The Ensemble Kalman Filter for Rare Event Estimation
SIAM/ASA J. Uncertain. Quantification(2022)
摘要
We present a novel sampling-based method for estimating probabilities of rare or failure events. Our approach is founded on the Ensemble Kalman filter (EnKF) for inverse problems. Therefore, we reformulate the rare event problem as an inverse problem and apply the EnKF to generate failure samples. To estimate the probability of failure, we use the final EnKF samples to fit a distribution model and apply Importance Sampling with respect to the fitted distribution. This leads to an unbiased estimator if the density of the fitted distribution admits positive values within the whole failure domain. To handle multi-modal failure domains, we localise the covariance matrices in the EnKF update step around each particle and fit a mixture distribution model in the Importance Sampling step. For affine linear limit-state functions, we investigate the continuous-time limit and large time properties of the EnKF update. We prove that the mean of the particles converges to a convex combination of the most likely failure point and the mean of the optimal Importance Sampling density if the EnKF is applied without noise. We provide numerical experiments to compare the performance of the EnKF with Sequential Importance Sampling.
更多查看译文
关键词
ensemble kalman filter,estimation
AI 理解论文
溯源树
样例
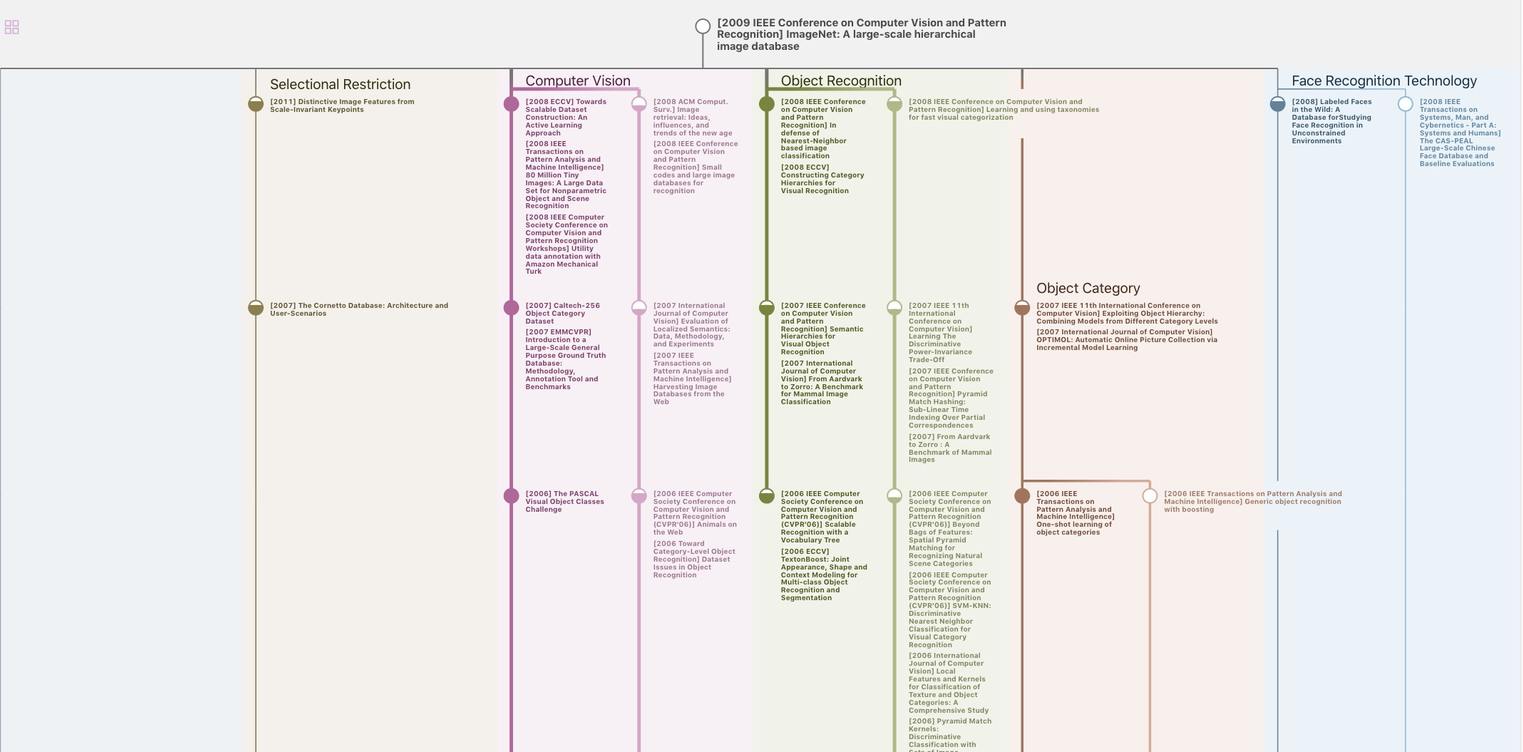
生成溯源树,研究论文发展脉络
Chat Paper
正在生成论文摘要