Characterizing Tissue Composition Through Combined Analysis of Morphologies and Transcriptional States
user-5d4bc4a8530c70a9b361c870(2021)
摘要
Advances in spatial transcriptomics technologies enable simultaneous profiling of morphological and transcriptional modalities from the same cells or regions within tissues. Here, we present multi-modal structured embedding (MUSE), an approach to deeply characterize tissue heterogeneity through analysis of combined image and transcriptional measurements. We demonstrate that MUSE can discover tissue subpopulations missed by either modality as well as compensate for modality-specific noise. We apply MUSE to diverse datasets containing spatial transcriptomics (seqFISH+, STARmap or ST) and imaging (H&E or fluorescence microscopy) modalities. MUSE identified biologically meaningful tissue subpopulations and stereotyped spatial patterning within heterogeneous mouse cortex, human cancer tissue and mouse brain sections with Alzheimer’s disease. MUSE provides a framework for combining multi-modal data to reveal deeper insights into the states, functions and organizations of cells in complex biological tissues.
更多查看译文
关键词
Computational biology,Modality (human–computer interaction),Transcriptome,Biology,Human cancer,Mouse cortex,Tissue composition,Tissue heterogeneity
AI 理解论文
溯源树
样例
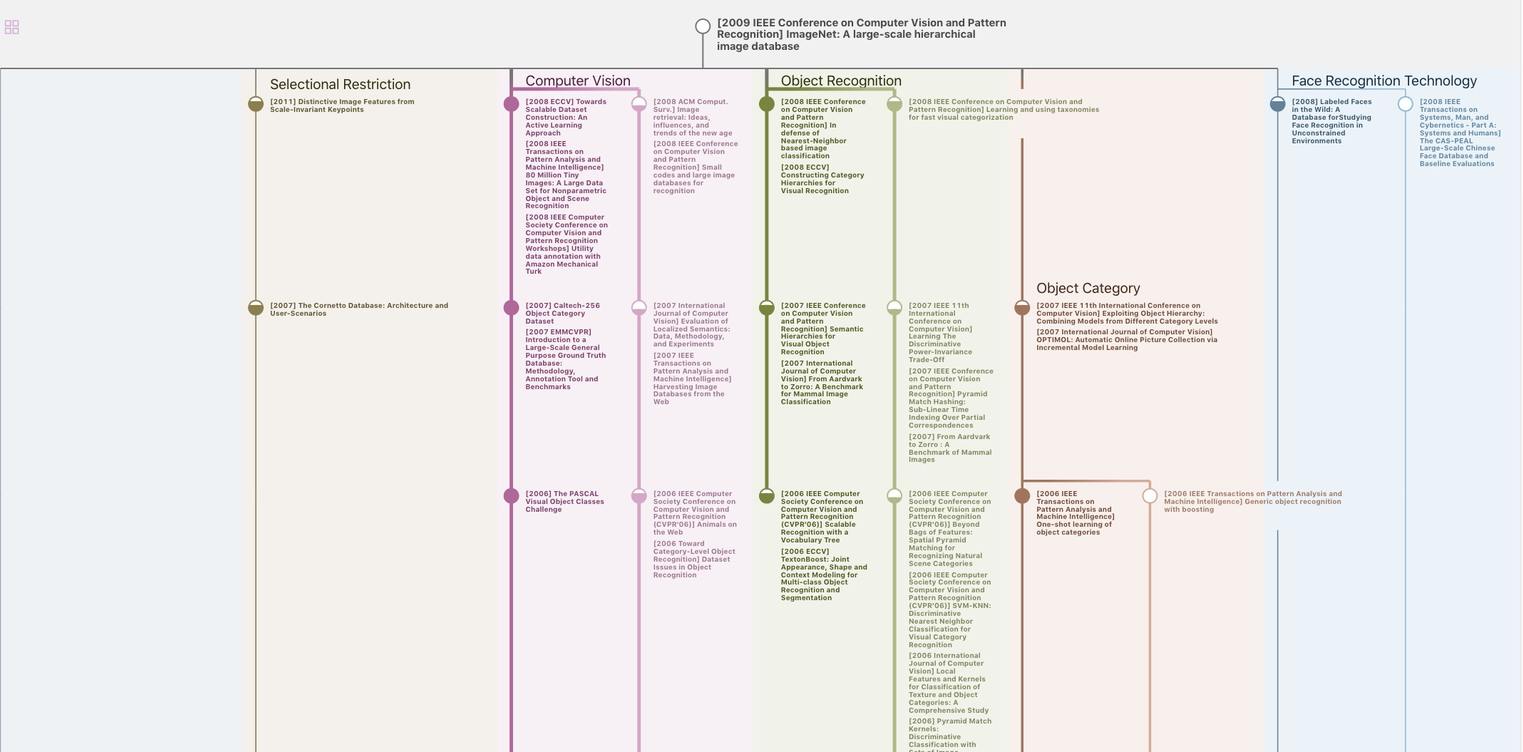
生成溯源树,研究论文发展脉络
Chat Paper
正在生成论文摘要