vehicles increases, neither cloud servers nor multi-access edge computing (MEC) servers alone could sufficiently meet the Quality-of-Service (QoS) requirements for computing-intensive vehicle tasks. In this paper, we consider a hierarchical offloading scenario, where vehicle tasks are allowed to execute in MEC servers, convergence servers or cloud servers. To reduce the cost of latency and energy, we optimize the communication and computation resource allocation problem. The optimization problem is converted to a Markov decision process, and deep reinforcement learning is used to tackle the resource slicing and scheduling problem. Simulation results show that the proposed scheme is more resilient and efficient than that of single cloud server offloading or single MEC server offloading.
更多查看译文
关键词
Vehicular edge computing, 5G, resource slicing, deep reinforcement learning
AI 理解论文
溯源树
样例
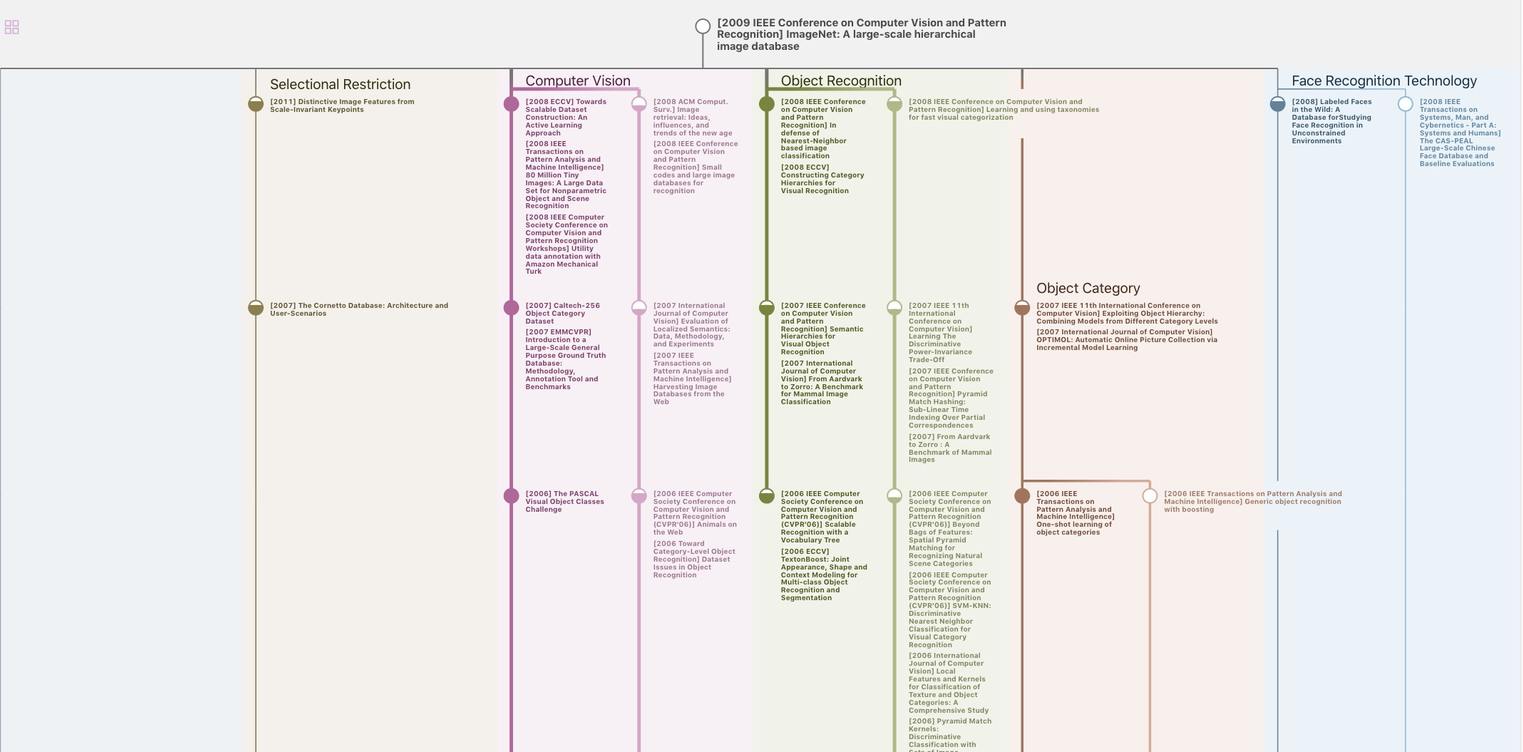
生成溯源树,研究论文发展脉络
Chat Paper
正在生成论文摘要